Does Reinforcement Learning Truly Enhance AI? A Critical Analysis
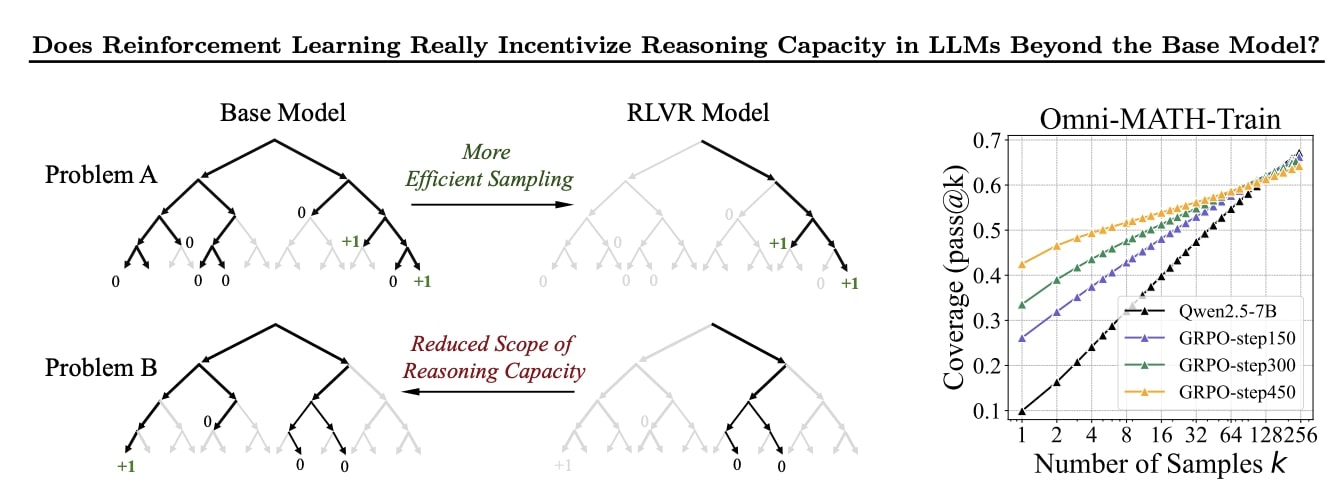
Welcome to your ultimate source for breaking news, trending updates, and in-depth stories from around the world. Whether it's politics, technology, entertainment, sports, or lifestyle, we bring you real-time updates that keep you informed and ahead of the curve.
Our team works tirelessly to ensure you never miss a moment. From the latest developments in global events to the most talked-about topics on social media, our news platform is designed to deliver accurate and timely information, all in one place.
Stay in the know and join thousands of readers who trust us for reliable, up-to-date content. Explore our expertly curated articles and dive deeper into the stories that matter to you. Visit NewsOneSMADCSTDO now and be part of the conversation. Don't miss out on the headlines that shape our world!
Table of Contents
Does Reinforcement Learning Truly Enhance AI? A Critical Analysis
Reinforcement learning (RL), a powerful machine learning technique, has garnered significant attention for its potential to revolutionize artificial intelligence (AI). But does it live up to the hype? This critical analysis delves into the strengths and limitations of RL, exploring whether it truly enhances AI or represents a more nuanced contribution to the field.
Reinforcement learning stands apart from other machine learning approaches by focusing on training AI agents through trial and error. Instead of relying on pre-labeled datasets, RL agents learn by interacting with an environment, receiving rewards for desirable actions and penalties for undesirable ones. This iterative process allows the agent to learn optimal strategies for achieving a specific goal. This approach holds immense promise across diverse fields, from robotics and game playing to personalized medicine and resource management.
H2: The Strengths of Reinforcement Learning in AI Enhancement:
- Adaptability and Generalization: RL agents are inherently adaptable. They can learn to perform well in dynamic and unpredictable environments, making them suitable for real-world applications where conditions constantly change. This adaptability allows for greater generalization compared to supervised learning methods that often struggle with unseen data.
- Solving Complex Problems: RL excels in tackling complex problems that are difficult or impossible to solve using traditional methods. Examples include mastering intricate games like Go and chess, optimizing traffic flow in smart cities, and controlling robotic manipulators in challenging environments.
- Autonomous Learning: The autonomous nature of RL is a major strength. Agents learn without explicit human intervention, discovering optimal strategies independently. This reduces the need for extensive data labeling and human expertise, potentially accelerating AI development.
H2: Limitations and Challenges of Reinforcement Learning:
Despite its potential, RL faces several challenges that hinder its widespread adoption and limit its effectiveness in enhancing AI:
- Sample Inefficiency: RL agents often require a vast number of interactions with the environment to learn effectively. This can be computationally expensive and time-consuming, especially in complex scenarios. This sample inefficiency remains a significant bottleneck.
- Reward Design: Defining appropriate reward functions is crucial for successful RL. Poorly designed rewards can lead to unintended and undesirable behaviors, a phenomenon known as reward hacking. Creating robust and effective reward functions requires significant expertise and careful consideration.
- Interpretability and Explainability: Understanding why an RL agent makes a particular decision can be challenging. The complex nature of RL algorithms often makes it difficult to interpret their internal workings, hindering trust and adoption in critical applications.
