Does Reinforcement Learning Truly Advance AI? A Data-Driven Perspective
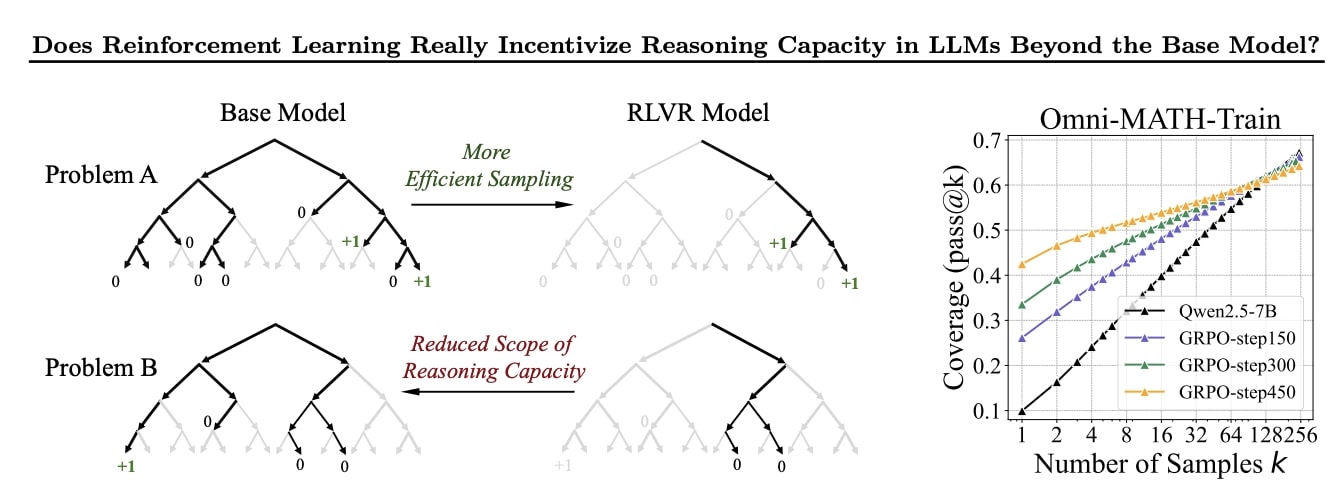
Welcome to your ultimate source for breaking news, trending updates, and in-depth stories from around the world. Whether it's politics, technology, entertainment, sports, or lifestyle, we bring you real-time updates that keep you informed and ahead of the curve.
Our team works tirelessly to ensure you never miss a moment. From the latest developments in global events to the most talked-about topics on social media, our news platform is designed to deliver accurate and timely information, all in one place.
Stay in the know and join thousands of readers who trust us for reliable, up-to-date content. Explore our expertly curated articles and dive deeper into the stories that matter to you. Visit NewsOneSMADCSTDO now and be part of the conversation. Don't miss out on the headlines that shape our world!
Table of Contents
Does Reinforcement Learning Truly Advance AI? A Data-Driven Perspective
Reinforcement learning (RL), a subfield of machine learning, has garnered significant attention for its potential to revolutionize artificial intelligence. But does it live up to the hype? This data-driven analysis explores the advancements and limitations of RL in propelling AI forward. While RL has undeniably achieved impressive feats, a balanced perspective reveals both its triumphs and ongoing challenges.
RL's Triumphs: Game-Changing Applications
Reinforcement learning's success is undeniable in specific domains. Its ability to learn optimal strategies through trial and error has yielded remarkable results:
- Gaming: AlphaGo's victory over a Go world champion was a watershed moment, showcasing RL's power in mastering complex, strategic games. Similar successes have been seen in other games like chess and StarCraft II, highlighting RL's adaptability.
- Robotics: RL algorithms are increasingly used to train robots to perform intricate tasks, from manipulating objects to navigating complex environments. This has implications for automation in manufacturing, logistics, and even healthcare.
- Resource Optimization: In areas like energy management and traffic control, RL algorithms are employed to optimize resource allocation, leading to increased efficiency and cost savings. These applications demonstrate RL's potential for real-world impact.
Limitations and Challenges Facing Reinforcement Learning
Despite its successes, RL faces significant hurdles in its quest to become a truly transformative force in AI:
- Data Dependency: RL algorithms require vast amounts of data for effective training. This data can be expensive and time-consuming to acquire, particularly in real-world scenarios where simulations may not perfectly reflect reality. This data hunger limits its applicability in situations with limited data availability.
- Sample Inefficiency: RL algorithms can be notoriously sample inefficient, meaning they require a large number of trials to learn effectively. This can be a major bottleneck, especially in applications where experimentation is costly or dangerous.
- Interpretability and Explainability: Understanding why an RL agent makes a particular decision can be challenging. This lack of transparency poses significant concerns in applications where trust and accountability are paramount, such as autonomous driving or medical diagnosis.
