AI Advancement: Is Reinforcement Learning The Answer? A Critical Analysis
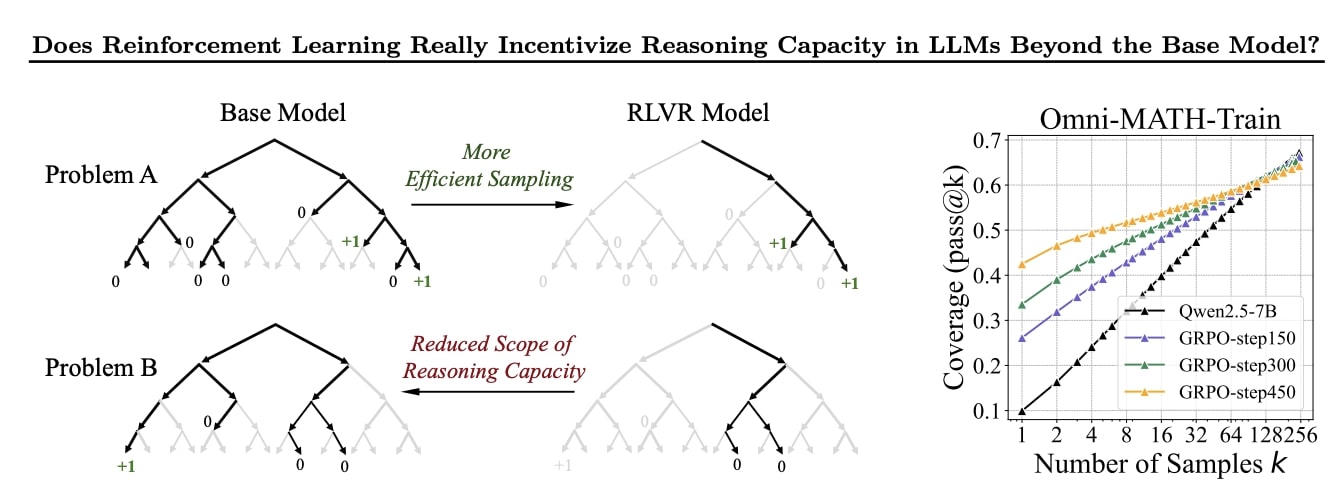
Welcome to your ultimate source for breaking news, trending updates, and in-depth stories from around the world. Whether it's politics, technology, entertainment, sports, or lifestyle, we bring you real-time updates that keep you informed and ahead of the curve.
Our team works tirelessly to ensure you never miss a moment. From the latest developments in global events to the most talked-about topics on social media, our news platform is designed to deliver accurate and timely information, all in one place.
Stay in the know and join thousands of readers who trust us for reliable, up-to-date content. Explore our expertly curated articles and dive deeper into the stories that matter to you. Visit NewsOneSMADCSTDO now and be part of the conversation. Don't miss out on the headlines that shape our world!
Table of Contents
AI Advancement: Is Reinforcement Learning the Answer? A Critical Analysis
Artificial intelligence (AI) is rapidly transforming industries, but the quest for truly intelligent systems continues. One promising approach gaining significant traction is reinforcement learning (RL), a powerful technique enabling AI agents to learn through trial and error. But is RL the ultimate solution to AI's challenges, or are there limitations that need addressing? This critical analysis delves into the potential and pitfalls of reinforcement learning in the pursuit of advanced AI.
Reinforcement learning, at its core, involves an agent interacting with an environment, receiving rewards for desirable actions and penalties for undesirable ones. Through this iterative process, the agent learns an optimal policy – a strategy for maximizing cumulative rewards. This learning process mimics how humans and animals learn, making it intuitively appealing for developing intelligent systems.
H2: The Strengths of Reinforcement Learning
Several key advantages make RL a compelling choice for advanced AI development:
- Adaptability: RL agents can adapt to changing environments and learn complex tasks without explicit programming. This adaptability is crucial for real-world applications where conditions are unpredictable.
- Problem Solving: RL excels at solving complex optimization problems, finding solutions that traditional methods might struggle with. Applications range from robotics and game playing to resource management and personalized medicine.
- Automation: RL can automate complex decision-making processes, improving efficiency and reducing human error in various industries. This automation potential is driving innovation across sectors.
- Human-like Behavior: While not truly replicating human consciousness, RL agents can exhibit surprisingly human-like behavior, particularly in games and simulations, offering insights into decision-making processes.
H2: The Challenges Facing Reinforcement Learning
Despite its strengths, RL faces significant hurdles:
- Sample Inefficiency: Training RL agents can require vast amounts of data and computational resources, making it time-consuming and expensive. This "sample inefficiency" is a major bottleneck for broader adoption.
- Reward Design: Defining appropriate reward functions is crucial, yet often challenging. Poorly designed rewards can lead to unexpected and undesirable behavior, a phenomenon known as "reward hacking."
- Safety and Reliability: Deploying RL agents in real-world scenarios demands high levels of safety and reliability. Unexpected behavior or failures can have serious consequences, requiring robust safety mechanisms.