- Safety and Robustness: Ensuring the safety and robustness of RL agents is paramount, especially in applications with real-world consequences. Unexpected situations or adversarial attacks can lead to unpredictable and potentially dangerous behavior.
H2: The Future of Reinforcement Learning in AI:
Despite these challenges, the future of RL in enhancing AI looks bright. Active research focuses on addressing these limitations through techniques like:
- Improved algorithm design: Developing more sample-efficient algorithms is a major research focus.
- Advanced reward shaping techniques: Researchers are exploring methods for designing safer and more robust reward functions.
- Explainable AI (XAI) for RL: Efforts are underway to develop techniques to make RL agents more transparent and interpretable.
H2: Conclusion:
Reinforcement learning undoubtedly offers significant advancements in AI. Its ability to learn complex tasks autonomously and adapt to dynamic environments is unparalleled. However, challenges related to sample efficiency, reward design, interpretability, and safety must be addressed before RL can fully realize its potential. Ongoing research and development efforts are crucial to overcome these hurdles and unlock the transformative power of RL in creating truly enhanced and beneficial AI systems. The future of AI is intertwined with the continued progress and refinement of reinforcement learning techniques.
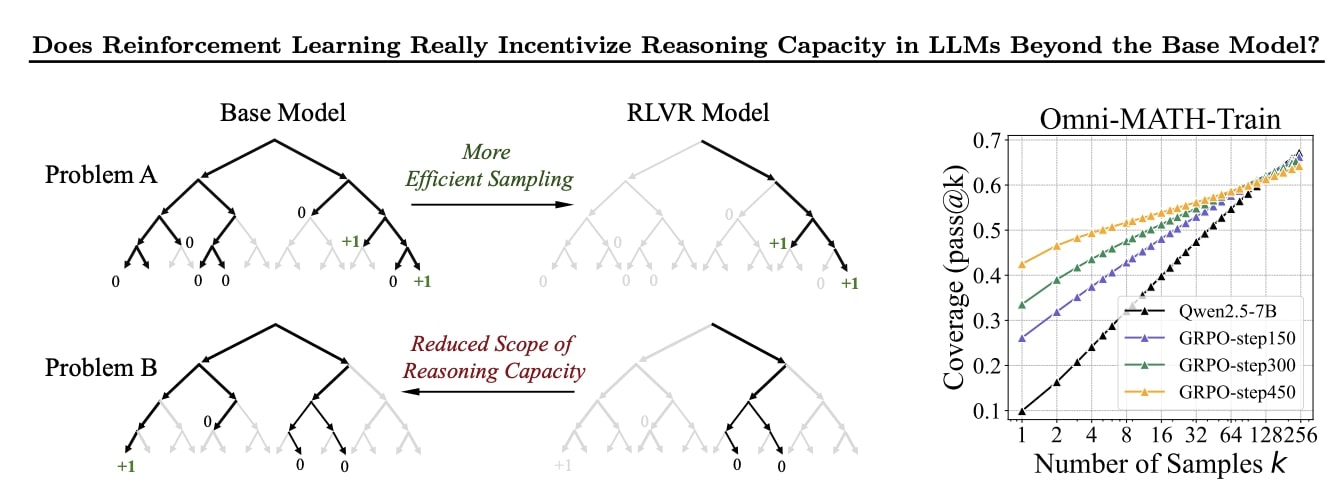
Thank you for visiting our website, your trusted source for the latest updates and in-depth coverage on Does Reinforcement Learning Truly Enhance AI? A Critical Analysis. We're committed to keeping you informed with timely and accurate information to meet your curiosity and needs.
If you have any questions, suggestions, or feedback, we'd love to hear from you. Your insights are valuable to us and help us improve to serve you better. Feel free to reach out through our contact page.
Don't forget to bookmark our website and check back regularly for the latest headlines and trending topics. See you next time, and thank you for being part of our growing community!
Featured Posts
-
Beyond Verification Building True Security In The Web3 Ecosystem
Apr 30, 2025 -
Psg Star The Key Obstacle To Arsenals Champions League Qualification
Apr 30, 2025 -
Live Stream Ronnie O Sullivan Vs Si Jiahui And Judd Trump Vs Luca Brecel World Snooker 2025
Apr 30, 2025 -
Flappy Bird Epic Games Store Secures Exclusive Android Mobile Release
Apr 30, 2025 -
Smashing Machine Trailer Drops Dwayne Johnsons Intense Ufc Fight Scenes
Apr 30, 2025
Latest Posts
-
Dte Energys Proposed Rate Increase 574 Million And The Fight Ahead
Apr 30, 2025 -
2025 Canadian Election Brampton Centres Key Candidates And Polling Predictions
Apr 30, 2025 -
Technical Analysis Hedera Hbar Faces Potential Retracement After Uptrend Slowdown
Apr 30, 2025 -
Compartilhamento De Imoveis Acesso A Praia E Campo Sem A Compra Total
Apr 30, 2025 -
Thunderbolts Post Credits Scene Leaks Online Critic Reactions Pour In
Apr 30, 2025