Debunking The Myth: Reinforcement Learning Doesn't Revolutionize AI
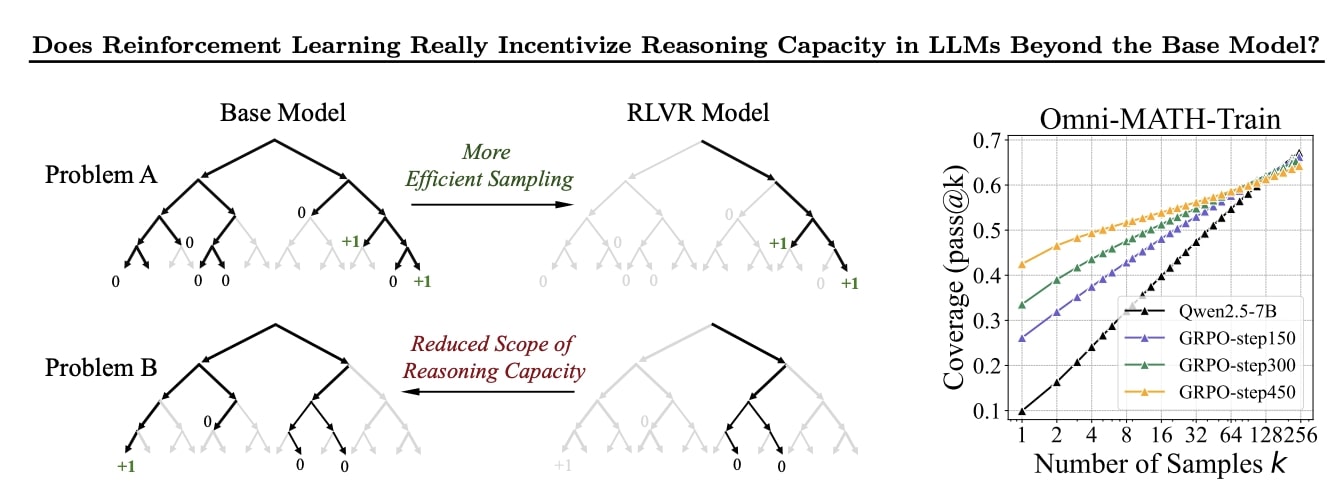
Welcome to your ultimate source for breaking news, trending updates, and in-depth stories from around the world. Whether it's politics, technology, entertainment, sports, or lifestyle, we bring you real-time updates that keep you informed and ahead of the curve.
Our team works tirelessly to ensure you never miss a moment. From the latest developments in global events to the most talked-about topics on social media, our news platform is designed to deliver accurate and timely information, all in one place.
Stay in the know and join thousands of readers who trust us for reliable, up-to-date content. Explore our expertly curated articles and dive deeper into the stories that matter to you. Visit NewsOneSMADCSTDO now and be part of the conversation. Don't miss out on the headlines that shape our world!
Table of Contents
Debunking the Myth: Reinforcement Learning Doesn't Revolutionize AI – Yet
Reinforcement learning (RL) has captured the imagination of the tech world, promising a revolution in artificial intelligence. Images of self-driving cars navigating complex roads and AI agents mastering intricate games fuel this hype. However, the reality is more nuanced. While RL is a powerful technique with significant applications, it's crucial to debunk the myth that it single-handedly revolutionizes AI. This article explores the limitations and overhyped aspects of RL, providing a balanced perspective on its current capabilities and future potential.
The Allure of Reinforcement Learning
Reinforcement learning, a subfield of machine learning, focuses on training agents to make decisions in an environment by rewarding desired behaviors and penalizing undesirable ones. This trial-and-error approach mimics how humans learn, leading to its widespread appeal. Successful applications in areas like game playing (AlphaGo famously beating a Go champion) and robotics have further solidified its reputation as a transformative technology.
Limitations and Challenges of Reinforcement Learning
Despite its successes, RL faces significant challenges that prevent it from being the AI revolution many anticipate:
-
Data Dependency: RL algorithms require vast amounts of data for training, often gathered through extensive simulations or real-world interactions. This data collection process can be expensive, time-consuming, and sometimes impossible to achieve in complex real-world scenarios.
-
Sample Inefficiency: RL agents can be incredibly inefficient in learning. They often require millions, or even billions, of trials before achieving satisfactory performance. This makes training computationally expensive and practically infeasible for many applications.
-
Reward Shaping Challenges: Defining appropriate reward functions is crucial for RL's success. Poorly designed reward functions can lead to unintended and undesirable behavior, a problem known as reward hacking. Creating effective reward functions for complex tasks remains a significant hurdle.
-
Generalization Issues: RL agents often struggle to generalize their learned knowledge to new or unseen situations. A robot trained to navigate a specific warehouse might fail to adapt to a slightly different environment, highlighting the limitations of their adaptability.
-
Safety and Explainability: The "black box" nature of many RL algorithms makes it difficult to understand their decision-making processes. This lack of transparency poses significant safety concerns, especially in critical applications like autonomous driving or medical diagnosis.
