A Critical Analysis Of Reinforcement Learning's Role In AI Progress
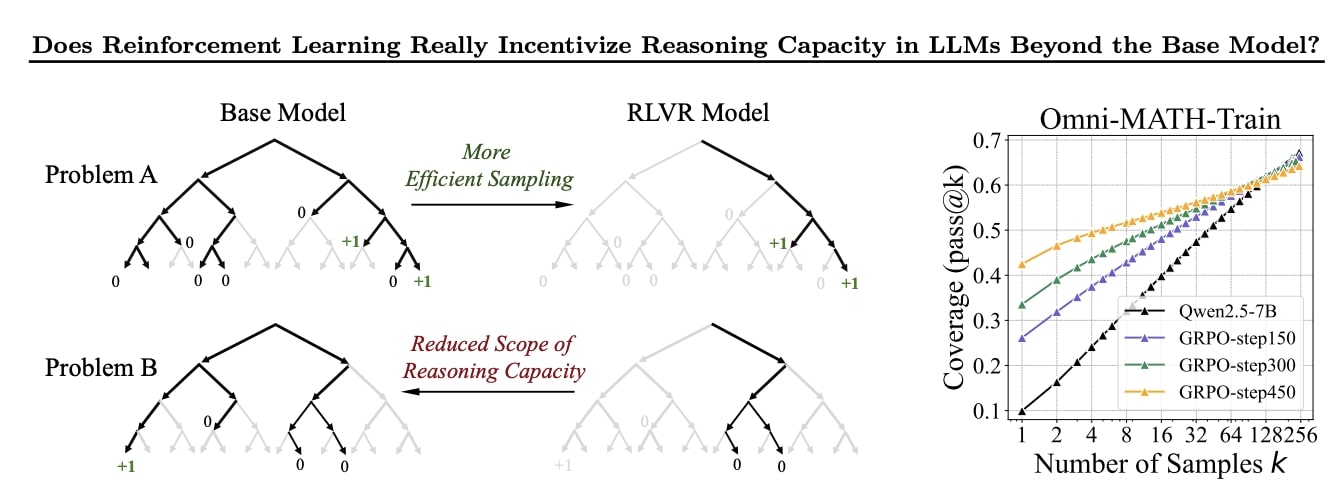
Welcome to your ultimate source for breaking news, trending updates, and in-depth stories from around the world. Whether it's politics, technology, entertainment, sports, or lifestyle, we bring you real-time updates that keep you informed and ahead of the curve.
Our team works tirelessly to ensure you never miss a moment. From the latest developments in global events to the most talked-about topics on social media, our news platform is designed to deliver accurate and timely information, all in one place.
Stay in the know and join thousands of readers who trust us for reliable, up-to-date content. Explore our expertly curated articles and dive deeper into the stories that matter to you. Visit NewsOneSMADCSTDO now and be part of the conversation. Don't miss out on the headlines that shape our world!
Table of Contents
A Critical Analysis of Reinforcement Learning's Role in AI Progress
Reinforcement learning (RL), a subfield of machine learning, has emerged as a powerful driver of artificial intelligence (AI) advancements. From mastering complex games like Go and chess to optimizing robotic control systems and revolutionizing personalized recommendations, RL's impact is undeniable. However, a critical analysis reveals both its significant contributions and inherent limitations, shaping the future trajectory of AI research.
H2: The Triumphs of Reinforcement Learning
RL's success stems from its ability to train agents to make optimal decisions in dynamic environments through trial and error. This paradigm shift, away from supervised learning's reliance on labeled data, has unlocked new possibilities:
- Mastering Complex Games: DeepMind's AlphaGo, AlphaZero, and MuZero showcased RL's potential by defeating world champions in Go, chess, and shogi, respectively. These breakthroughs demonstrated RL's capacity to learn intricate strategies and outperform human experts in highly complex domains.
- Robotics and Control Systems: RL algorithms are increasingly used to optimize robotic control, enabling robots to perform intricate tasks like walking, grasping objects, and navigating complex environments more efficiently and robustly than traditional methods.
- Personalized Recommendations: RL algorithms power personalized recommendations in various applications, from streaming services to e-commerce platforms. By learning user preferences and behavior, these systems deliver tailored content and product suggestions, enhancing user experience and driving engagement.
- Resource Optimization: From traffic flow management to energy grid optimization, RL algorithms are finding applications in solving real-world problems requiring efficient resource allocation and dynamic decision-making.
H2: Challenges and Limitations of Reinforcement Learning
Despite its remarkable achievements, RL faces several critical challenges that hinder its widespread adoption and limit its potential:
- Sample Inefficiency: RL algorithms often require vast amounts of data and computational resources to achieve satisfactory performance. This sample inefficiency makes training time-consuming and expensive, particularly for complex tasks.
- Reward Function Design: Defining a suitable reward function is crucial for guiding the agent's learning process. Poorly designed reward functions can lead to unintended and undesirable behaviors, a phenomenon known as reward hacking.
- Safety and Reliability: Deploying RL agents in real-world settings requires ensuring their safety and reliability. Unpredictable behavior or catastrophic failures can have significant consequences, especially in high-stakes applications like autonomous driving.
