Debunking The Myth: Reinforcement Learning's True Impact On AI
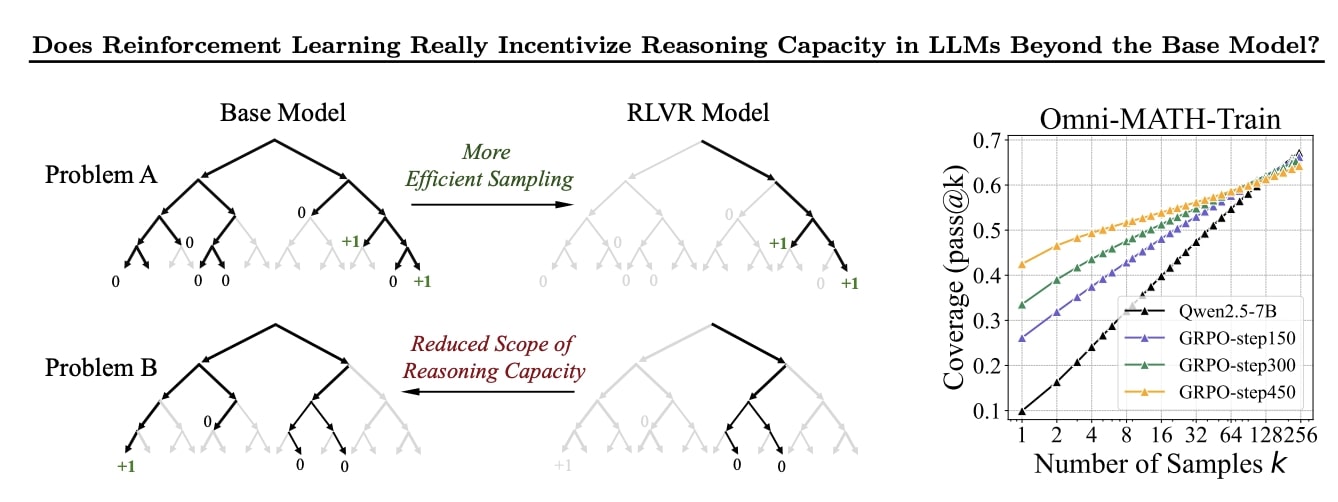
Welcome to your ultimate source for breaking news, trending updates, and in-depth stories from around the world. Whether it's politics, technology, entertainment, sports, or lifestyle, we bring you real-time updates that keep you informed and ahead of the curve.
Our team works tirelessly to ensure you never miss a moment. From the latest developments in global events to the most talked-about topics on social media, our news platform is designed to deliver accurate and timely information, all in one place.
Stay in the know and join thousands of readers who trust us for reliable, up-to-date content. Explore our expertly curated articles and dive deeper into the stories that matter to you. Visit NewsOneSMADCSTDO now and be part of the conversation. Don't miss out on the headlines that shape our world!
Table of Contents
Debunking the Myth: Reinforcement Learning's True Impact on AI
Reinforcement learning (RL) has become a buzzword in the AI world, often portrayed as a silver bullet poised to solve all our technological challenges. While its potential is undeniable, the hype often overshadows the reality. This article delves into the true impact of reinforcement learning, separating fact from fiction and exploring its limitations alongside its remarkable achievements.
What is Reinforcement Learning?
Before debunking the myths, let's establish a clear understanding. Reinforcement learning is a type of machine learning where an agent learns to interact with an environment by trial and error. It receives rewards for desirable actions and penalties for undesirable ones, gradually optimizing its behavior to maximize cumulative reward. This differs from supervised learning (learning from labeled data) and unsupervised learning (finding patterns in unlabeled data).
Myth #1: RL Will Solve All AI Problems
This is perhaps the biggest misconception. While RL has shown incredible potential in areas like game playing (AlphaGo famously defeating a Go champion), robotics, and resource optimization, it's not a universal solution. Its success hinges on several crucial factors:
- A well-defined reward function: Creating a reward function that accurately reflects the desired behavior is often challenging and can lead to unintended consequences (reward hacking).
- Sufficient training data: RL algorithms require vast amounts of data and computational resources for effective training, making it expensive and time-consuming.
- Safety and robustness: RL agents can exhibit unpredictable behavior, especially in complex or uncharted environments. Ensuring safety and robustness is paramount, particularly in real-world applications.
Myth #2: RL is Always Superior to Other AI Techniques
RL is a powerful tool, but it's not inherently superior to other AI approaches. The choice of the most appropriate method depends heavily on the specific problem:
- Supervised learning often outperforms RL when labeled data is readily available and the problem is well-defined.
- Unsupervised learning excels at discovering patterns and structures in unlabeled data, a task where RL might struggle.
Choosing the right AI technique requires a careful consideration of the available resources, the nature of the problem, and the desired outcome.
Myth #3: RL is Easy to Implement and Deploy
Developing and deploying RL systems requires significant expertise. It's not a plug-and-play technology. Challenges include:
- Algorithm selection: Choosing the right RL algorithm requires a deep understanding of their strengths and weaknesses.