Where RL Excels and Where it Falls Short
Reinforcement learning is undeniably a powerful tool, excelling in specific areas such as:
- Game playing: RL has demonstrated remarkable success in mastering complex games, showcasing its ability to learn optimal strategies.
- Robotics control: RL algorithms can be used to train robots to perform intricate tasks, such as manipulating objects or navigating challenging environments.
- Personalized recommendations: RL can be applied to optimize personalized recommendations in areas like e-commerce and entertainment.
However, claiming RL as a complete AI revolution is premature. Its limitations in data efficiency, generalization, and safety significantly restrict its broader applicability. Many real-world problems remain intractable for current RL techniques.
The Future of Reinforcement Learning
While RL doesn't represent a complete AI revolution today, its potential remains significant. Ongoing research focuses on:
- Improved sample efficiency: Researchers are actively developing algorithms that require less data for training.
- Safe RL: Methods to ensure the safety and reliability of RL agents are being developed and improved.
- Transfer learning: Techniques to enable RL agents to transfer knowledge learned in one environment to another are being explored.
These advancements will gradually expand RL's capabilities and potentially lead to more widespread adoption in diverse fields. However, it's important to maintain a realistic perspective – the AI revolution is a marathon, not a sprint, and RL is just one crucial piece of the puzzle. Its role is significant, but it's not the sole driver of AI's advancement.
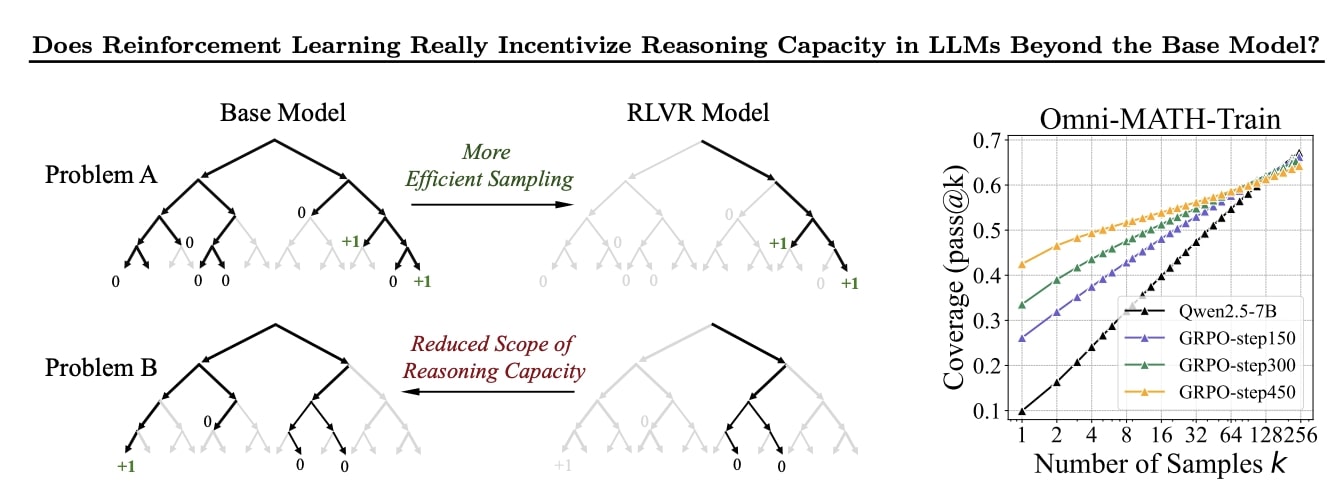
Thank you for visiting our website, your trusted source for the latest updates and in-depth coverage on Debunking The Myth: Reinforcement Learning Doesn't Revolutionize AI. We're committed to keeping you informed with timely and accurate information to meet your curiosity and needs.
If you have any questions, suggestions, or feedback, we'd love to hear from you. Your insights are valuable to us and help us improve to serve you better. Feel free to reach out through our contact page.
Don't forget to bookmark our website and check back regularly for the latest headlines and trending topics. See you next time, and thank you for being part of our growing community!
Featured Posts
-
Elon Musks Grok Ai Finds A Home Microsofts Cloud To Power Next Gen Ai
May 03, 2025 -
Baseball Picks Today 5 2 Fernando Tatis Jr S Performance Projections
May 03, 2025 -
Lapu Lapu Day Attack Vancouver Teen Speaks Out About Familys Loss
May 03, 2025 -
Lorde Credits Charli Xcx For Virgin Album The Power Of Collaboration
May 03, 2025 -
Who Is The Spokesperson For Clive Palmers High Profile Advertising Campaign
May 03, 2025
Latest Posts
-
Watch Caitlin Clark Live Indiana Fever Vs Washington Mystics Tv Schedule And Start Time
May 03, 2025 -
Nea And Neh Funding Trump Administrations Proposed Cuts
May 03, 2025 -
Prime Video Expands Shop The Show A Boon For Business Or Bane For Storytelling
May 03, 2025 -
Long Road Ahead Padres Journey Begins In Pittsburgh
May 03, 2025 -
Ge 2025 What To Expect From Singapores 14th General Election
May 03, 2025