Debunking The Hype: Reinforcement Learning's Impact On AI Model Improvement
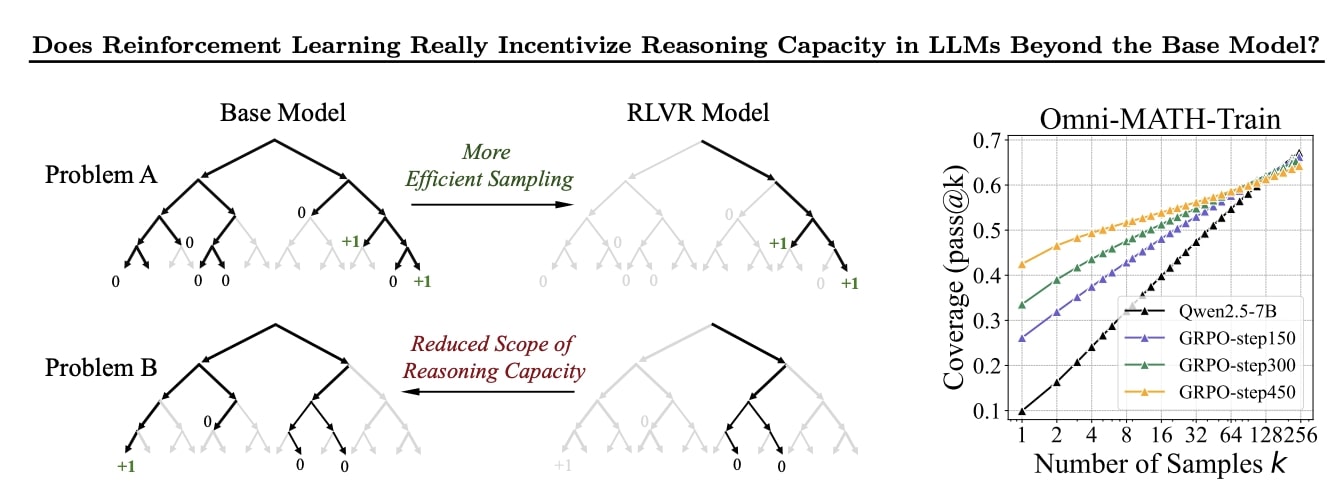
Welcome to your ultimate source for breaking news, trending updates, and in-depth stories from around the world. Whether it's politics, technology, entertainment, sports, or lifestyle, we bring you real-time updates that keep you informed and ahead of the curve.
Our team works tirelessly to ensure you never miss a moment. From the latest developments in global events to the most talked-about topics on social media, our news platform is designed to deliver accurate and timely information, all in one place.
Stay in the know and join thousands of readers who trust us for reliable, up-to-date content. Explore our expertly curated articles and dive deeper into the stories that matter to you. Visit NewsOneSMADCSTDO now and be part of the conversation. Don't miss out on the headlines that shape our world!
Table of Contents
Debunking the Hype: Reinforcement Learning's Impact on AI Model Improvement
Reinforcement learning (RL) has exploded in popularity, often touted as the silver bullet for significantly improving AI model performance. While its potential is undeniable, the reality is more nuanced. This article delves into the actual impact of RL on AI model improvement, separating fact from fiction and exploring its limitations alongside its successes.
H2: What is Reinforcement Learning and How Does it Work?
Before dissecting the hype, let's establish a clear understanding. Reinforcement learning is a type of machine learning where an agent learns to interact with an environment by receiving rewards or penalties for its actions. This trial-and-error approach allows the agent to optimize its behavior over time, aiming for maximum cumulative reward. Think of it like training a dog – rewarding good behavior and discouraging bad behavior until it learns the desired actions.
This differs significantly from supervised learning (using labeled data) and unsupervised learning (finding patterns in unlabeled data). RL's strength lies in its ability to handle complex, dynamic environments where the optimal action isn't immediately obvious.
H2: Reinforcement Learning's Proven Successes in AI Model Improvement:
RL has undeniably yielded impressive results in specific domains:
- Game Playing: DeepMind's AlphaGo famously defeated a world champion Go player, showcasing RL's prowess in mastering complex strategic games. Similar successes have been seen in chess, shogi, and other challenging games.
- Robotics: RL is proving invaluable in training robots to perform intricate tasks, from navigating complex terrains to manipulating objects with dexterity. This is particularly useful in manufacturing, logistics, and exploration.
- Resource Optimization: RL algorithms are being employed to optimize resource allocation in areas like energy grids, traffic management, and supply chain logistics, leading to increased efficiency and cost savings.
- Personalized Recommendations: RL can personalize recommendations in areas like online advertising, e-commerce, and streaming services by learning user preferences and providing tailored experiences.
H2: The Limitations and Unrealistic Expectations of Reinforcement Learning:
Despite its successes, it's crucial to acknowledge RL's limitations:
- Data Efficiency: RL often requires vast amounts of data and extensive training time, making it computationally expensive and potentially impractical for certain applications.
- Reward Function Design: Defining an appropriate reward function is crucial, but it can be challenging and highly domain-specific. A poorly designed reward function can lead to unexpected and undesirable behaviors.
