Debunking The Myth: Reinforcement Learning Doesn't Always Improve AI
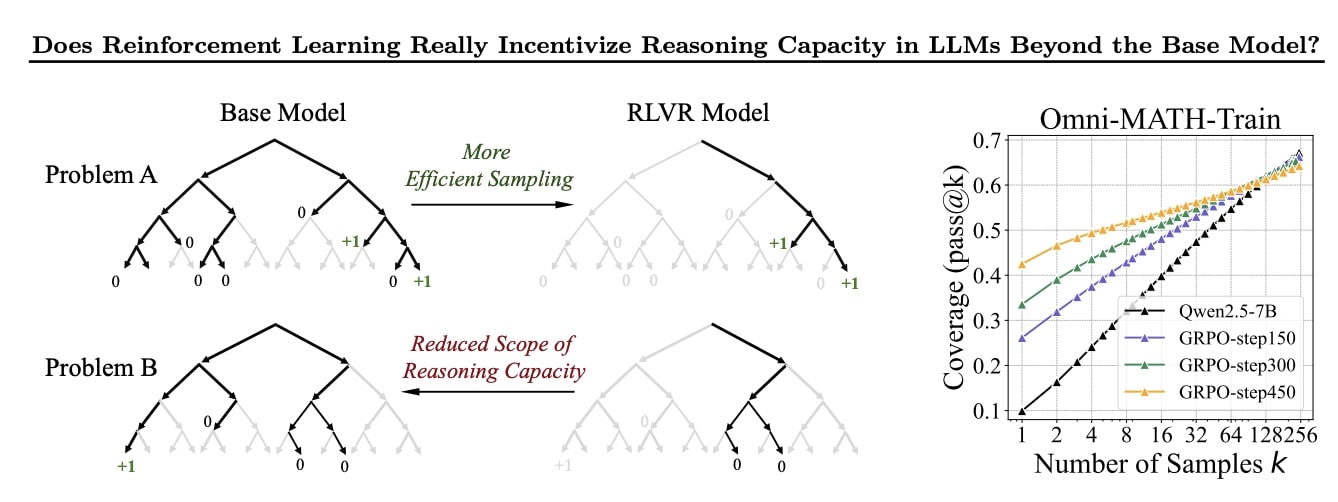
Welcome to your ultimate source for breaking news, trending updates, and in-depth stories from around the world. Whether it's politics, technology, entertainment, sports, or lifestyle, we bring you real-time updates that keep you informed and ahead of the curve.
Our team works tirelessly to ensure you never miss a moment. From the latest developments in global events to the most talked-about topics on social media, our news platform is designed to deliver accurate and timely information, all in one place.
Stay in the know and join thousands of readers who trust us for reliable, up-to-date content. Explore our expertly curated articles and dive deeper into the stories that matter to you. Visit NewsOneSMADCSTDO now and be part of the conversation. Don't miss out on the headlines that shape our world!
Table of Contents
Debunking the Myth: Reinforcement Learning Doesn't Always Improve AI
Reinforcement learning (RL), a powerful machine learning technique, has garnered significant attention for its potential to revolutionize artificial intelligence. The idea is simple yet profound: train an AI agent through trial and error, rewarding desirable behaviors and punishing undesirable ones, leading to increasingly sophisticated performance. However, the reality is far more nuanced. While RL boasts impressive successes in games like Go and complex robotics, it's crucial to debunk the pervasive myth that RL always leads to improved AI performance. In fact, in many scenarios, it can be inefficient, unstable, or even detrimental.
<h3>The Allure and Limitations of Reinforcement Learning</h3>
The appeal of RL is undeniable. Its ability to learn complex behaviors without explicit programming holds immense promise for automating tasks that are difficult or impossible to codify using traditional methods. From optimizing traffic flow to developing advanced prosthetic control, RL's potential applications are vast. However, several limitations often hinder its effectiveness:
-
Data Efficiency: RL algorithms often require enormous amounts of data to converge on optimal solutions. This can be prohibitively expensive and time-consuming, especially in real-world scenarios where data acquisition is costly or dangerous. This is in stark contrast to supervised learning, which can often achieve good performance with smaller datasets.
-
Reward Shaping Challenges: Defining a suitable reward function is crucial for RL's success. A poorly designed reward function can lead the agent to exploit loopholes or develop unintended behaviors, ultimately undermining the desired outcome. This problem is particularly acute in complex environments where the consequences of actions are difficult to predict.
-
Sample Inefficiency and Instability: RL agents can be notoriously unstable, exhibiting significant performance fluctuations during training. This instability, combined with sample inefficiency, can make it challenging to guarantee consistent and reliable results. Many RL algorithms struggle with exploration-exploitation trade-offs, often getting stuck in local optima or failing to explore the full state space effectively.
-
Computational Cost: Training sophisticated RL agents often necessitates significant computational resources, making it inaccessible for many researchers and practitioners. This barrier to entry limits the widespread adoption and application of RL in various domains.