- Reward Function Design: The effectiveness of an RL agent is heavily reliant on the design of its reward function. Poorly designed reward functions can lead to unintended and undesirable behaviors, highlighting the crucial role of human oversight and careful design.
The Future of Reinforcement Learning in AI
While reinforcement learning presents considerable challenges, its potential remains immense. Ongoing research focuses on addressing its limitations through:
- Improved algorithms: Developing more sample-efficient and robust RL algorithms is a key area of focus.
- Transfer learning: Leveraging knowledge learned in one task to improve performance on another can significantly reduce the data requirements.
- Explainable RL: Creating methods for interpreting and explaining the decisions made by RL agents is crucial for building trust and ensuring responsible deployment.
Conclusion:
Reinforcement learning is undoubtedly a powerful tool in the AI arsenal, driving significant advancements in various fields. However, its limitations cannot be ignored. Addressing these challenges through continued research and development will be crucial in unlocking the full potential of RL and ensuring its responsible integration into our increasingly AI-driven world. The future of AI hinges not only on the successes of RL, but also on a clear understanding of its limitations and a commitment to responsible innovation.
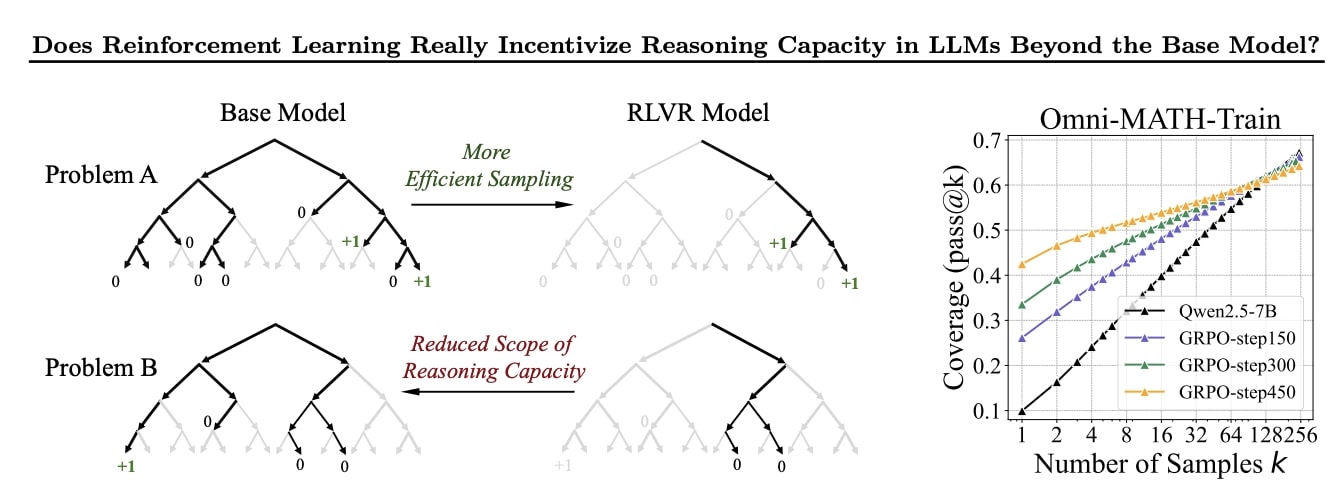
Thank you for visiting our website, your trusted source for the latest updates and in-depth coverage on Does Reinforcement Learning Truly Advance AI? A Data-Driven Perspective. We're committed to keeping you informed with timely and accurate information to meet your curiosity and needs.
If you have any questions, suggestions, or feedback, we'd love to hear from you. Your insights are valuable to us and help us improve to serve you better. Feel free to reach out through our contact page.
Don't forget to bookmark our website and check back regularly for the latest headlines and trending topics. See you next time, and thank you for being part of our growing community!
Featured Posts
-
Preparing For A Power Cut 5 Crucial Items To Stock Up On
Apr 30, 2025 -
National Theatre Announces Star Studded Cast Mescal Wright Barbaro Coughlan
Apr 30, 2025 -
No Fear In Milan Inzaghi On Thurams Selection And Facing Barcelonas Winning Run
Apr 30, 2025 -
Diffusion En Direct Ligue Des Champions Arsenal Affronte Le Psg 2024 2025
Apr 30, 2025 -
Rcb Triumph Highlights Delhi Capitals Tactical Flaws Kumble Bangar React
Apr 30, 2025
Latest Posts
-
9 Years In The Making Star Wars Explains The Origins Of Its Most Mysterious Character
May 01, 2025 -
Ipca Industria E China Previsoes Economicas E Influencia Do Copom
May 01, 2025 -
Security Risks Of Granting Key Access To Ai In Web3 Applications
May 01, 2025 -
From Chain Smoking To Goals The Unexpected Journey Of A Football Star
May 01, 2025 -
Once Titular Del Barca Vs Inter La Alineacion De Hansi Flick
May 01, 2025