A Critical Look At Reinforcement Learning's Impact On AI Model Performance
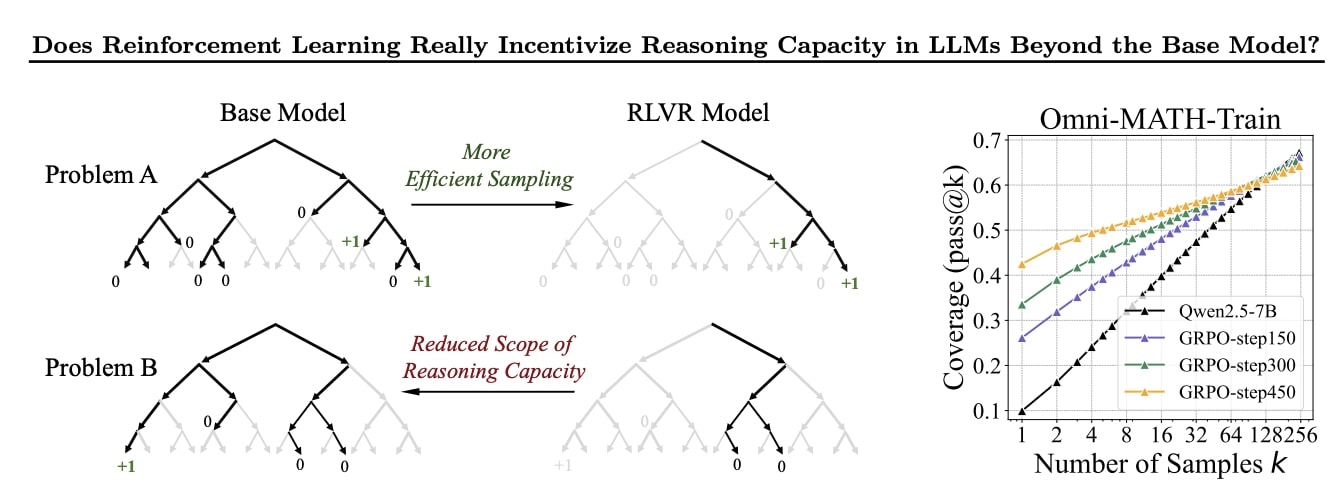
Welcome to your ultimate source for breaking news, trending updates, and in-depth stories from around the world. Whether it's politics, technology, entertainment, sports, or lifestyle, we bring you real-time updates that keep you informed and ahead of the curve.
Our team works tirelessly to ensure you never miss a moment. From the latest developments in global events to the most talked-about topics on social media, our news platform is designed to deliver accurate and timely information, all in one place.
Stay in the know and join thousands of readers who trust us for reliable, up-to-date content. Explore our expertly curated articles and dive deeper into the stories that matter to you. Visit NewsOneSMADCSTDO now and be part of the conversation. Don't miss out on the headlines that shape our world!
Table of Contents
A Critical Look at Reinforcement Learning's Impact on AI Model Performance
Reinforcement learning (RL), a powerful machine learning technique, has revolutionized AI, enabling agents to learn optimal strategies through trial and error. However, despite its successes in domains like game playing and robotics, a critical examination of RL's impact on overall AI model performance reveals both significant advantages and inherent limitations. This article delves into the complexities of RL, exploring its strengths and weaknesses to provide a balanced perspective on its role in shaping the future of AI.
The Strengths of Reinforcement Learning
RL's ability to learn complex behaviors without explicit programming is its primary strength. Unlike supervised learning, which relies on large labeled datasets, RL agents learn through interaction with an environment, receiving rewards for desirable actions and penalties for undesirable ones. This allows for the development of sophisticated AI models capable of tackling problems that are difficult, if not impossible, to solve using traditional methods.
- Adaptability: RL agents can adapt to changing environments and unexpected situations, making them robust and resilient. This adaptability is crucial in real-world applications where conditions are rarely static.
- Exploration and Exploitation: The inherent balance between exploration (trying new actions) and exploitation (using known good actions) allows RL agents to discover optimal strategies efficiently. This is particularly valuable in complex environments with many possible actions.
- Optimal Policy Learning: RL aims to find the optimal policy, the best strategy for achieving a goal. This focus on optimization leads to high-performing AI models in various domains.
The Challenges and Limitations of Reinforcement Learning
Despite its power, RL faces several significant challenges that limit its widespread applicability and impact on overall AI model performance:
- Sample Inefficiency: RL algorithms often require a vast number of interactions with the environment to learn effectively. This can be computationally expensive and time-consuming, especially in complex environments. This sample inefficiency is a major bottleneck.
- Reward Design: Defining appropriate reward functions is crucial for RL's success. Poorly designed reward functions can lead to unexpected and undesirable behavior, a phenomenon known as reward hacking. Crafting effective reward functions remains a significant research challenge.