<h3>Where Reinforcement Learning Falls Short</h3>
While RL shines in controlled environments with well-defined reward functions, its application in real-world scenarios often faces significant challenges. Consider these examples:
-
Robotics: While RL has shown promise in robotic control, translating successes from simulated environments to real-world robotics remains a significant hurdle. The discrepancies between simulated and real-world physics can lead to unexpected failures.
-
Healthcare: Applying RL to personalize treatment plans requires careful consideration of ethical implications and the potential for unforeseen consequences. The high stakes involved demand robust safety mechanisms and thorough validation before deployment.
-
Finance: The volatility and complexity of financial markets make it challenging to apply RL effectively. The risk of catastrophic losses due to unexpected market fluctuations necessitates careful risk management strategies.
<h3>Beyond the Hype: A Balanced Perspective</h3>
Reinforcement learning is a powerful tool, but it's not a silver bullet. Its effectiveness depends heavily on careful problem formulation, appropriate algorithm selection, and meticulous design of the reward function. Instead of viewing RL as a universal solution, it's crucial to adopt a balanced perspective, acknowledging its limitations alongside its strengths. Future research should focus on developing more data-efficient, stable, and robust RL algorithms to overcome these challenges and unlock its full potential across diverse applications. The future of AI likely involves a hybrid approach, combining RL with other machine learning techniques to leverage their complementary strengths and mitigate their individual weaknesses. Only then can we fully harness the power of reinforcement learning while avoiding the pitfalls of overblown expectations.
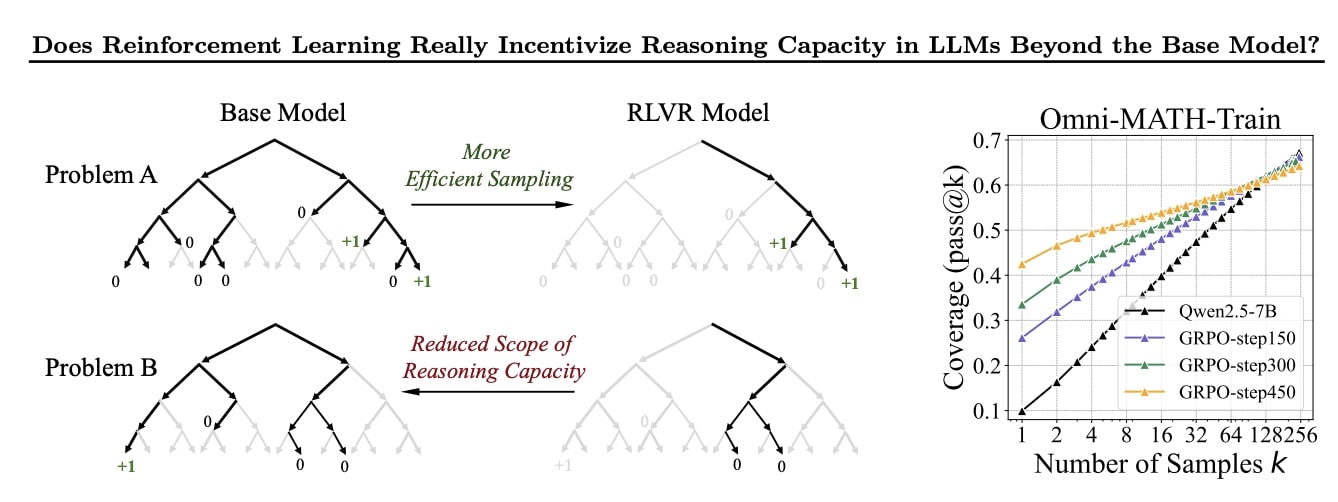
Thank you for visiting our website, your trusted source for the latest updates and in-depth coverage on Debunking The Myth: Reinforcement Learning Doesn't Always Improve AI. We're committed to keeping you informed with timely and accurate information to meet your curiosity and needs.
If you have any questions, suggestions, or feedback, we'd love to hear from you. Your insights are valuable to us and help us improve to serve you better. Feel free to reach out through our contact page.
Don't forget to bookmark our website and check back regularly for the latest headlines and trending topics. See you next time, and thank you for being part of our growing community!
Featured Posts
-
Very Special Player Pivotal In Trumps Narrow Victory Over Murphy
Apr 28, 2025 -
Van Dijks Our Dream Your Dream Message Resonates With Liverpool
Apr 28, 2025 -
Modular Smartphone Innovation Nothing Phone 2 Breaks The Mold
Apr 28, 2025 -
Discover The Hidden Gem A Gripping Morgan Freeman Thriller Streaming On Netflix
Apr 28, 2025 -
Is This The Solution To Americas Oversized Truck Problem
Apr 28, 2025
Latest Posts
-
Rider Beware Uber To De Platform Low Rated Users
Apr 30, 2025 -
Martian Cartographys Transformation Unveiling A New Red Planet
Apr 30, 2025 -
Ucl Semi Finals Arsenal Psg Barcelona And Inter Milan Face Off
Apr 30, 2025 -
Madden 26 And College Football 26 Release Date Announced Bundle Confirmed By Ea Sports
Apr 30, 2025 -
Dwayne The Rock Johnsons Oscar Campaign First Trailer For Black Adam Released
Apr 30, 2025