A Realistic Assessment Of Reinforcement Learning's Impact On AI Models
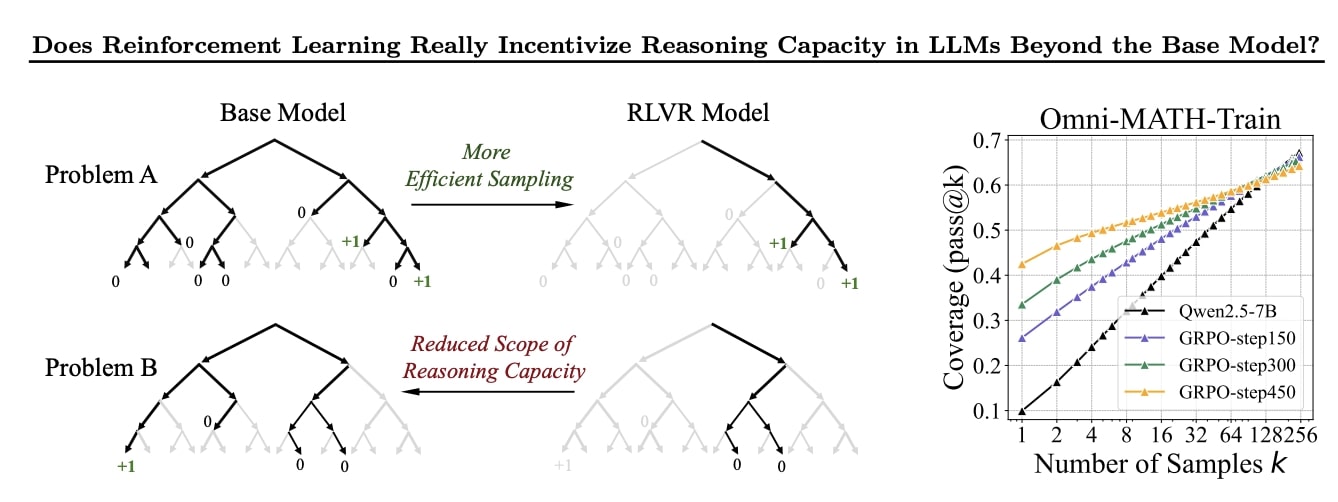
Welcome to your ultimate source for breaking news, trending updates, and in-depth stories from around the world. Whether it's politics, technology, entertainment, sports, or lifestyle, we bring you real-time updates that keep you informed and ahead of the curve.
Our team works tirelessly to ensure you never miss a moment. From the latest developments in global events to the most talked-about topics on social media, our news platform is designed to deliver accurate and timely information, all in one place.
Stay in the know and join thousands of readers who trust us for reliable, up-to-date content. Explore our expertly curated articles and dive deeper into the stories that matter to you. Visit NewsOneSMADCSTDO now and be part of the conversation. Don't miss out on the headlines that shape our world!
Table of Contents
A Realistic Assessment of Reinforcement Learning's Impact on AI Models
Reinforcement learning (RL), a subfield of machine learning, is generating significant buzz. Its potential to create highly adaptable and intelligent AI systems is undeniable, but hype often obscures a realistic appraisal of its current capabilities and limitations. This article delves into a balanced assessment of RL's impact, exploring its successes, challenges, and future prospects.
The Rise of Reinforcement Learning in AI
Reinforcement learning stands out from other machine learning approaches because it focuses on training AI agents to make decisions within an environment. Through trial and error, the agent learns to optimize its actions to maximize cumulative rewards. This paradigm has proven remarkably effective in several domains, leading to breakthroughs in:
- Game Playing: RL's triumph in defeating human champions at complex games like Go and StarCraft II is a testament to its power. These achievements highlight RL's ability to master intricate strategies and adapt to unforeseen circumstances. AlphaGo, developed by DeepMind, is a prime example of this success.
- Robotics: RL algorithms are increasingly used to train robots to perform complex tasks, from grasping objects to navigating challenging terrains. The ability of RL to learn directly from experience makes it particularly well-suited for tasks where explicit programming is difficult or impossible.
- Resource Management: RL is showing promise in optimizing resource allocation in areas such as energy grids, traffic control, and supply chain management. By learning optimal strategies, RL can improve efficiency and reduce waste.
Challenges and Limitations of Current RL Models
Despite its impressive achievements, RL faces significant hurdles:
- Data Efficiency: RL algorithms often require vast amounts of data and training time to achieve satisfactory performance. This can be a major bottleneck, especially in real-world applications where data collection is expensive or time-consuming. Addressing sample inefficiency is a critical area of ongoing research.
