Balancing Innovation And Accuracy: Addressing Hallucinations In Advanced AI Like ChatGPT
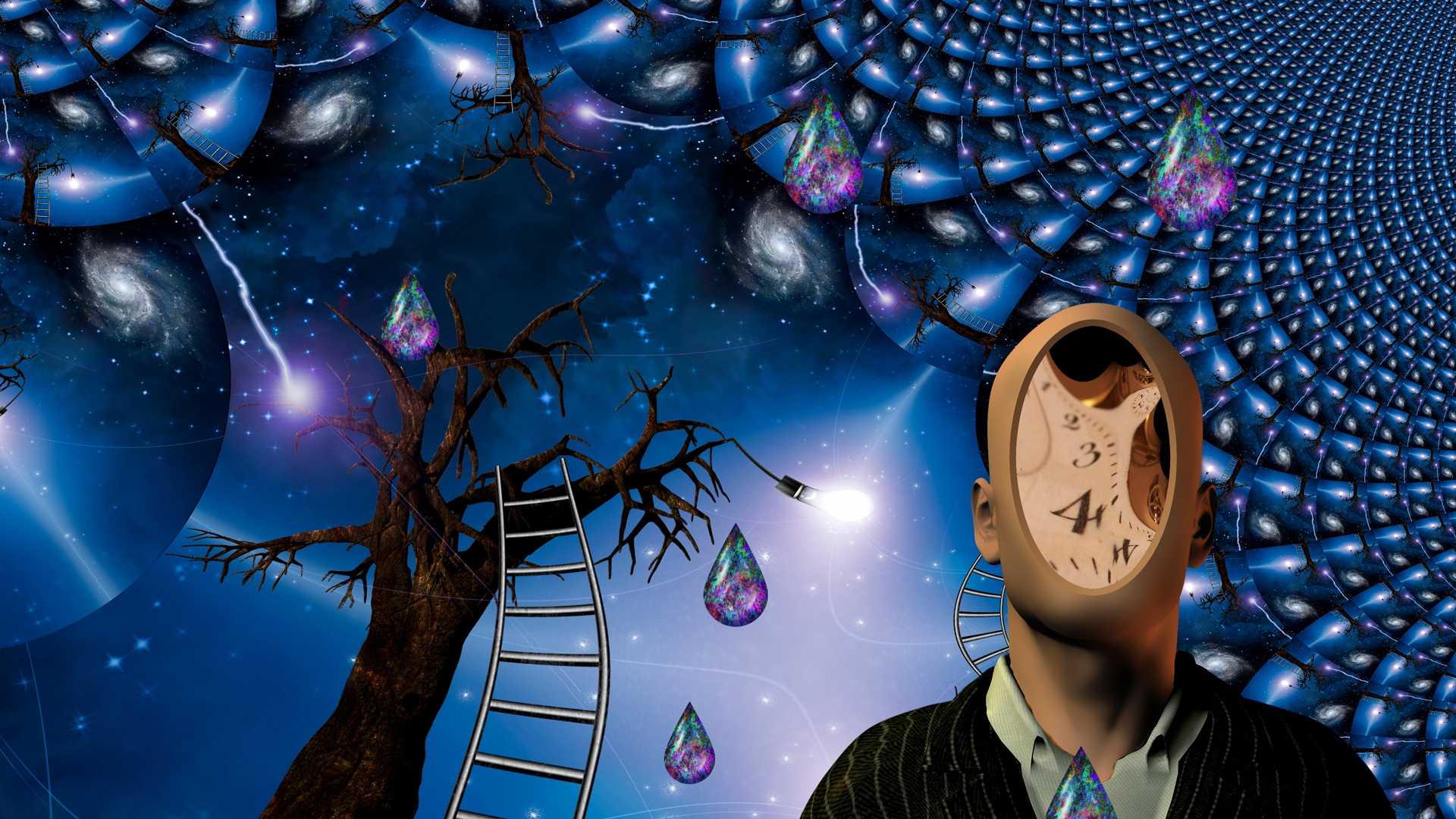
Welcome to your ultimate source for breaking news, trending updates, and in-depth stories from around the world. Whether it's politics, technology, entertainment, sports, or lifestyle, we bring you real-time updates that keep you informed and ahead of the curve.
Our team works tirelessly to ensure you never miss a moment. From the latest developments in global events to the most talked-about topics on social media, our news platform is designed to deliver accurate and timely information, all in one place.
