AI Value Crisis: Global Finance Grapples With Costly Implementations
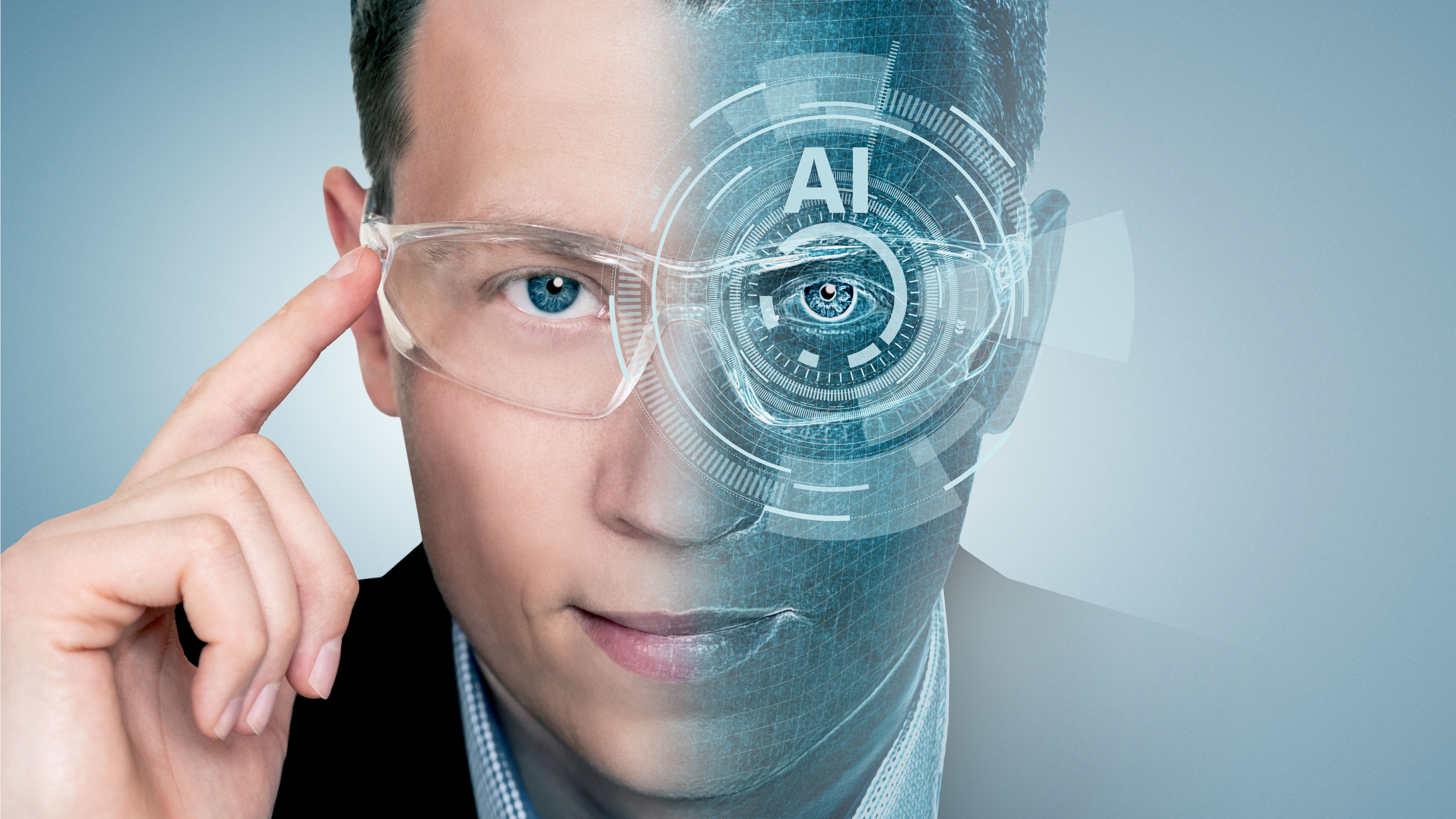
Welcome to your ultimate source for breaking news, trending updates, and in-depth stories from around the world. Whether it's politics, technology, entertainment, sports, or lifestyle, we bring you real-time updates that keep you informed and ahead of the curve.
Our team works tirelessly to ensure you never miss a moment. From the latest developments in global events to the most talked-about topics on social media, our news platform is designed to deliver accurate and timely information, all in one place.
