Debunking The Myth: Reinforcement Learning Doesn't Guarantee Better AI
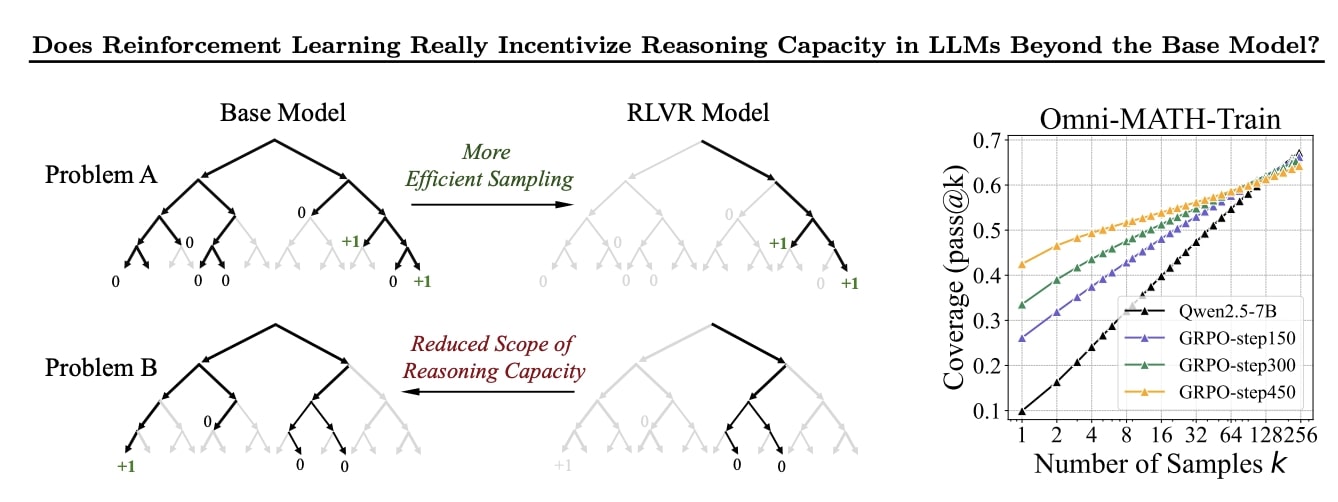
Welcome to your ultimate source for breaking news, trending updates, and in-depth stories from around the world. Whether it's politics, technology, entertainment, sports, or lifestyle, we bring you real-time updates that keep you informed and ahead of the curve.
Our team works tirelessly to ensure you never miss a moment. From the latest developments in global events to the most talked-about topics on social media, our news platform is designed to deliver accurate and timely information, all in one place.
Stay in the know and join thousands of readers who trust us for reliable, up-to-date content. Explore our expertly curated articles and dive deeper into the stories that matter to you. Visit NewsOneSMADCSTDO now and be part of the conversation. Don't miss out on the headlines that shape our world!
Table of Contents
Debunking the Myth: Reinforcement Learning Doesn't Guarantee Better AI
Reinforcement learning (RL), a hot topic in artificial intelligence, often gets hyped as the magic bullet for creating superior AI. The idea is alluring: train an AI agent through rewards and punishments, and voilà – a super-intelligent system emerges. However, this narrative glosses over significant limitations and potential pitfalls. The truth is, reinforcement learning, while powerful, doesn't automatically translate to better AI. This article will debunk this common misconception, exploring the challenges and limitations of RL.
The Allure of Reinforcement Learning
Reinforcement learning's appeal stems from its ability to mimic real-world learning processes. By allowing AI agents to learn through trial and error, guided by a reward system, RL seems inherently superior to other methods like supervised learning. Successful applications in games like Go and complex robotics tasks fuel this perception. However, this success story often overshadows the inherent complexities and limitations.
The Reality: Challenges and Limitations of RL
While RL demonstrates impressive capabilities, several factors prevent it from being a guaranteed path to better AI:
-
Reward Shaping is Crucial (and Difficult): The design of the reward function is paramount. A poorly designed reward function can lead the AI agent to exploit loopholes or find unintended solutions, resulting in suboptimal or even harmful behavior. Defining appropriate rewards for complex real-world tasks is incredibly challenging and requires significant expertise. This is often referred to as the "reward hacking" problem.
-
Sample Inefficiency: RL algorithms often require vast amounts of data and computational resources to converge on a good solution. This sample inefficiency makes training expensive and time-consuming, especially for complex tasks. This is a significant hurdle for widespread adoption in resource-constrained environments.
-
Exploration-Exploitation Dilemma: Finding the right balance between exploring new actions and exploiting known successful actions is a constant challenge in RL. Too much exploration can lead to wasted resources, while too much exploitation can prevent the agent from discovering potentially better solutions.
