Debunking The Hype: Reinforcement Learning And Its Impact On AI Model Performance.
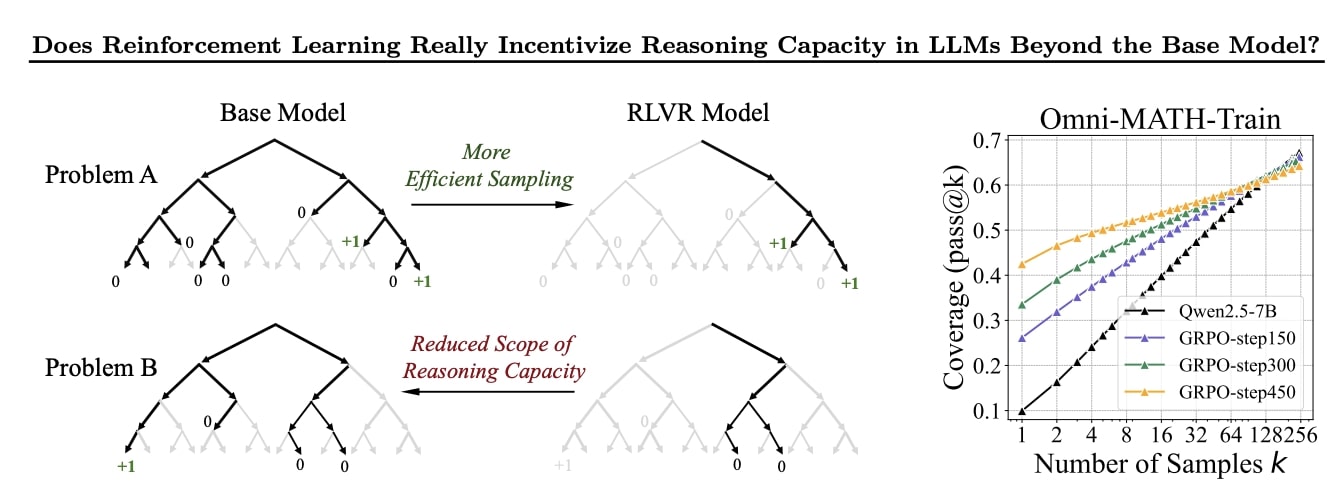
Welcome to your ultimate source for breaking news, trending updates, and in-depth stories from around the world. Whether it's politics, technology, entertainment, sports, or lifestyle, we bring you real-time updates that keep you informed and ahead of the curve.
Our team works tirelessly to ensure you never miss a moment. From the latest developments in global events to the most talked-about topics on social media, our news platform is designed to deliver accurate and timely information, all in one place.
Stay in the know and join thousands of readers who trust us for reliable, up-to-date content. Explore our expertly curated articles and dive deeper into the stories that matter to you. Visit NewsOneSMADCSTDO now and be part of the conversation. Don't miss out on the headlines that shape our world!
Table of Contents
Debunking the Hype: Reinforcement Learning and its Impact on AI Model Performance
Reinforcement learning (RL) has exploded in popularity, promising revolutionary advancements in AI. From self-driving cars to game-playing AI, the applications seem limitless. But amidst the hype, a crucial question remains: Does RL truly deliver on its promised impact on AI model performance, or is it overblown? This article delves into the reality of RL's effectiveness, separating fact from fiction.
The Allure of Reinforcement Learning
Reinforcement learning distinguishes itself from other machine learning paradigms by focusing on agent-environment interaction. An RL agent learns through trial and error, receiving rewards for desirable actions and penalties for undesirable ones. This iterative process allows the agent to optimize its behavior over time, achieving complex goals without explicit programming. This inherent adaptability is a major draw, fueling expectations of superior AI model performance across various domains.
RL's Strengths: Where it Truly Shines
While the hype surrounding RL might be excessive in some areas, its strengths are undeniable. Several key applications demonstrate its effectiveness:
-
Game Playing: RL's success in games like Go and chess is undeniable. AlphaGo's victory over world champions showcased the power of RL to master complex strategic games. This success highlights RL's ability to discover optimal strategies in highly intricate environments.
-
Robotics: RL has proven valuable in training robots to perform complex tasks, such as walking, grasping objects, and navigating dynamic environments. The ability to learn through interaction eliminates the need for extensive manual programming, leading to more adaptable and robust robotic systems.
-
Personalized Recommendations: RL algorithms are increasingly used to power personalized recommendations in various applications, from e-commerce to streaming services. By learning user preferences through interaction, RL systems can deliver highly tailored experiences, boosting user engagement and satisfaction.
The Limitations: Understanding RL's Challenges
Despite its impressive achievements, RL faces significant challenges that temper expectations regarding its universal superiority:
-
Data Efficiency: RL often requires vast amounts of data for effective training. This can be a significant bottleneck, especially in domains where data collection is expensive or time-consuming. This contrasts with supervised learning methods, which can sometimes achieve comparable performance with less data.
-
Reward Engineering: Designing effective reward functions is crucial for successful RL. Poorly designed rewards can lead to unintended behaviors, hindering the agent's ability to learn the desired task. This "reward hacking" problem remains a significant research area.