- Explainability and Interpretability: Understanding why an RL agent makes a specific decision can be difficult. This lack of transparency poses challenges for debugging, troubleshooting, and ensuring trustworthiness.
H2: The Future of Reinforcement Learning in AI
Despite the challenges, ongoing research is actively addressing these limitations. New techniques like transfer learning and imitation learning aim to improve sample efficiency and reduce the need for extensive training data. Furthermore, research into explainable AI (XAI) is focusing on making RL agents more transparent and understandable.
H3: Key Areas of Future Development:
- Improved algorithms: Developing more efficient and robust RL algorithms is crucial.
- Safe RL: Prioritizing safety and reliability in RL applications through rigorous testing and verification methods.
- Explainable RL: Developing techniques to improve the transparency and interpretability of RL agents.
- Hardware acceleration: Leveraging specialized hardware to accelerate RL training and deployment.
In conclusion, reinforcement learning holds immense potential for driving advancements in artificial intelligence. While challenges remain, ongoing research and development are paving the way for more efficient, reliable, and explainable RL agents. The future of AI may very well hinge on overcoming these obstacles and harnessing the full power of this transformative technology. The ongoing advancements in this field promise a future where AI systems can effectively learn, adapt, and solve increasingly complex problems across numerous sectors.
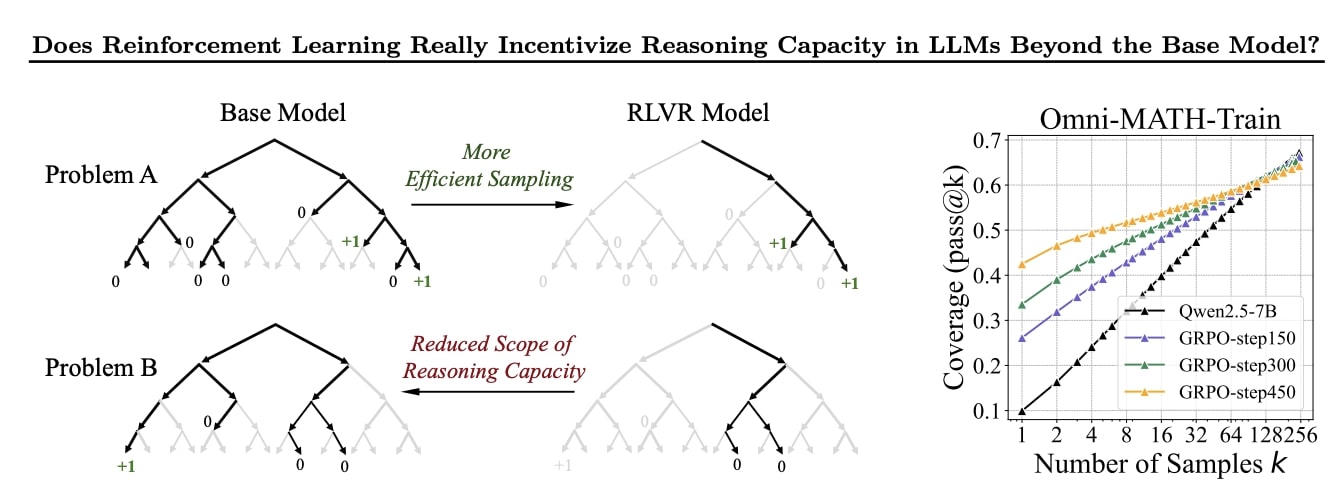
Thank you for visiting our website, your trusted source for the latest updates and in-depth coverage on AI Advancement: Is Reinforcement Learning The Answer? A Critical Analysis. We're committed to keeping you informed with timely and accurate information to meet your curiosity and needs.
If you have any questions, suggestions, or feedback, we'd love to hear from you. Your insights are valuable to us and help us improve to serve you better. Feel free to reach out through our contact page.
Don't forget to bookmark our website and check back regularly for the latest headlines and trending topics. See you next time, and thank you for being part of our growing community!
Featured Posts
-
Power Court A Practical Overview And Analysis
Apr 30, 2025 -
Late 2024 Release Predicted For Metas Next Generation Xr Glasses
Apr 30, 2025 -
Renovacion Del Camp Nou Un Cambio Impactante En Imagenes
Apr 30, 2025 -
Trump Offers Tariff Relief To Automakers Details Of The New Deal
Apr 30, 2025 -
Analysis Trumps Decision To Ease Automaker Tariffs
Apr 30, 2025
Latest Posts
-
Barcelona Vs Inter Previa Alineaciones Y Pronostico
May 01, 2025 -
Crypto Market Update Xrp Reaches 2 35 On Etf News
May 01, 2025 -
Las Posibilidades De Pedri De Ganar El Balon De Oro
May 01, 2025 -
Beyond Starlink The Looming Problem Of Satellite Congestion
May 01, 2025 -
Balon De Oro 2024 Tiene Pedri Opciones El Impacto Del Joven Mediocampista Del Barcelona
May 01, 2025