Debunking The Myth: Reinforcement Learning's Impact On AI Model Improvement
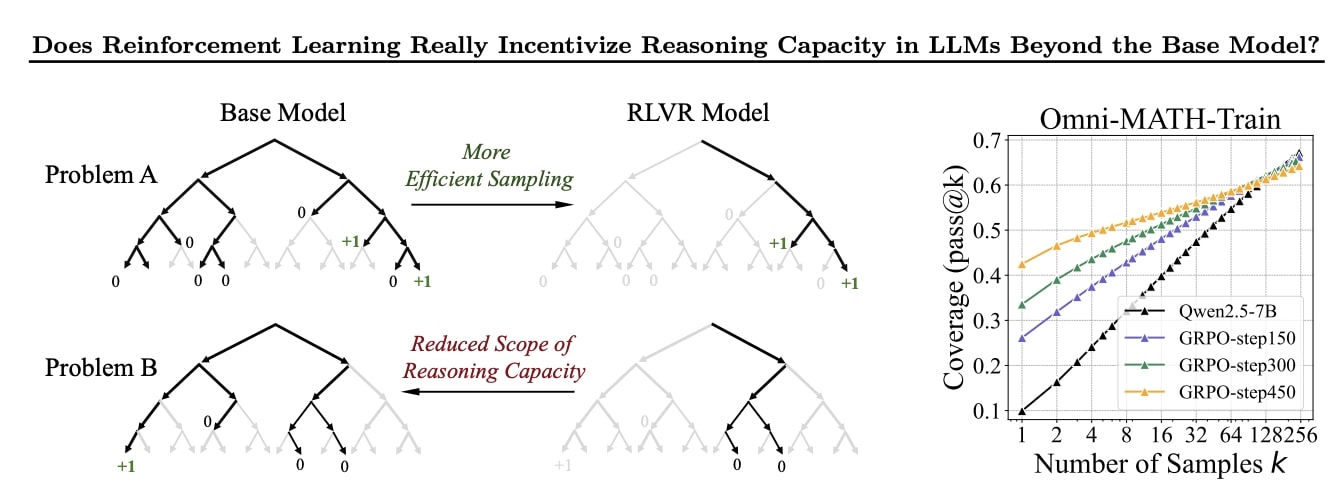
Welcome to your ultimate source for breaking news, trending updates, and in-depth stories from around the world. Whether it's politics, technology, entertainment, sports, or lifestyle, we bring you real-time updates that keep you informed and ahead of the curve.
Our team works tirelessly to ensure you never miss a moment. From the latest developments in global events to the most talked-about topics on social media, our news platform is designed to deliver accurate and timely information, all in one place.
Stay in the know and join thousands of readers who trust us for reliable, up-to-date content. Explore our expertly curated articles and dive deeper into the stories that matter to you. Visit NewsOneSMADCSTDO now and be part of the conversation. Don't miss out on the headlines that shape our world!
Table of Contents
Debunking the Myth: Reinforcement Learning's Impact on AI Model Improvement
Reinforcement learning (RL) has emerged as a powerful technique in artificial intelligence, promising significant advancements in AI model improvement. However, misconceptions persist about its capabilities and limitations. This article debunks common myths surrounding RL's impact on AI model enhancement, providing a clear and concise understanding of its true potential and challenges.
The Rise of Reinforcement Learning in AI
Reinforcement learning algorithms, inspired by behavioral psychology, enable AI agents to learn optimal strategies through trial and error. Unlike supervised learning, which relies on labeled data, RL agents learn by interacting with an environment, receiving rewards for desirable actions and penalties for undesirable ones. This iterative process allows the agent to refine its behavior over time, ultimately achieving a desired goal. This makes RL particularly suitable for complex tasks where explicit programming is difficult, such as game playing, robotics, and resource management.
Myth 1: RL is a Silver Bullet for All AI Problems
A common misconception is that RL can magically solve all AI model improvement challenges. While RL offers substantial advantages, it's not a universal solution. Its effectiveness depends heavily on several factors:
- Reward Function Design: Defining a precise and accurate reward function is crucial. A poorly designed reward function can lead the agent to exploit loopholes or achieve unintended outcomes. This is a significant hurdle in many real-world applications.
- Exploration-Exploitation Dilemma: Balancing exploration (trying new actions) and exploitation (using known good actions) is essential. Too much exploration can lead to slow learning, while too much exploitation can prevent the discovery of better strategies.
- Sample Efficiency: RL algorithms often require a large number of interactions with the environment to learn effectively. This can be computationally expensive and time-consuming, particularly in complex scenarios.
- Generalization: An agent trained in one environment may not generalize well to another, limiting its applicability to diverse real-world situations.
Myth 2: RL Always Leads to Superior Performance
While RL can significantly improve AI model performance in specific tasks, it doesn't automatically guarantee superior results compared to other machine learning methods. The performance gains depend heavily on the specific problem, the quality of the RL algorithm, and the design of the reward function. In some cases, supervised or unsupervised learning techniques may be more efficient and effective.