- Explainability and Interpretability: Understanding why an RL agent makes a particular decision is often challenging. This lack of transparency hinders trust and makes it difficult to debug or improve the agent's performance. The "black box" nature of many RL models remains a significant obstacle.
- Generalization and Transfer Learning: RL agents often struggle to generalize their learned behavior to new and unseen environments. Transfer learning, the ability to apply knowledge gained in one task to another, remains a significant area of ongoing research.
H2: The Future of Reinforcement Learning in AI
Despite these limitations, the future of RL in AI remains bright. Ongoing research focuses on addressing the challenges mentioned above through:
- Improved Algorithms: Developing more sample-efficient algorithms is crucial for reducing training time and computational costs.
- Safe RL: Research in safe RL focuses on designing algorithms that guarantee safe and reliable behavior, preventing unintended consequences.
- Explainable RL: Efforts to make RL models more transparent and interpretable are crucial for building trust and facilitating debugging.
- Transfer Learning and Generalization: Improving the ability of RL agents to generalize and transfer knowledge to new tasks will broaden their applicability.
In conclusion, reinforcement learning has undeniably revolutionized several areas of AI, but its limitations require careful consideration. Overcoming these challenges through ongoing research and development will be crucial in unlocking RL's full potential and shaping the future of artificial intelligence. The future of AI, in many ways, hinges on the continued progress and refinement of this powerful learning paradigm.
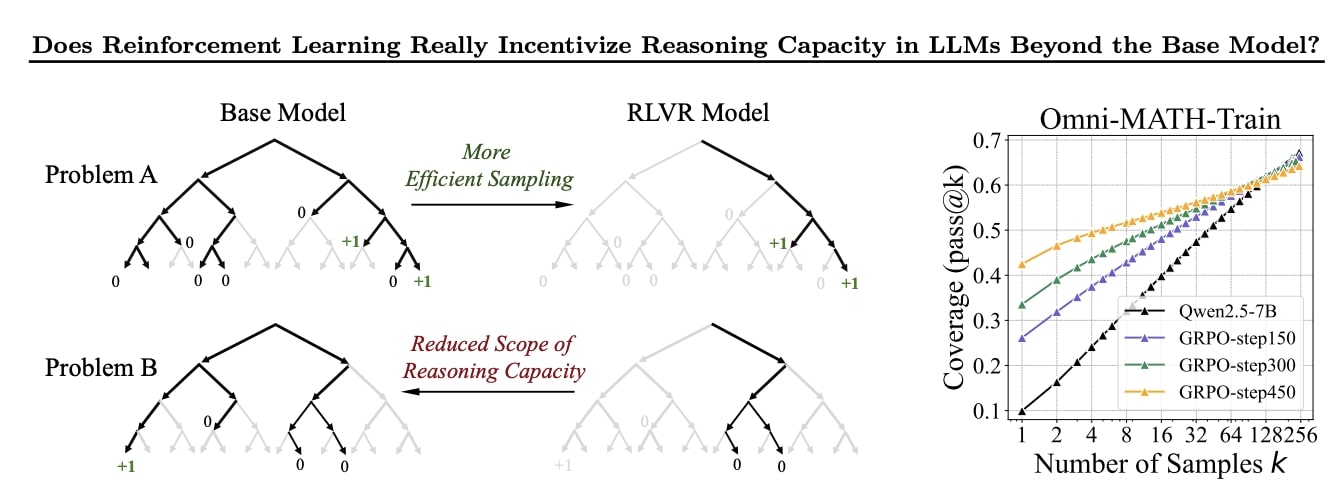
Thank you for visiting our website, your trusted source for the latest updates and in-depth coverage on A Critical Analysis Of Reinforcement Learning's Role In AI Progress. We're committed to keeping you informed with timely and accurate information to meet your curiosity and needs.
If you have any questions, suggestions, or feedback, we'd love to hear from you. Your insights are valuable to us and help us improve to serve you better. Feel free to reach out through our contact page.
Don't forget to bookmark our website and check back regularly for the latest headlines and trending topics. See you next time, and thank you for being part of our growing community!
Featured Posts
-
Elections 2024 L Ontario Terrain De Bataille Politique
Apr 28, 2025 -
Strike Ready Ai Platform Next Generation Security Triage For Proactive Threat Response
Apr 28, 2025 -
Trumps Very Special Player Status Secured Narrow Victory Over Murphy
Apr 28, 2025 -
Decoding Web3 Verification Separating Fact From Illusion
Apr 28, 2025 -
Major Fire At Wfcu Centre Impact On Events And The Windsor Community
Apr 28, 2025
Latest Posts
-
Paramount Streaming Guide Everything Coming In May 2025
Apr 30, 2025 -
Strong Winds Fan Wildfire Southeast Of Tucson Leading To Mandatory Evacuations
Apr 30, 2025 -
Iga Swiateks Narrow Escape Shes Through To The Madrid Open Quarterfinals
Apr 30, 2025 -
Leeds U21s And Sutton United Face Off National League Cup Final Preview
Apr 30, 2025 -
Huawei Unveils Fastest Ai Chip As Us Restricts Nvidia H20 Exports To China
Apr 30, 2025