- Sample Inefficiency: RL agents can struggle to learn efficiently, requiring many trials and errors before converging on an optimal solution. This can be particularly problematic in real-world scenarios where experimentation is costly or risky.
- Generalization Challenges: RL agents often struggle to generalize their learned behavior to new, unseen environments or situations. This limits their applicability to dynamic and unpredictable real-world scenarios.
- Safety Concerns: In safety-critical applications, ensuring the reliability and safety of RL agents is paramount. Unpredictable or unintended behavior can have severe consequences.
H2: The Future of Reinforcement Learning in AI Model Improvement:
While the hype surrounding RL might be overblown in some aspects, its potential remains significant. Ongoing research focuses on:
- Improving data efficiency: Developing algorithms that learn more efficiently from limited data.
- Robust reward function design: Creating methods to automatically learn or adapt reward functions.
- Enhanced safety and reliability: Developing techniques to ensure the safe and reliable operation of RL agents.
- Transfer learning: Enabling RL agents to transfer knowledge learned in one environment to another.
H2: Conclusion:
Reinforcement learning is a powerful tool for improving AI models, but it's not a magic bullet. Its successes are significant, but its limitations must be carefully considered. By tempering expectations and focusing on addressing its challenges, we can unlock its full potential and harness its power to create truly intelligent and adaptable AI systems. The future of AI model improvement hinges on a realistic understanding of RL's capabilities and limitations, leading to more focused and impactful research and development.
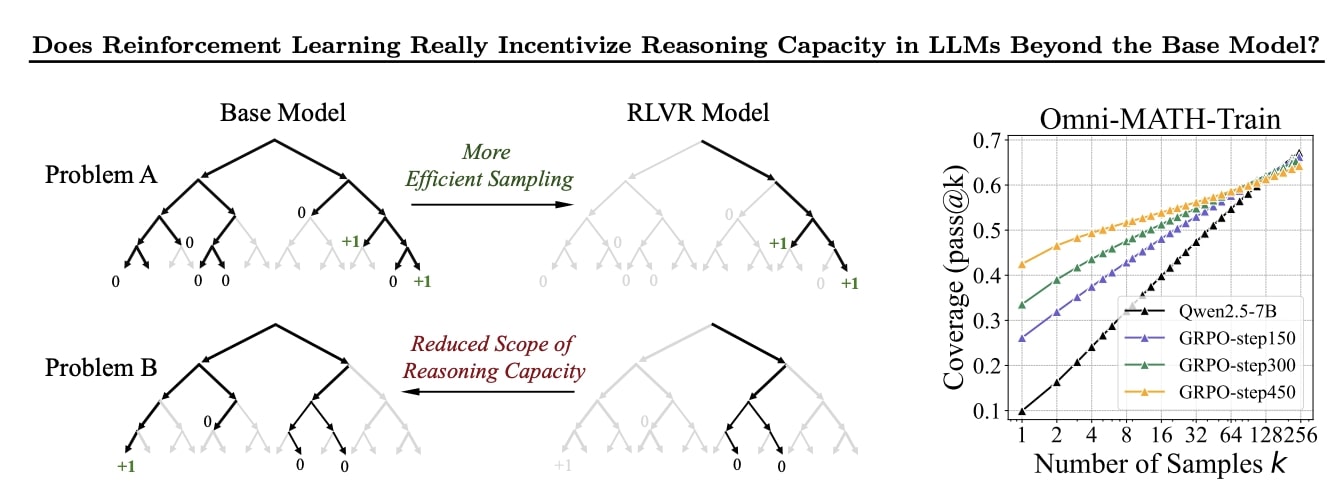
Thank you for visiting our website, your trusted source for the latest updates and in-depth coverage on Debunking The Hype: Reinforcement Learning's Impact On AI Model Improvement. We're committed to keeping you informed with timely and accurate information to meet your curiosity and needs.
If you have any questions, suggestions, or feedback, we'd love to hear from you. Your insights are valuable to us and help us improve to serve you better. Feel free to reach out through our contact page.
Don't forget to bookmark our website and check back regularly for the latest headlines and trending topics. See you next time, and thank you for being part of our growing community!
Featured Posts
-
Meghan Markle Interview Abruptly Ended By Whoopi Goldberg
May 02, 2025 -
Close To Success Man Utd Player On Amorims Positive Influence
May 02, 2025 -
Dutton Concedes Possible Coalition Loss Amidst Favorable Labor Polls
May 02, 2025 -
Reddit Beats Revenue Estimates Digital Ad Spending Fuels Growth
May 02, 2025 -
Emma Hayes Legacy And Lauren James Rise Understanding Chelseas Continued Wsl Success
May 02, 2025
Latest Posts
-
Predicting 2025 Rookie Rb Production A Fantasy Football Guide To Jeanty Henderson Etc
May 02, 2025 -
Live Football Man Utd Tottenham Chelseas European Fixtures Scores And Radio
May 02, 2025 -
Pacers Win Overshadowed Tyrese Haliburtons Father Apologizes For Giannis Confrontation
May 02, 2025 -
Economia Brasileira Em Foco Analise Do Copom Ipca E Perspectivas Da Industria
May 02, 2025 -
Link11 Unifies Three Brands Under Single Platform With Rebranding
May 02, 2025