Myth 3: Implementing RL is Simple and Straightforward
Deploying RL in real-world applications is far from trivial. It demands specialized expertise in algorithm design, reward function engineering, and hyperparameter tuning. The computational resources required can also be substantial, particularly for large-scale problems.
The Real Impact of Reinforcement Learning:
Despite the myths, reinforcement learning offers significant contributions to AI model improvement:
- Adaptability: RL agents can adapt to changing environments and learn to perform effectively in dynamic situations.
- Automation: RL can automate complex decision-making processes, reducing the need for human intervention.
- Optimization: RL algorithms can find optimal solutions to complex problems, often surpassing human performance.
Conclusion:
Reinforcement learning is a powerful tool in the AI arsenal, but its effectiveness depends on careful consideration of its limitations. By understanding the challenges and potential pitfalls associated with RL, researchers and developers can harness its true power to enhance AI models and drive innovation across various fields. The future of AI hinges on a balanced understanding and application of RL, moving beyond the myths and focusing on its genuine potential for improving AI performance and capabilities.
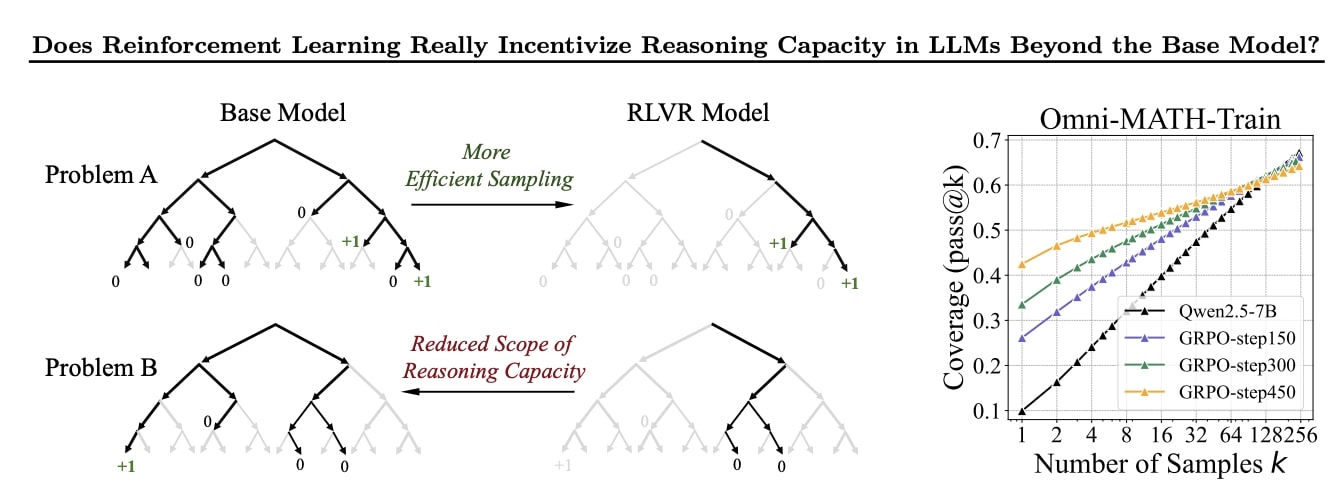
Thank you for visiting our website, your trusted source for the latest updates and in-depth coverage on Debunking The Myth: Reinforcement Learning's Impact On AI Model Improvement. We're committed to keeping you informed with timely and accurate information to meet your curiosity and needs.
If you have any questions, suggestions, or feedback, we'd love to hear from you. Your insights are valuable to us and help us improve to serve you better. Feel free to reach out through our contact page.
Don't forget to bookmark our website and check back regularly for the latest headlines and trending topics. See you next time, and thank you for being part of our growing community!
Featured Posts
-
Otp 2 Significant Slowdown Observed In Propellantless Satellites Orbital Decay
Apr 30, 2025 -
First Round Domination The Rocks Upcoming Role As The Smashing Machine
Apr 30, 2025 -
Arsenal Psg Champions League Clash Live Score And Highlights From The First Leg
Apr 30, 2025 -
Impacto Das Chuvas No Rs 75 Mortes 839 Mil Casas Sem Agua E 420 Mil Sem Energia Eletrica
Apr 30, 2025 -
Arsenal Psg En Direct Doue Et Dembele Titulaires Pour La Demi Finale Aller
Apr 30, 2025
Latest Posts
-
Effective I Phone Screen Time Management Practical Steps For A Healthier Digital Life
Apr 30, 2025 -
Manchester United Women Vs Chelsea Key Players Prediction And Where To Watch
Apr 30, 2025 -
Live Uefa Champions League Barcelona Vs Inter Milan Key Moments And Analysis
Apr 30, 2025 -
Kawasakis Unlikely Path To Victory Confronting Al Nassrs Star Power
Apr 30, 2025 -
Which Dyson Fan Should I Buy A Comprehensive Guide To Dyson Fan Models
Apr 30, 2025