Assessing The True Impact Of Reinforcement Learning On AI
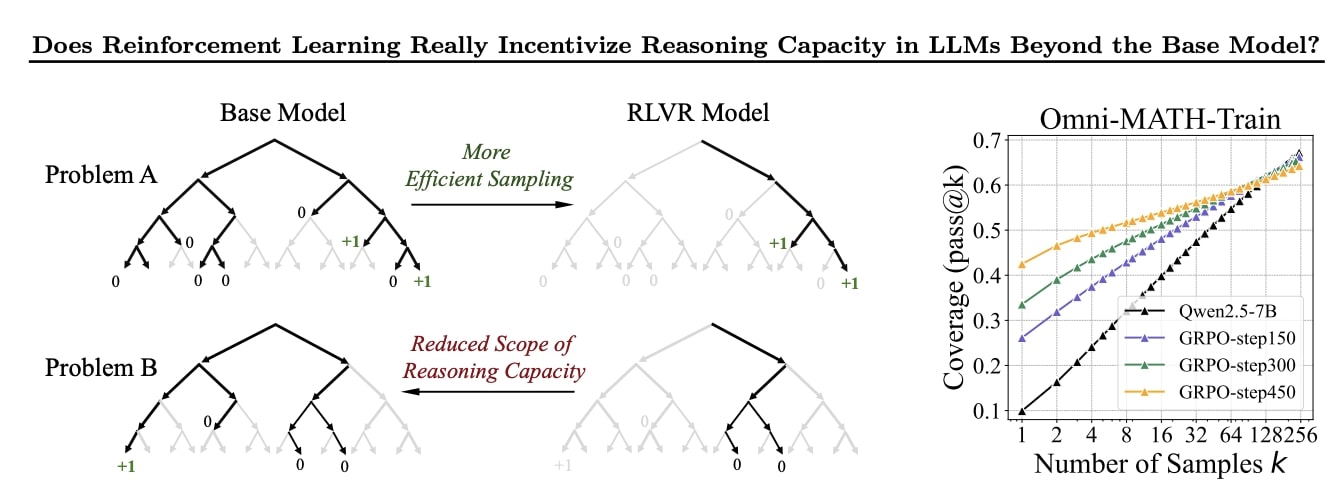
Welcome to your ultimate source for breaking news, trending updates, and in-depth stories from around the world. Whether it's politics, technology, entertainment, sports, or lifestyle, we bring you real-time updates that keep you informed and ahead of the curve.
Our team works tirelessly to ensure you never miss a moment. From the latest developments in global events to the most talked-about topics on social media, our news platform is designed to deliver accurate and timely information, all in one place.
Stay in the know and join thousands of readers who trust us for reliable, up-to-date content. Explore our expertly curated articles and dive deeper into the stories that matter to you. Visit NewsOneSMADCSTDO now and be part of the conversation. Don't miss out on the headlines that shape our world!
Table of Contents
Assessing the True Impact of Reinforcement Learning on AI: Beyond the Hype
Reinforcement learning (RL), a subfield of machine learning, has exploded in popularity, promising revolutionary advancements in artificial intelligence. From self-driving cars navigating complex traffic patterns to robots mastering intricate manipulation tasks, the applications seem limitless. But beyond the hype, what is the true impact of RL on AI, and where are its limitations? This article delves into the current state of RL, exploring its successes, challenges, and future potential.
H2: The Triumphs of Reinforcement Learning:
Reinforcement learning's success stems from its ability to train agents to achieve complex goals through trial and error. Unlike supervised learning, which relies on labeled data, RL agents learn by interacting with their environment, receiving rewards for desirable actions and penalties for undesirable ones. This iterative process allows for the development of sophisticated strategies and behaviors that would be difficult, if not impossible, to program explicitly.
- Game Playing: DeepMind's AlphaGo, which famously defeated a world champion Go player, is a prime example of RL's power. This victory showcased the potential of RL to tackle problems previously considered intractable for AI.
- Robotics: RL is transforming robotics, enabling robots to learn complex manipulation skills, navigate dynamic environments, and collaborate with humans. Applications range from warehouse automation to surgical assistance.
- Resource Management: In fields like energy and transportation, RL algorithms are being used to optimize resource allocation, leading to increased efficiency and reduced costs.
H2: The Challenges Facing Reinforcement Learning:
Despite its impressive achievements, RL faces significant challenges that limit its widespread adoption:
- Data Efficiency: RL algorithms often require vast amounts of data and extensive training time, making them computationally expensive and impractical for many real-world applications.
- Sample Inefficiency: The trial-and-error nature of RL can lead to significant sample inefficiency, meaning that the agent might need to explore many unsuccessful actions before finding an optimal strategy.
- Safety and Robustness: Ensuring the safety and robustness of RL agents is crucial, especially in applications with high stakes, such as autonomous driving. Unforeseen situations can lead to unpredictable and potentially dangerous behavior.
- Interpretability and Explainability: Understanding why an RL agent makes a particular decision can be difficult, hindering trust and making it challenging to debug or improve the system. The "black box" nature of some RL models poses a significant challenge.
