Debunking The Myth: Reinforcement Learning's Impact On AI Model Performance
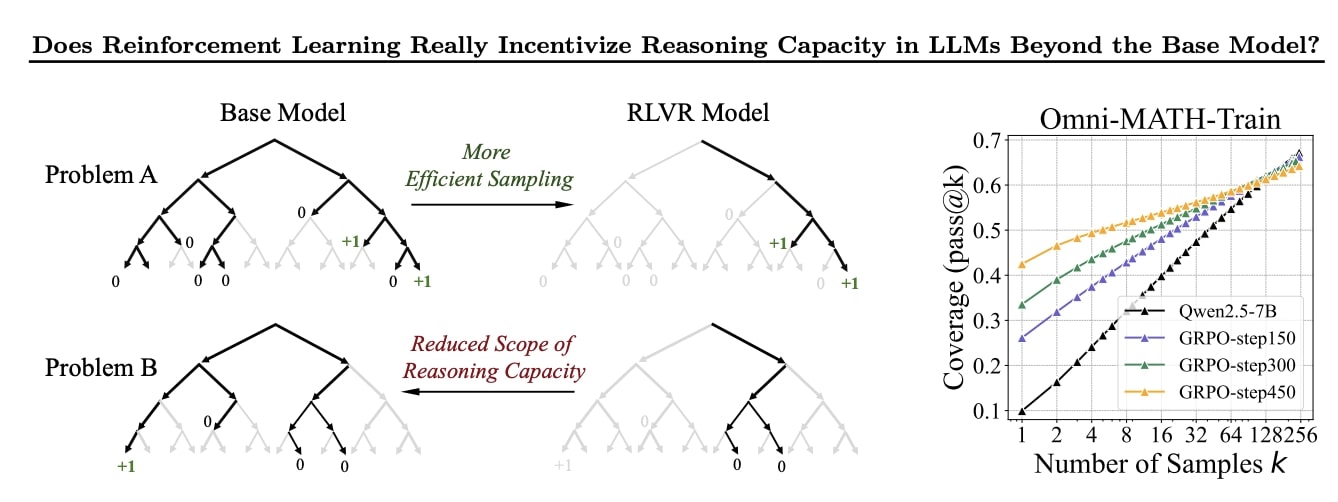
Welcome to your ultimate source for breaking news, trending updates, and in-depth stories from around the world. Whether it's politics, technology, entertainment, sports, or lifestyle, we bring you real-time updates that keep you informed and ahead of the curve.
Our team works tirelessly to ensure you never miss a moment. From the latest developments in global events to the most talked-about topics on social media, our news platform is designed to deliver accurate and timely information, all in one place.
Stay in the know and join thousands of readers who trust us for reliable, up-to-date content. Explore our expertly curated articles and dive deeper into the stories that matter to you. Visit NewsOneSMADCSTDO now and be part of the conversation. Don't miss out on the headlines that shape our world!
Table of Contents
Debunking the Myth: Reinforcement Learning's Impact on AI Model Performance
Reinforcement learning (RL) has emerged as a powerful technique in artificial intelligence, promising significant improvements in AI model performance. However, the hype surrounding RL sometimes overshadows a nuanced understanding of its actual impact. This article delves into the realities of RL's contribution, debunking common myths and exploring its true potential and limitations.
The Allure of Reinforcement Learning:
RL's appeal lies in its ability to train agents to achieve complex goals through trial and error. Unlike supervised learning, which relies on labeled datasets, RL agents learn by interacting with an environment, receiving rewards for desirable actions and penalties for undesirable ones. This adaptive learning process seems inherently superior, leading to the misconception that RL automatically translates to superior AI model performance.
Myth 1: RL Always Outperforms Supervised Learning:
This is demonstrably false. While RL excels in scenarios requiring adaptability and decision-making in dynamic environments (like game playing or robotics), it's not a universal panacea. Supervised learning often proves more efficient and accurate for tasks with readily available labeled data and clear objective functions. The choice between RL and supervised learning depends heavily on the specific problem and available resources. Choosing the right approach is crucial for optimal results.
Myth 2: RL Requires Minimal Data:
Another misconception is that RL requires less data than supervised learning. In reality, training robust RL agents often demands substantial interaction with the environment, which can translate into a significant data collection burden. Furthermore, effectively designing reward functions and managing exploration-exploitation trade-offs are complex tasks requiring expertise and iterative refinement. The "data efficiency" of RL is often overstated.
Myth 3: RL Models are Always Robust and Generalizable:
RL models, like any AI model, are susceptible to overfitting and poor generalization. An agent trained to excel in a specific simulated environment might perform poorly when deployed in the real world due to discrepancies between the simulated and real-world conditions. Robustness and generalization require careful design and rigorous testing.