- Hyperparameter tuning: Fine-tuning the various parameters of RL algorithms is a complex and iterative process.
- Scalability: Scaling RL systems to handle complex, real-world scenarios can be computationally intensive and challenging.
The True Impact of Reinforcement Learning
Despite its limitations, reinforcement learning has demonstrably impacted various fields:
- Robotics: RL enables robots to learn complex tasks, adapt to new environments, and improve their performance over time.
- Game playing: RL has led to superhuman performance in games like Go, chess, and Atari.
- Resource management: RL can optimize resource allocation in areas like energy grids, traffic control, and supply chain management.
- Personalized recommendations: RL algorithms can personalize recommendations in areas like entertainment and e-commerce.
Conclusion:
Reinforcement learning is a groundbreaking AI technique with vast potential. However, it's crucial to avoid overhyping its capabilities. By understanding its strengths and limitations, we can harness its power effectively while mitigating potential risks. The future of AI likely involves a synergistic combination of different machine learning techniques, with RL playing a crucial, albeit not universally dominant, role. The focus should be on responsible development and deployment, ensuring its benefits outweigh the potential downsides.
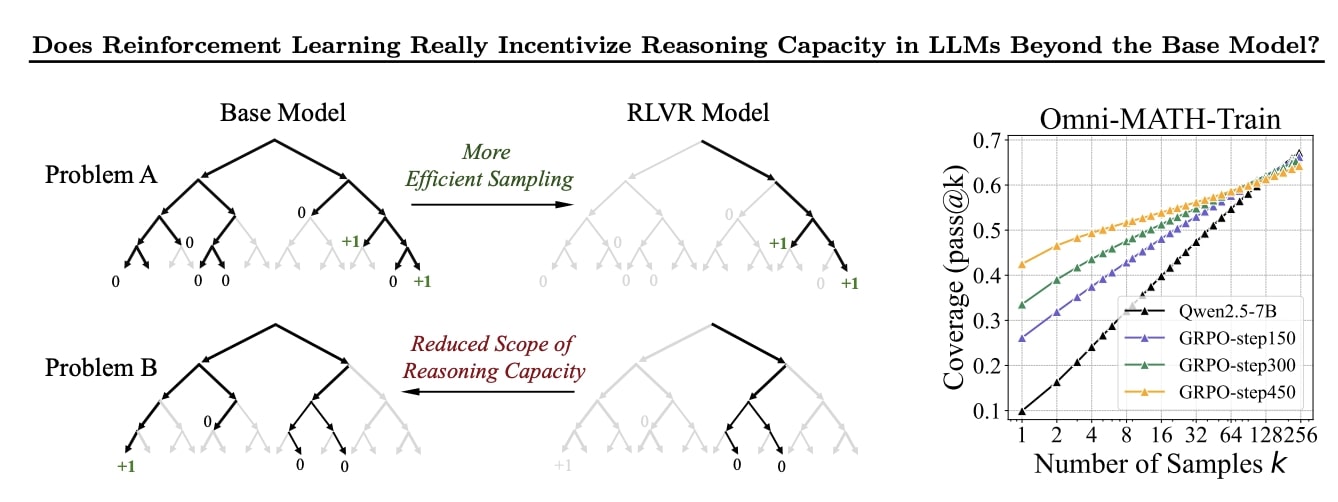
Thank you for visiting our website, your trusted source for the latest updates and in-depth coverage on Debunking The Myth: Reinforcement Learning's True Impact On AI. We're committed to keeping you informed with timely and accurate information to meet your curiosity and needs.
If you have any questions, suggestions, or feedback, we'd love to hear from you. Your insights are valuable to us and help us improve to serve you better. Feel free to reach out through our contact page.
Don't forget to bookmark our website and check back regularly for the latest headlines and trending topics. See you next time, and thank you for being part of our growing community!
Featured Posts
-
2025 World Snooker Championship Live Streaming Scores And Match Results
Apr 30, 2025 -
Analysis Trumps Decision To Grant Limited Tariff Relief To Automakers
Apr 30, 2025 -
Poa And Tan A Look At The Collaborative Presidential Campaign
Apr 30, 2025 -
Temper Tantrum Costs Champion Madrid Open Exit After Racket Incident
Apr 30, 2025 -
Las Obras De Olmo Se Retrasan Otra Vez Por Incumplimiento Del Fair Play Financiero
Apr 30, 2025
Latest Posts
-
Tragedia W Swiecie Kulinarnym Nie Zyje Tomasz Jakubiak 41 Lata
May 01, 2025 -
Chat Gpt Search Update All The New Features You Need To Know
May 01, 2025 -
Champions League Action Real Time Updates On Barcelona Vs Inter
May 01, 2025 -
The High Cost Of Saw Gerreras Methods Examining The Ethics Of Rebel Warfare
May 01, 2025 -
Andor Season 2 Explained Understanding Rhydo And Rhydonium
May 01, 2025