Debunking The Myth: Reinforcement Learning's Impact On AI Is Overstated
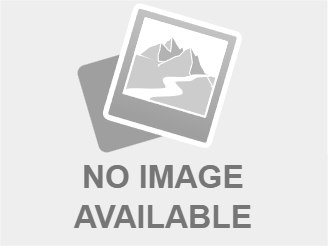
Welcome to your ultimate source for breaking news, trending updates, and in-depth stories from around the world. Whether it's politics, technology, entertainment, sports, or lifestyle, we bring you real-time updates that keep you informed and ahead of the curve.
Our team works tirelessly to ensure you never miss a moment. From the latest developments in global events to the most talked-about topics on social media, our news platform is designed to deliver accurate and timely information, all in one place.
Stay in the know and join thousands of readers who trust us for reliable, up-to-date content. Explore our expertly curated articles and dive deeper into the stories that matter to you. Visit NewsOneSMADCSTDO now and be part of the conversation. Don't miss out on the headlines that shape our world!
Table of Contents
Debunking the Myth: Reinforcement Learning's Impact on AI is Overstated
The field of Artificial Intelligence (AI) is constantly evolving, with new breakthroughs and advancements capturing headlines. Reinforcement learning (RL), a powerful technique enabling AI agents to learn through trial and error, has been particularly hyped as a revolutionary force poised to reshape everything from self-driving cars to personalized medicine. However, a closer look reveals a more nuanced reality: the impact of reinforcement learning on AI, while significant, is often overstated. This article will dissect the hype and explore the limitations of RL, highlighting where its impact is genuinely transformative and where expectations need recalibration.
The Allure of Reinforcement Learning: Why the Hype?
Reinforcement learning's appeal lies in its ability to train AI agents to master complex tasks without explicit programming. By rewarding desired behaviors and penalizing undesired ones, RL algorithms can achieve impressive results, often surpassing human performance in specific domains like game playing (e.g., AlphaGo). This success has fueled the narrative of RL as a universal solution for AI challenges. Keywords such as AI breakthroughs, machine learning, deep reinforcement learning, and artificial intelligence advancements frequently accompany discussions of RL's potential.
However, this narrative overlooks crucial limitations.
The Reality Check: Limitations of Reinforcement Learning
While undeniably powerful, reinforcement learning suffers from several significant drawbacks:
-
Data Hunger: RL algorithms require massive amounts of data to learn effectively. This can be computationally expensive and time-consuming, particularly in real-world applications where data acquisition is often challenging and costly. The need for big data in RL is a considerable hurdle.
-
Sample Inefficiency: RL agents often learn slowly, requiring numerous iterations before achieving satisfactory performance. This inefficiency can make RL impractical for tasks with high stakes or limited opportunities for experimentation. Improving sample efficiency is a major area of ongoing research.
-
Safety and Reliability: The trial-and-error nature of RL can lead to unpredictable and potentially dangerous behavior, especially in safety-critical applications like autonomous driving. Ensuring the safety and reliability of RL agents is paramount but remains a significant challenge.
-
Interpretability and Explainability: Understanding why an RL agent makes a particular decision can be incredibly difficult. This lack of interpretability and explainability limits its use in domains requiring transparency and accountability, such as healthcare and finance.
-
Reward Engineering: Defining appropriate reward functions is crucial for RL's success. Poorly designed reward functions can lead to unexpected and undesirable outcomes, a phenomenon known as reward hacking. This highlights the importance of careful reward engineering.
Where RL Truly Shines: Specific Applications
Despite its limitations, reinforcement learning excels in specific areas:
-
Robotics: RL is proving invaluable in training robots to perform complex manipulation tasks and navigate challenging environments. Robotics applications are a strong suit for RL.
-
Game Playing: As demonstrated by AlphaGo and other examples, RL has achieved superhuman performance in various games.
-
Resource Optimization: RL can be used to optimize resource allocation in various settings, such as traffic management and energy grids. Resource optimization is a promising area for RL deployment.
Conclusion: A Balanced Perspective on Reinforcement Learning's Impact
Reinforcement learning is a powerful tool with the potential to revolutionize aspects of AI. However, the hype surrounding its capabilities often overshadows its limitations. A more balanced and realistic perspective is crucial. While RL shows promise in specific domains, it's not a universal solution for all AI problems. Focusing research on addressing its limitations – particularly sample efficiency, safety, and interpretability – will be key to unlocking its full potential and ensuring its responsible deployment across diverse applications. Only then can we fully appreciate the genuine – and significant – impact of reinforcement learning on the future of artificial intelligence.
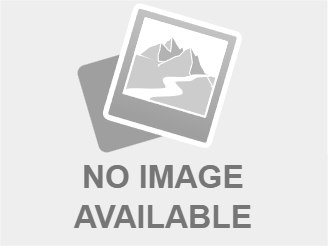
Thank you for visiting our website, your trusted source for the latest updates and in-depth coverage on Debunking The Myth: Reinforcement Learning's Impact On AI Is Overstated. We're committed to keeping you informed with timely and accurate information to meet your curiosity and needs.
If you have any questions, suggestions, or feedback, we'd love to hear from you. Your insights are valuable to us and help us improve to serve you better. Feel free to reach out through our contact page.
Don't forget to bookmark our website and check back regularly for the latest headlines and trending topics. See you next time, and thank you for being part of our growing community!
Featured Posts
-
Madrid Tennis Ruuds Victory Over Fritz Highlights Days Play Sabalenka And Medvedev Progress
May 01, 2025 -
Derby D Italia Giudizi Sulle Prestazioni Angelino Vs Dimarco
May 01, 2025 -
Yamal Rejects Messi Comparisons Barcelona Prodigy Charts His Own Course
May 01, 2025 -
Grigor Dimitrov Wins Against Fearnley In Madrid
May 01, 2025 -
Investimento Em Risco Criptomoeda De Rede Social Sofre Queda Estupenda De 98
May 01, 2025
Latest Posts
-
Slow Road To Recovery Analyzing The Mantra Om Price After Aprils Dip
May 01, 2025 -
Coalitions Nuclear Energy Push Progress And Challenges Towards A 2030s Target
May 01, 2025 -
Manchester Uniteds Resolve Mounts Assurance Of Unending Fight
May 01, 2025 -
Micro Strategy Mstr Stock Analyst Predicts Q1 Earnings Boost
May 01, 2025 -
Two Senior Man United Executives To Exit In Latest Round Of Layoffs
May 01, 2025