Stay in the know and join thousands of readers who trust us for reliable, up-to-date content. Explore our expertly curated articles and dive deeper into the stories that matter to you. Visit NewsOneSMADCSTDO now and be part of the conversation. Don't miss out on the headlines that shape our world!
Table of Contents
Balancing Innovation and Accuracy: Addressing Hallucinations in Advanced AI like ChatGPT
The rise of advanced AI models like ChatGPT has ushered in an era of unprecedented technological progress. These powerful tools can generate human-quality text, translate languages, and even write different kinds of creative content. However, a significant challenge remains: the tendency of these AI systems to produce factually incorrect or nonsensical information, a phenomenon known as "hallucination." This article delves into the nature of AI hallucinations, their impact, and the ongoing efforts to mitigate this critical limitation.
What are AI Hallucinations?
AI hallucinations occur when a large language model (LLM) like ChatGPT generates outputs that are confidently presented as factual but are entirely fabricated or distorted. These aren't simply minor inaccuracies; they can be elaborate, convincing falsehoods that lack any basis in reality. This can stem from various factors, including:
- Data Bias: AI models are trained on massive datasets, and if these datasets contain biases or inaccuracies, the model will inherit and amplify them.
- Lack of Real-World Understanding: LLMs lack genuine understanding of the world; they identify patterns and relationships in data but don't possess genuine knowledge or common sense.
- Statistical Probability over Meaning: The model prioritizes statistically likely word sequences over semantic coherence or factual accuracy. It chooses the most probable next word, regardless of whether it creates a logically sound statement.
- Overfitting: The model may overfit to the training data, memorizing specific patterns without generalizing to new, unseen information.
The Impact of AI Hallucinations:
The consequences of AI hallucinations are far-reaching and potentially damaging:
- Misinformation and Disinformation: Hallucinations can contribute to the spread of false information, impacting public opinion and potentially influencing critical decisions.
- Erosion of Trust: The ability of AI to convincingly generate falsehoods erodes public trust in both AI technology and information sources in general.
- Safety Concerns: In high-stakes applications like medical diagnosis or financial advice, hallucinations could have serious, even life-threatening, consequences.
- Ethical Dilemmas: The generation of fabricated information raises significant ethical concerns about accountability, transparency, and the potential for misuse.
Mitigating AI Hallucinations: Current Strategies and Future Directions
Researchers and developers are actively working on strategies to reduce AI hallucinations. These include:
- Improved Training Data: Focus on using higher-quality, more diverse, and thoroughly fact-checked training datasets.
- Reinforcement Learning from Human Feedback (RLHF): Training models to better align with human values and preferences by incorporating human feedback during training.
- Fact Verification and External Knowledge Bases: Integrating external knowledge bases and fact-checking mechanisms to verify the information generated by the model.
- Transparency and Explainability: Developing methods to make the AI's reasoning process more transparent, allowing users to understand how the model arrived at its conclusions.
- Better Prompt Engineering: Carefully crafting prompts to guide the model towards more accurate and relevant responses.
Conclusion:
While AI models like ChatGPT offer incredible potential, addressing the issue of hallucinations is crucial for their responsible and safe deployment. The ongoing research and development efforts focused on improving data quality, training methodologies, and model architecture are vital steps towards ensuring that these powerful tools are used for good and contribute to a more informed and trustworthy information landscape. The future of AI depends on striking a careful balance between innovation and accuracy, ensuring that the benefits of this technology outweigh its potential risks.