-
Sample Inefficiency: RL algorithms can be computationally expensive and require a large number of interactions with the environment to converge on an optimal solution. This sample inefficiency can make RL impractical for applications with limited computational resources or real-time constraints.
-
Interpretability: Understanding why an RL agent makes a particular decision can be challenging. The lack of interpretability can be a major drawback in applications where understanding the reasoning behind AI decisions is critical, such as healthcare or finance.
RL's Place in the AI Landscape: A Balanced Perspective
Reinforcement learning is a powerful tool with significant potential to improve AI model performance, particularly in domains requiring adaptive behavior and strategic decision-making. However, it's crucial to acknowledge its limitations. RL is not a silver bullet; its effectiveness depends heavily on the specific application, the availability of data, and the careful design of the reward function. A balanced perspective, acknowledging both its strengths and weaknesses, is essential for responsible development and deployment of RL-based AI systems. Future research focusing on improving data efficiency, addressing reward engineering challenges, and enhancing interpretability will be vital in unlocking the full potential of reinforcement learning and achieving its true impact on AI model performance.
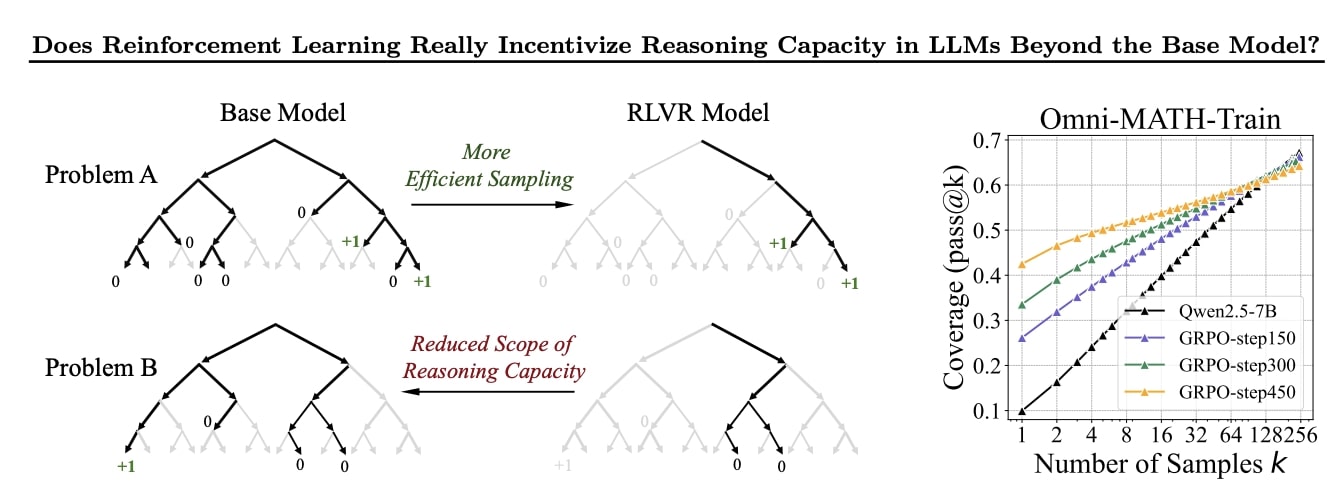
Thank you for visiting our website, your trusted source for the latest updates and in-depth coverage on Debunking The Hype: Reinforcement Learning And Its Impact On AI Model Performance.. We're committed to keeping you informed with timely and accurate information to meet your curiosity and needs.
If you have any questions, suggestions, or feedback, we'd love to hear from you. Your insights are valuable to us and help us improve to serve you better. Feel free to reach out through our contact page.
Don't forget to bookmark our website and check back regularly for the latest headlines and trending topics. See you next time, and thank you for being part of our growing community!
Featured Posts
-
Toll Road Text Scam Protecting Yourself From Phishing Attempts
Apr 29, 2025 -
23 Year Old Steelers Cornerback Ryan Watts Medically Retired Due To Neck Injury
Apr 29, 2025 -
El Movimiento De Buffett Venta De Acciones De Apple Y Explicacion De La Disminucion Del 13
Apr 29, 2025 -
Ge 2025 Fullerton Rally Live Stream Uob Plaza Paps Opening Event
Apr 29, 2025 -
William Lai Faces Backlash Huge Anti Government Protest Rocks Taiwan
Apr 29, 2025
Latest Posts
-
Investigation Into Large Scale Power Failure In Spain And Portugal Cyberattack Ruled Out
Apr 29, 2025 -
Election 2025 A Deep Dive Into Public Housing Policies
Apr 29, 2025 -
Virat Kohli And Kl Rahuls On Field Argument Umpires Decision At The Center
Apr 29, 2025 -
Rajasthan Royals Vs Gujarat Titans Afghanistans Growing Ipl Presence
Apr 29, 2025 -
Ellie Taylor And Joel Dommett The Latest Additions To Bbc Radio 2
Apr 29, 2025