The Reality of Reinforcement Learning's Impact:
Reinforcement learning's impact on AI model performance is significant, but it's not a magic bullet. Its true value lies in its ability to:
- Solve complex sequential decision-making problems: RL shines in scenarios where actions have long-term consequences, requiring strategic planning and adaptation.
- Automate tasks requiring continuous adaptation: In dynamic environments, RL agents can learn and adjust their behavior effectively.
- Improve performance in areas where human expertise is limited: RL can provide solutions in domains where explicit programming is difficult or impossible.
Conclusion: A Balanced Perspective
Reinforcement learning offers exciting possibilities for enhancing AI model performance, but its application requires careful consideration. Understanding its strengths and limitations is crucial for successfully integrating RL into AI systems. Rather than viewing RL as a superior alternative to other machine learning approaches, it's more accurate to see it as a powerful tool in the AI arsenal, best utilized when its unique capabilities align with the specific problem being addressed. Focusing on responsible development and deployment will unlock RL's true potential and avoid falling prey to inflated expectations.
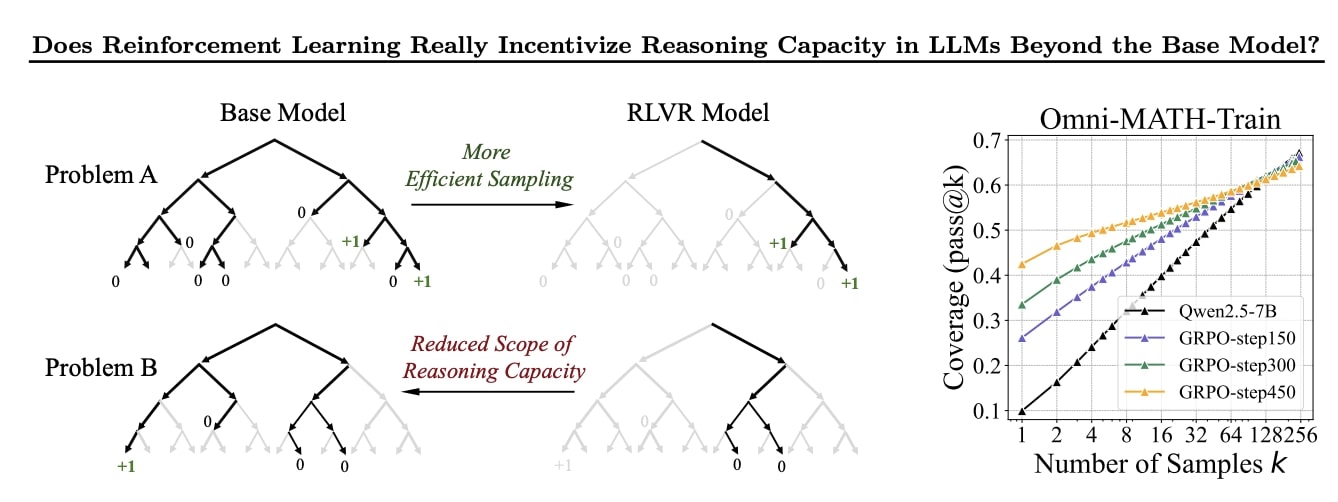
Thank you for visiting our website, your trusted source for the latest updates and in-depth coverage on Debunking The Myth: Reinforcement Learning's Impact On AI Model Performance. We're committed to keeping you informed with timely and accurate information to meet your curiosity and needs.
If you have any questions, suggestions, or feedback, we'd love to hear from you. Your insights are valuable to us and help us improve to serve you better. Feel free to reach out through our contact page.
Don't forget to bookmark our website and check back regularly for the latest headlines and trending topics. See you next time, and thank you for being part of our growing community!
Featured Posts
-
Injury Blow For Fiorentina Cataldis Absence Could Decide Conference League Fate
May 02, 2025 -
Lauren James Impact How Chelsea Conquered The Wsl Under New Management
May 02, 2025 -
Sons Heartfelt Remembrance Of Mother Killed In Vancouver Festival Attack
May 02, 2025 -
Agora Assista Ao Vivo A Reuniao Anual Da Berkshire Hathaway 2024 Via Info Money
May 02, 2025 -
The Royal Familys 2025 Curveball Prince Harrys Latest Action
May 02, 2025
Latest Posts
-
Ge 2025 Dpm Gans Punggol Shift A Strategic Decision Or Sign Of Diminished Influence Pritams Analysis
May 03, 2025 -
The Movie Title Sequel A Disappointing Return
May 03, 2025 -
May Showers Likely Met Service Forecasts Thundery Downpours
May 03, 2025 -
Sui Token Price Prediction Experts See Potential For 4
May 03, 2025 -
The Urgent Need For Crypto Tax Reform Beyond The 2014 Framework
May 03, 2025