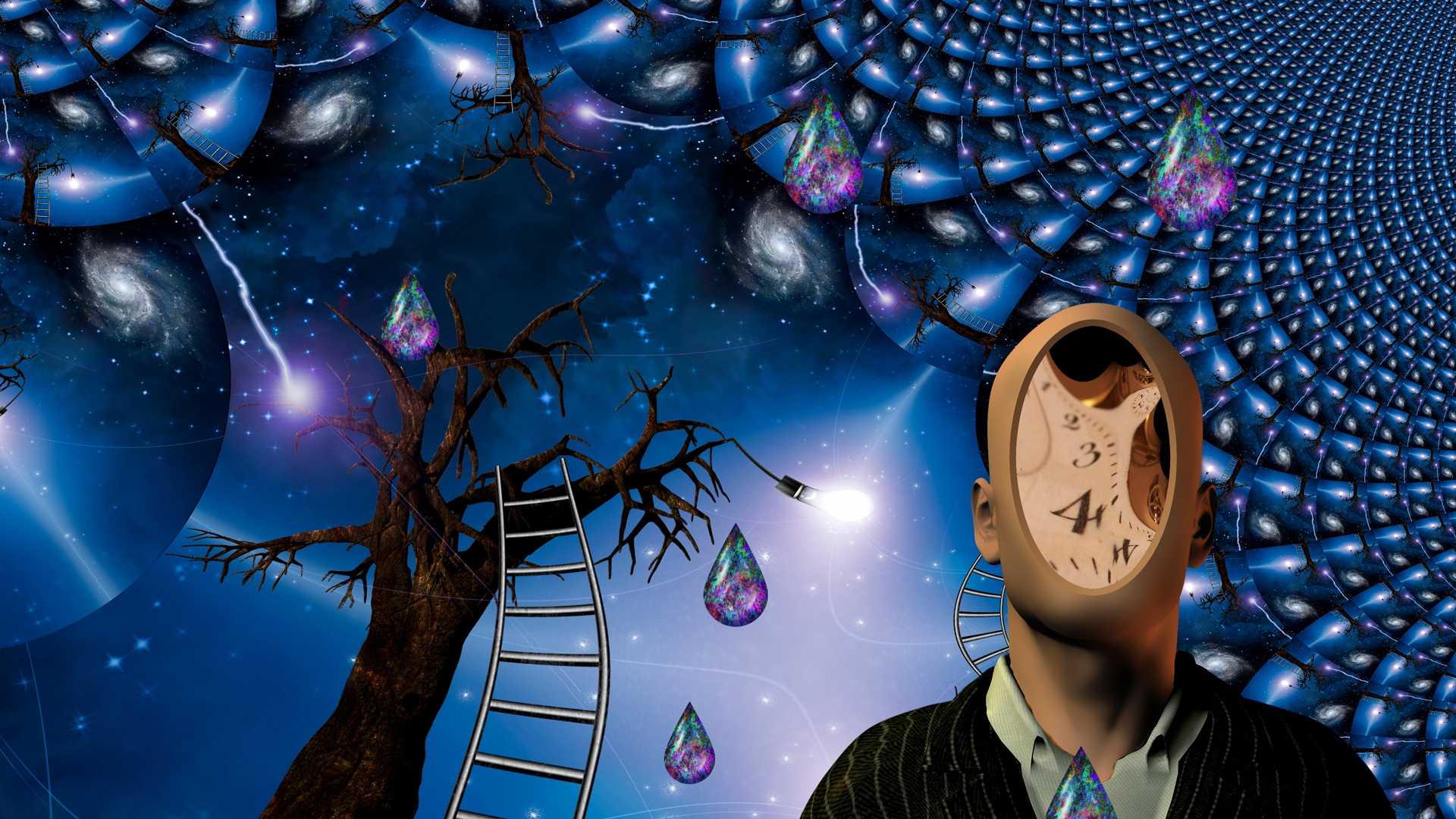
Thank you for visiting our website, your trusted source for the latest updates and in-depth coverage on Balancing Innovation And Accuracy: Addressing Hallucinations In Advanced AI Like ChatGPT. We're committed to keeping you informed with timely and accurate information to meet your curiosity and needs.
If you have any questions, suggestions, or feedback, we'd love to hear from you. Your insights are valuable to us and help us improve to serve you better. Feel free to reach out through our contact page.
Don't forget to bookmark our website and check back regularly for the latest headlines and trending topics. See you next time, and thank you for being part of our growing community!
Featured Posts
-
Stablecoin Integration A New Strategy For Banks To Enhance Liquidity And Customer Deposits
May 08, 2025 -
Reserve Bank Holds Interest Rates April Decision Impacts Households
May 08, 2025 -
Oklahoma City Thunder At Denver Nuggets Post Game Report May 7 2025
May 08, 2025 -
Heat Anticipate Significant Improvement From Wiggins In 2024
May 08, 2025 -
28 Years Later Filming Locations Where Was It Shot
May 08, 2025
Latest Posts
-
Setor Publico Em Greve Consequencias Financeiras Para Empresas E Setores
May 08, 2025 -
Black Rock Bitcoin Etf 530 Million Influx Ethereum Funds Remain Static
May 08, 2025 -
Uk Foreign Office Issues New Travel Advisory Popular Holiday Spot Now High Risk
May 08, 2025 -
New Research Reproducing Taste The Science Behind The Sensation
May 08, 2025 -
Get Your 200 Cost Of Living Payment Early This Summer
May 08, 2025