Stay in the know and join thousands of readers who trust us for reliable, up-to-date content. Explore our expertly curated articles and dive deeper into the stories that matter to you. Visit NewsOneSMADCSTDO now and be part of the conversation. Don't miss out on the headlines that shape our world!
Table of Contents
AI Value Crisis: Global Finance Grapples with Costly Implementations
The promise of artificial intelligence (AI) transforming the global finance sector has hit a snag. While AI offers potential for increased efficiency and profitability, a growing number of financial institutions are facing a stark reality: the hefty costs of implementation often outweigh the immediate returns. This "AI value crisis" is forcing a critical reevaluation of AI strategies across the industry.
The High Cost of AI Adoption in Finance:
The initial allure of AI solutions – automated trading, fraud detection, risk management, and personalized customer service – has led many financial firms to invest heavily in AI infrastructure and talent. However, the reality is far more complex. Implementing AI requires significant upfront investment in:
- Specialized Hardware: High-performance computing infrastructure, including powerful GPUs and specialized servers, is crucial for training and running sophisticated AI models. This represents a substantial capital expenditure.
- Data Acquisition and Preparation: AI algorithms thrive on data. Financial institutions need to invest heavily in collecting, cleaning, and preparing vast datasets, a process often more complex and time-consuming than anticipated.
- Skilled Personnel: Finding and retaining data scientists, AI engineers, and other specialists with the expertise to develop, deploy, and maintain AI systems is a major challenge, driving up salaries and recruitment costs.
- Integration Challenges: Integrating AI solutions into existing legacy systems can be a complex and costly undertaking, often requiring significant modifications and potentially causing disruptions to existing workflows.
- Ongoing Maintenance and Updates: AI models require continuous monitoring, retraining, and updates to maintain accuracy and effectiveness, adding to the ongoing operational expenses.
Falling Short of Expectations:
Many financial institutions are finding that the projected ROI on their AI investments isn't materializing as quickly, or at all, as initially hoped. This is due to several factors:
- Unrealistic Expectations: Some organizations overestimated the immediate impact of AI, failing to account for the complexities of implementation and the time required to see tangible results.
- Data Quality Issues: Poor data quality can severely limit the accuracy and effectiveness of AI models, leading to inaccurate predictions and poor decision-making.
- Lack of Clear Strategy: Implementing AI without a clear, well-defined strategy, including specific goals and measurable KPIs, can lead to wasted resources and disappointing outcomes.
- Talent Shortages: The scarcity of skilled AI professionals is hindering the progress of many projects, creating bottlenecks and delays.
Navigating the AI Value Crisis:
To overcome this crisis, financial institutions need to adopt a more pragmatic and strategic approach to AI adoption:
- Focus on Specific Use Cases: Prioritize AI initiatives that offer the most immediate and measurable value, rather than attempting to implement AI across the board.
- Invest in Data Quality: Ensure data accuracy, completeness, and consistency are paramount to maximize the effectiveness of AI models.
- Build Internal Expertise: Invest in training and development programs to build internal AI expertise, reducing reliance on expensive external consultants.
- Adopt a Phased Approach: Implement AI in stages, starting with smaller, less complex projects to gain experience and build confidence before tackling larger initiatives.
- Partner with Specialized Vendors: Collaborate with experienced AI vendors to leverage their expertise and reduce implementation complexities.
The AI value crisis in global finance is not a sign of AI's failure, but rather a crucial learning experience. By adopting a more strategic, data-driven, and pragmatic approach, financial institutions can harness the transformative power of AI while mitigating the risks associated with costly implementations. The future of AI in finance hinges on a realistic assessment of its capabilities and a commitment to responsible, sustainable adoption.
