AI Model Access In Web3: A Security Risk Assessment
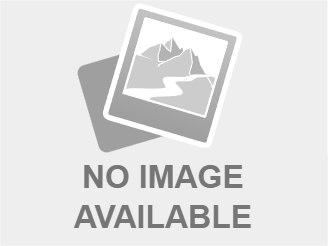
Welcome to your ultimate source for breaking news, trending updates, and in-depth stories from around the world. Whether it's politics, technology, entertainment, sports, or lifestyle, we bring you real-time updates that keep you informed and ahead of the curve.
Our team works tirelessly to ensure you never miss a moment. From the latest developments in global events to the most talked-about topics on social media, our news platform is designed to deliver accurate and timely information, all in one place.
Stay in the know and join thousands of readers who trust us for reliable, up-to-date content. Explore our expertly curated articles and dive deeper into the stories that matter to you. Visit NewsOneSMADCSTDO now and be part of the conversation. Don't miss out on the headlines that shape our world!
Table of Contents
AI Model Access in Web3: A Security Risk Assessment
The decentralized nature of Web3, while offering unprecedented opportunities, presents unique security challenges. The integration of AI models into this ecosystem significantly amplifies these risks, creating a landscape ripe for exploitation. This article explores the emerging security concerns surrounding AI model access within Web3 and offers insights into mitigating these vulnerabilities.
The Allure and the Peril of AI in Web3
AI models offer significant potential for Web3 applications, enhancing functionalities ranging from decentralized finance (DeFi) strategies and automated market making (AMM) to improved NFT creation and sophisticated on-chain analytics. However, the open and permissionless nature of many Web3 platforms makes them attractive targets for malicious actors seeking to exploit vulnerabilities in AI models.
Key Security Risks:
-
Data Poisoning: AI models are trained on data; corrupting this data, a process known as "data poisoning," can lead to flawed model outputs. In Web3, this could manifest as manipulating DeFi protocols' price prediction models, leading to skewed market valuations and financial losses for users. Malicious actors could manipulate the training data used by an AI model that determines lending rates in a DeFi application, allowing them to obtain favorable lending terms or trigger liquidation events for other users.
-
Model Extraction: Sophisticated attackers might attempt to extract the underlying model itself, potentially replicating it for malicious purposes or reverse-engineering its logic to find exploitable weaknesses. This is particularly dangerous for proprietary AI models powering critical Web3 infrastructure.
-
Inference Attacks: By feeding carefully chosen inputs to an AI model, attackers can infer sensitive information about the model's training data or its internal workings. This could reveal private keys, transaction patterns, or other confidential data used in DeFi protocols or NFT marketplaces.
-
Denial-of-Service (DoS) Attacks: Overloading an AI model with requests can render it unusable, disrupting services and causing significant operational disruptions. This is especially impactful for critical components of Web3 applications like decentralized exchanges (DEXs) that rely heavily on AI for order matching and liquidity management.
Mitigating the Risks:
Addressing these security concerns requires a multi-pronged approach:
-
Robust Data Validation: Implementing rigorous data validation procedures is crucial to prevent data poisoning attacks. This includes employing blockchain-based provenance tracking to ensure data integrity and authenticity.
-
Model Obfuscation and Protection: Techniques like model obfuscation and differential privacy can make it harder for attackers to extract or reverse-engineer the model. Secure enclaves and hardware-based security solutions also play a critical role.
-
Input Sanitization and Validation: Thoroughly sanitizing and validating all inputs to an AI model can significantly reduce the risk of inference attacks and prevent malicious code injection.
-
Rate Limiting and Distributed Systems: Implementing rate limiting and deploying AI models across a distributed network can help mitigate DoS attacks by making it harder to overload a single instance.
-
Regular Auditing and Penetration Testing: Regular security audits and penetration testing are essential to identify vulnerabilities early on and ensure the ongoing security of AI models within Web3 applications.
Conclusion:
The integration of AI models into Web3 presents a double-edged sword. While offering incredible potential, it also introduces significant security risks. Proactive measures, including robust data validation, model protection, input sanitization, and regular security audits, are crucial to mitigating these risks and ensuring the secure and sustainable growth of the Web3 ecosystem. Ignoring these challenges risks undermining the trust and stability vital for widespread adoption of this transformative technology.
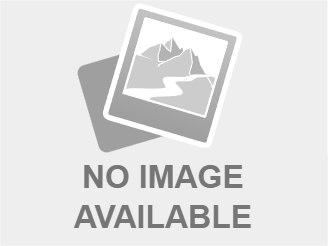
Thank you for visiting our website, your trusted source for the latest updates and in-depth coverage on AI Model Access In Web3: A Security Risk Assessment. We're committed to keeping you informed with timely and accurate information to meet your curiosity and needs.
If you have any questions, suggestions, or feedback, we'd love to hear from you. Your insights are valuable to us and help us improve to serve you better. Feel free to reach out through our contact page.
Don't forget to bookmark our website and check back regularly for the latest headlines and trending topics. See you next time, and thank you for being part of our growing community!
Featured Posts
-
Watch Quang Le Subdues Gaston Bolanos With Rear Naked Choke At Ufc On Espn 67
May 04, 2025 -
Controversy Erupts Police Hire Minority Candidate Despite Interview Failure
May 04, 2025 -
Ufc Fight Night May 3 In Depth Betting Odds And Predictions
May 04, 2025 -
Zelle Outage Latest News And Reports On Service Disruption
May 04, 2025 -
New Payment Features Arrive In Spotifys Updated I Phone App
May 04, 2025