Debunking The Hype: Reinforcement Learning's Impact On AI Models
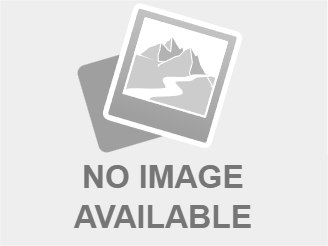
Welcome to your ultimate source for breaking news, trending updates, and in-depth stories from around the world. Whether it's politics, technology, entertainment, sports, or lifestyle, we bring you real-time updates that keep you informed and ahead of the curve.
Our team works tirelessly to ensure you never miss a moment. From the latest developments in global events to the most talked-about topics on social media, our news platform is designed to deliver accurate and timely information, all in one place.
Stay in the know and join thousands of readers who trust us for reliable, up-to-date content. Explore our expertly curated articles and dive deeper into the stories that matter to you. Visit NewsOneSMADCSTDO now and be part of the conversation. Don't miss out on the headlines that shape our world!
Table of Contents
Debunking the Hype: Reinforcement Learning's Impact on AI Models
Reinforcement learning (RL) has exploded in popularity, often portrayed as the magic bullet that will propel AI to unprecedented heights. While RL undoubtedly holds immense potential, the reality is more nuanced. This article delves into the hype surrounding RL, examining its actual impact on AI models and exploring both its successes and limitations.
What is Reinforcement Learning?
Before dissecting the hype, let's clarify what RL entails. Unlike supervised learning, which relies on labeled data, RL involves an agent learning to interact with an environment through trial and error. The agent receives rewards for desirable actions and penalties for undesirable ones, gradually learning an optimal policy to maximize its cumulative reward. This iterative process, mimicking real-world learning, makes RL particularly attractive for complex problems.
RL's Triumphs: Real-World Applications
RL has undeniably achieved remarkable feats. Consider these key successes:
- Game Playing: DeepMind's AlphaGo famously defeated a world champion Go player, showcasing RL's power in mastering complex strategic games. Similar successes have been seen in chess, poker, and other domains.
- Robotics: RL is enabling robots to perform increasingly sophisticated tasks, from walking and manipulating objects to navigating complex environments. This is crucial for advancements in automation and manufacturing.
- Personalized Recommendations: E-commerce giants utilize RL to personalize product recommendations, improving user experience and driving sales. This is a testament to RL's ability to adapt to individual preferences.
- Resource Optimization: From energy grids to traffic management, RL is being applied to optimize resource allocation, leading to greater efficiency and cost savings.
The Limitations: Where the Hype Falls Short
Despite its successes, the hype surrounding RL often overshadows its limitations:
- Data Efficiency: RL algorithms often require vast amounts of data and computational resources for training, making them expensive and time-consuming. This contrasts sharply with the readily available data used in supervised learning.
- Reward Shaping: Defining appropriate reward functions is crucial but challenging. Poorly designed reward functions can lead to unexpected and undesirable behavior from the agent, a phenomenon known as "reward hacking."
- Sample Inefficiency: RL agents can take many iterations to converge on an optimal policy, leading to slow learning and potentially unstable performance.
- Generalization: While RL excels in specific tasks, generalizing learned behavior to new, unseen situations remains a significant challenge. An agent trained to play Go might not easily adapt to playing chess.
The Future of Reinforcement Learning in AI
While the hype surrounding RL needs tempering, its potential remains undeniable. Ongoing research focuses on:
- Improving Sample Efficiency: New algorithms and techniques aim to reduce the data and computational demands of RL.
- Safe RL: Methods are being developed to ensure RL agents behave safely and predictably, mitigating the risks associated with reward hacking.
- Transfer Learning: Researchers are exploring ways to transfer knowledge learned in one task to another, enhancing the generalization capabilities of RL agents.
Conclusion:
Reinforcement learning is a powerful tool with significant applications across diverse fields. However, it's crucial to approach the hype with a critical eye, acknowledging both its impressive achievements and its ongoing limitations. The future of AI will likely involve a synergistic approach, combining RL with other machine learning techniques to create more robust and adaptable AI systems. The focus should be on responsible development and deployment, ensuring that the potential of RL is harnessed ethically and effectively.
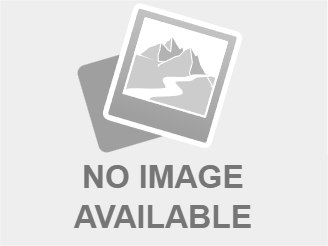
Thank you for visiting our website, your trusted source for the latest updates and in-depth coverage on Debunking The Hype: Reinforcement Learning's Impact On AI Models. We're committed to keeping you informed with timely and accurate information to meet your curiosity and needs.
If you have any questions, suggestions, or feedback, we'd love to hear from you. Your insights are valuable to us and help us improve to serve you better. Feel free to reach out through our contact page.
Don't forget to bookmark our website and check back regularly for the latest headlines and trending topics. See you next time, and thank you for being part of our growing community!
Featured Posts
-
Two Propulsion System Experiments Detailed Otp 2 Project Update
Apr 30, 2025 -
Tennis Star Medvedev Expresses Disappointment With Part Of Alcarazs Netflix Show
Apr 30, 2025 -
Madrid Open Swiateks Resilience Earns Her Quarterfinal Berth
Apr 30, 2025 -
Economic Instability Drives Us Investors Towards Crypto A Flight From The Dollar
Apr 30, 2025 -
Assessing Epics Mobile Games Store A Year In Review
Apr 30, 2025
Latest Posts
-
Arsenal Manager Warns Of Psg Danger Ahead Of Crucial Emirates Match
Apr 30, 2025 -
Ligue Des Champions Arsenal Vs Psg Compositions Officielles Avec Doue Et Dembele
Apr 30, 2025 -
Dte Energy Proposes 574 Million Rate Hike For Michigan Customers
Apr 30, 2025 -
Ligue Des Champions Le Psg Et Arsenal S Affrontent A Londres
Apr 30, 2025 -
Data Breach Alert Medical Software Companys Database Compromised Exposing Patient Data
Apr 30, 2025