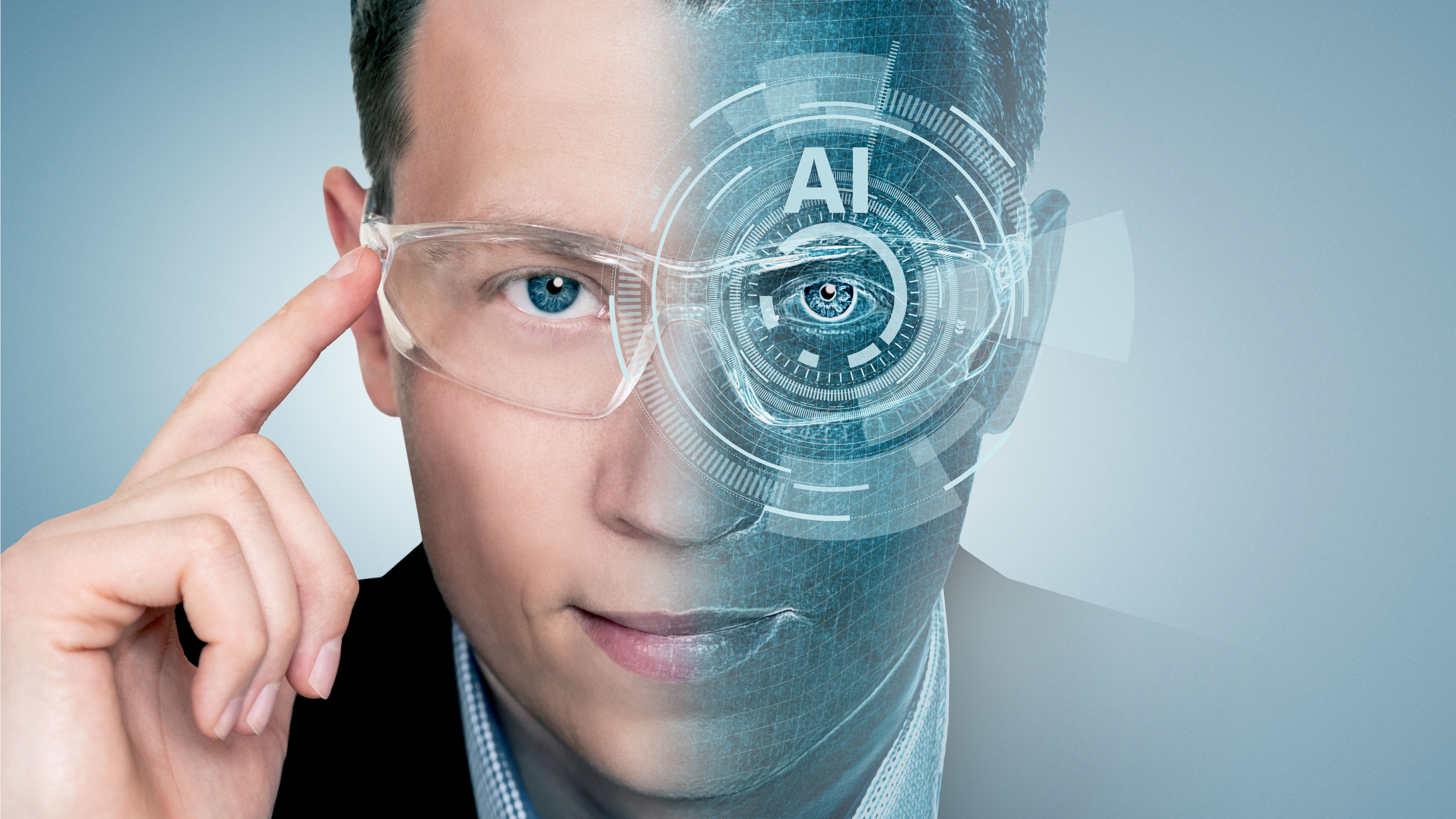
Thank you for visiting our website, your trusted source for the latest updates and in-depth coverage on AI Value Crisis: Global Finance Grapples With Costly Implementations. We're committed to keeping you informed with timely and accurate information to meet your curiosity and needs.
If you have any questions, suggestions, or feedback, we'd love to hear from you. Your insights are valuable to us and help us improve to serve you better. Feel free to reach out through our contact page.
Don't forget to bookmark our website and check back regularly for the latest headlines and trending topics. See you next time, and thank you for being part of our growing community!
Featured Posts
-
Mars Mapmakers Their Rivalry And Lasting Legacy
May 06, 2025 -
The Last Of Us Season 2 Explained A Guide To New Characters And Plot Points
May 06, 2025 -
Ge 2025 Wp Supporters React To Unexpected Election Results
May 06, 2025 -
Safety Alert Popular Kids Item Removed Due To Defect
May 06, 2025 -
1972 Soviet Satellite To Crash Land Global Impact Zone
May 06, 2025
Latest Posts
-
May 5 2025 Nuggets Vs Thunder Play By Play And Box Score
May 06, 2025 -
The New Name For Marvels Thunderbolts A Bold Marketing Gamble
May 06, 2025 -
Revolutionizing Web3 Gaming New Stablecoin Launches Exclusively On Sui
May 06, 2025 -
Palantirs Increased Revenue Projection An Ai Driven Boost Or Investor Disappointment
May 06, 2025 -
New Website Name A Worthy Sequel Or A Disappointing Follow Up
May 06, 2025