- Reward Function Design: The effectiveness of RL hinges on carefully designing the reward function, which defines the desired behavior of the agent. Poorly designed reward functions can lead to unintended consequences and suboptimal performance. This is a significant challenge, requiring domain expertise and careful consideration.
- Safety and Robustness: Ensuring the safety and robustness of RL agents is crucial, particularly in applications where mistakes can have serious consequences. Developing techniques to mitigate risks and guarantee reliable behavior remains an active area of research.
- Explainability and Interpretability: Understanding why an RL agent makes a particular decision is often difficult. The lack of transparency can hinder the adoption of RL in high-stakes applications where accountability is paramount. Developing more interpretable RL models is crucial for building trust.
The Future of Reinforcement Learning
Despite these challenges, the future of RL looks bright. Ongoing research is focused on addressing the limitations mentioned above, with promising developments in:
- Transfer Learning: Enabling RL agents to transfer knowledge learned in one environment to another can significantly improve data efficiency.
- Hierarchical Reinforcement Learning: Breaking down complex tasks into smaller subtasks can simplify the learning process and improve performance.
- Safe Reinforcement Learning: Developing methods to ensure the safety and robustness of RL agents is a priority area.
Conclusion:
Reinforcement learning represents a powerful paradigm for building intelligent AI systems. While current RL models face challenges in terms of data efficiency, reward function design, safety, and interpretability, ongoing research is addressing these limitations. The future holds immense potential for RL to transform various industries and solve complex real-world problems. However, a balanced perspective, acknowledging both the successes and limitations, is crucial for responsible development and deployment.
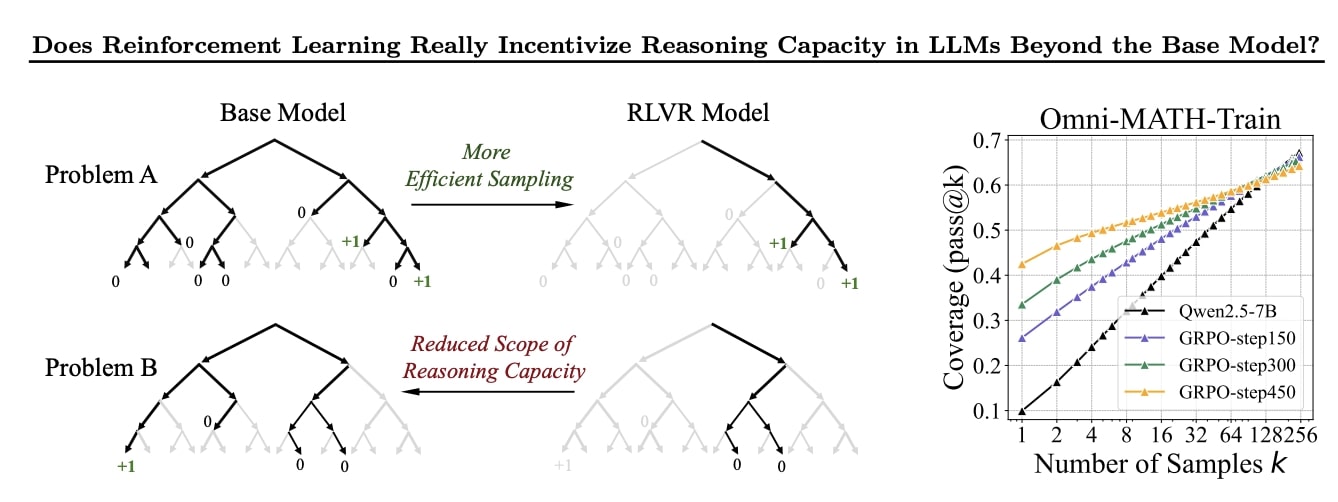
Thank you for visiting our website, your trusted source for the latest updates and in-depth coverage on A Realistic Assessment Of Reinforcement Learning's Impact On AI Models. We're committed to keeping you informed with timely and accurate information to meet your curiosity and needs.
If you have any questions, suggestions, or feedback, we'd love to hear from you. Your insights are valuable to us and help us improve to serve you better. Feel free to reach out through our contact page.
Don't forget to bookmark our website and check back regularly for the latest headlines and trending topics. See you next time, and thank you for being part of our growing community!
Featured Posts
-
Premier League Live Nottingham Forest Vs Brentford Score Team News And Highlights
May 02, 2025 -
Twins Vs Guardians April 28th 2025 Game Summary And Highlights 11 1
May 02, 2025 -
Gauffs Dominant Victory Over Swiatek In Madrid
May 02, 2025 -
The Contentious Creation Of Mars Maps Shaping Perceptions Of The Red Planet
May 02, 2025 -
Election 2024 Labor And Coalition Deliver Final Campaign Pitches
May 02, 2025