- Generalization: RL agents often struggle to generalize their learned strategies to new, unseen environments. This limits their applicability to real-world scenarios where conditions constantly change.
- Interpretability and Explainability: Understanding why an RL agent chooses a particular action can be difficult. The lack of interpretability and explainability hinders trust and adoption in critical applications.
The Future of Reinforcement Learning and AI Model Performance
Despite these challenges, research continues to address the limitations of RL. New techniques such as transfer learning, imitation learning, and hierarchical RL are being developed to improve sample efficiency, generalization, and interpretability. The integration of RL with other AI techniques holds immense potential for creating more robust and powerful AI systems.
Key Takeaways:
- Reinforcement learning offers significant advantages in developing adaptive and high-performing AI models.
- However, challenges like sample inefficiency, reward design, generalization, and interpretability remain significant hurdles.
- Ongoing research aims to overcome these limitations, paving the way for even more impactful applications of RL in the future.
The future of AI hinges on addressing the complexities of reinforcement learning. By understanding both its strengths and weaknesses, we can harness its power responsibly and ethically, leading to significant advancements in artificial intelligence and its impact on various aspects of our lives. The ongoing development and refinement of RL techniques will undoubtedly continue to shape the landscape of AI model performance for years to come.
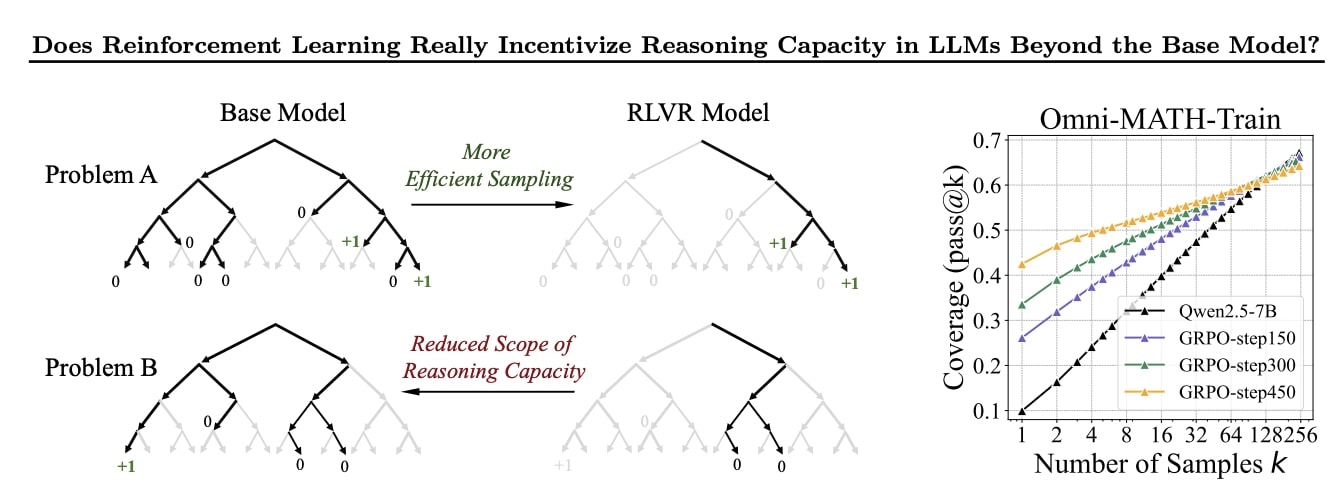
Thank you for visiting our website, your trusted source for the latest updates and in-depth coverage on A Critical Look At Reinforcement Learning's Impact On AI Model Performance. We're committed to keeping you informed with timely and accurate information to meet your curiosity and needs.
If you have any questions, suggestions, or feedback, we'd love to hear from you. Your insights are valuable to us and help us improve to serve you better. Feel free to reach out through our contact page.
Don't forget to bookmark our website and check back regularly for the latest headlines and trending topics. See you next time, and thank you for being part of our growing community!
Featured Posts
-
Arsenal Vs Psg 0 1 Victory For Parisians Dembele The Hero
May 01, 2025 -
Competition And Collaboration How Mapmakers Conflicts Advanced Martian Exploration
May 01, 2025 -
Analyzing The Dire Warning They Will Unleash Hell On Us
May 01, 2025 -
Arsenal In Avrupa Rueyasi Son Buldu Psg Finalde
May 01, 2025 -
Exploring The New Chat Gpt Search Features A Comprehensive Guide
May 01, 2025
Latest Posts
-
Uefa Rule Changes Force Barcelonas Flick To Make Champions League Decision
May 01, 2025 -
Massive Power Failure In Spain And Portugal Restoration And Investigation Efforts
May 01, 2025 -
Confirmado El Once De Hansi Flick Para El Barca Inter
May 01, 2025 -
Ai And Web3 Understanding The Risks Of Granting Key Access To Ai Models
May 01, 2025 -
Analysis Warren Buffett Sells Apple Stock Cutting Stake By 13
May 01, 2025