H2: The Future of Reinforcement Learning in AI:
Despite these challenges, the future of reinforcement learning in AI remains bright. Ongoing research focuses on addressing the limitations outlined above, with promising advancements in:
- Transfer Learning: Developing techniques that allow RL agents to transfer knowledge learned in one environment to another, improving data efficiency and reducing training time.
- Imitation Learning: Combining RL with imitation learning, where the agent learns by observing human demonstrations, can improve sample efficiency and safety.
- Safe RL: Developing methods to ensure the safety and robustness of RL agents, such as incorporating constraints and safety mechanisms into the learning process.
- Explainable RL: Developing techniques to make RL models more interpretable and explainable, increasing trust and facilitating debugging.
H2: Conclusion:
Reinforcement learning represents a powerful paradigm shift in AI, enabling the development of intelligent agents capable of tackling complex tasks. While challenges remain, ongoing research is paving the way for wider adoption and even more impressive achievements. The true impact of RL on AI is still unfolding, but its potential to revolutionize various industries is undeniable. As researchers overcome the limitations of current RL techniques, we can expect to see even more transformative applications in the years to come. The journey is ongoing, but the destination promises a future where AI plays an even more significant role in solving global challenges.
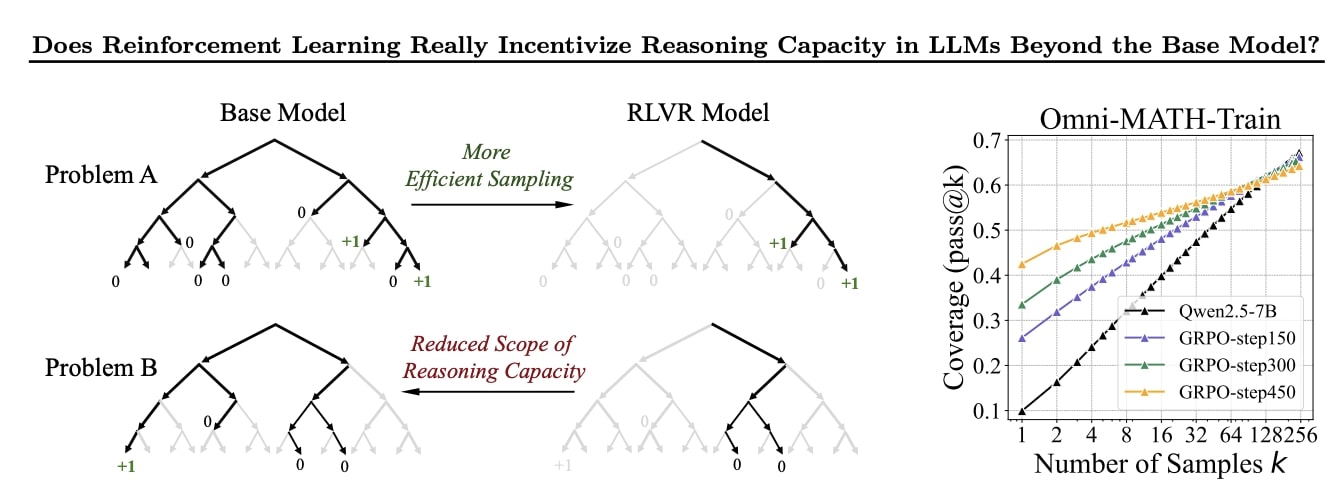
Thank you for visiting our website, your trusted source for the latest updates and in-depth coverage on Assessing The True Impact Of Reinforcement Learning On AI. We're committed to keeping you informed with timely and accurate information to meet your curiosity and needs.
If you have any questions, suggestions, or feedback, we'd love to hear from you. Your insights are valuable to us and help us improve to serve you better. Feel free to reach out through our contact page.
Don't forget to bookmark our website and check back regularly for the latest headlines and trending topics. See you next time, and thank you for being part of our growing community!
Featured Posts
-
Pittsburgh Starts Padres Extensive Road Trip A Preview
May 03, 2025 -
The Walking Dead Dead City Lauren Cohan Discusses Filming In Massachusetts
May 03, 2025 -
Review The First Half Of The Walking Dead Dead City Season 2
May 03, 2025 -
May 2nd Mlb Picks Analyzing Fernando Tatis Jr S Sleeper Potential
May 03, 2025 -
Top 25 College Softball Week 12 Results And Updated Rankings Analysis
May 03, 2025
Latest Posts
-
Comparing Endings Beyond Paradise Season 3 Vs Death In Paradise A Key Difference
May 03, 2025 -
Re Election Bid Albaneses Challenges And The Coalitions Hopes
May 03, 2025 -
Watch Indiana Fever Vs Brazil Womens National Team Free Online Streaming Guide
May 03, 2025 -
Significant Wwe Releases Strowman Kai And Others Let Go
May 03, 2025 -
Samsung Galaxy Z Flip 7 What To Expect In Specs
May 03, 2025