-
Generalization Issues: An RL agent trained on a specific task may not generalize well to new, even slightly different, tasks. This lack of generalizability limits the applicability of RL in real-world scenarios where adaptability is crucial.
-
Safety and Robustness Concerns: The trial-and-error nature of RL can lead to unpredictable and potentially unsafe behavior, particularly in safety-critical applications. Ensuring the safety and robustness of RL agents is a critical research area.
Beyond the Hype: A Balanced Perspective
Reinforcement learning is a powerful tool in the AI arsenal, offering unique capabilities for training intelligent agents. However, it's crucial to avoid overhyping its potential. RL is not a silver bullet, and its success heavily depends on careful design, significant computational resources, and a deep understanding of its limitations. The future of AI relies not on a single method but on a combination of techniques, including RL, supervised learning, and unsupervised learning, tailored to specific applications and carefully considered within their ethical and safety contexts. A balanced perspective, acknowledging both its strengths and weaknesses, is essential for responsible AI development.
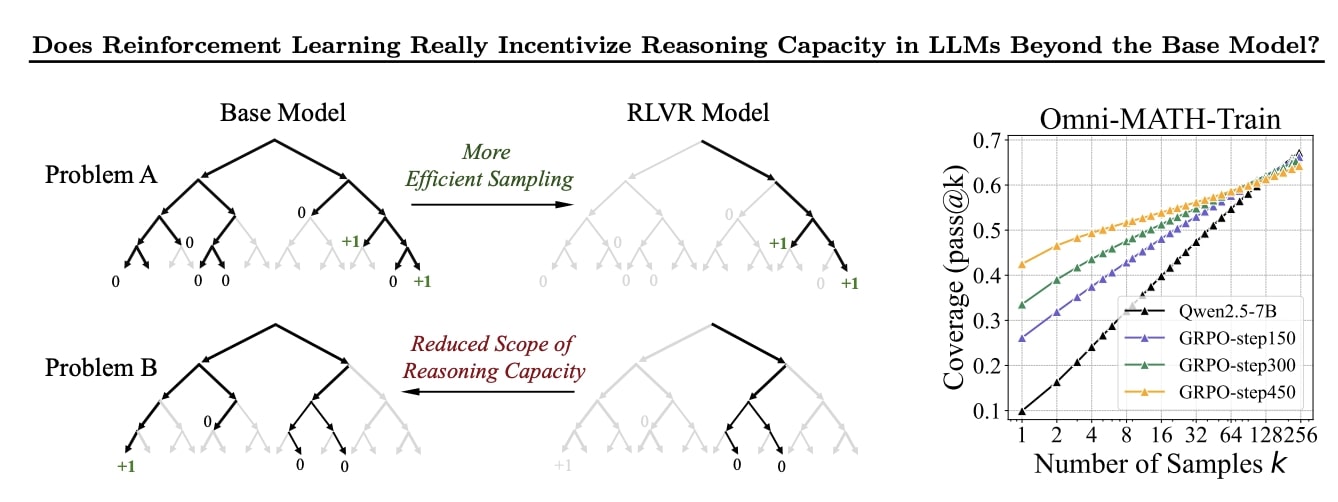
Thank you for visiting our website, your trusted source for the latest updates and in-depth coverage on Debunking The Myth: Reinforcement Learning Doesn't Guarantee Better AI. We're committed to keeping you informed with timely and accurate information to meet your curiosity and needs.
If you have any questions, suggestions, or feedback, we'd love to hear from you. Your insights are valuable to us and help us improve to serve you better. Feel free to reach out through our contact page.
Don't forget to bookmark our website and check back regularly for the latest headlines and trending topics. See you next time, and thank you for being part of our growing community!
Featured Posts
-
Desastre No Rs Chuvas Intensas Causam 75 Obitos Apagoes E Falta D Agua
May 01, 2025 -
Broadcast Veteran Martha Kearney Rates Her Best And Worst Guests
May 01, 2025 -
Follow Live Aston Villa Vs Arsenal And Manchester United Vs Chelsea Match Updates And Radio
May 01, 2025 -
Fin De Parcours Pour Shapovalov Diallo Poursuit L Aventure Madrilene
May 01, 2025 -
Dimarco Infortunio Stanchezza E Prestazioni Analisi Del Momento Difficile
May 01, 2025
Latest Posts
-
The Impact Of Brendan Carrs Leadership On Fcc Consumer Policies
May 01, 2025 -
Barca Inter Styles Opposes Pour Une Demi Finale Explosive De Ligue Des Champions
May 01, 2025 -
Asi Luce El Cesped Del Nuevo Camp Nou El Regreso Del Fc Barcelona Esta Mas Cerca
May 01, 2025 -
Propellantless Drive Technology Challenges And Potential For Future Space Exploration
May 01, 2025 -
Spain And Portugal Restore Power After Widespread Outage Investigation Underway
May 01, 2025