Does Reinforcement Learning Truly Enhance AI Models? A Critical Analysis
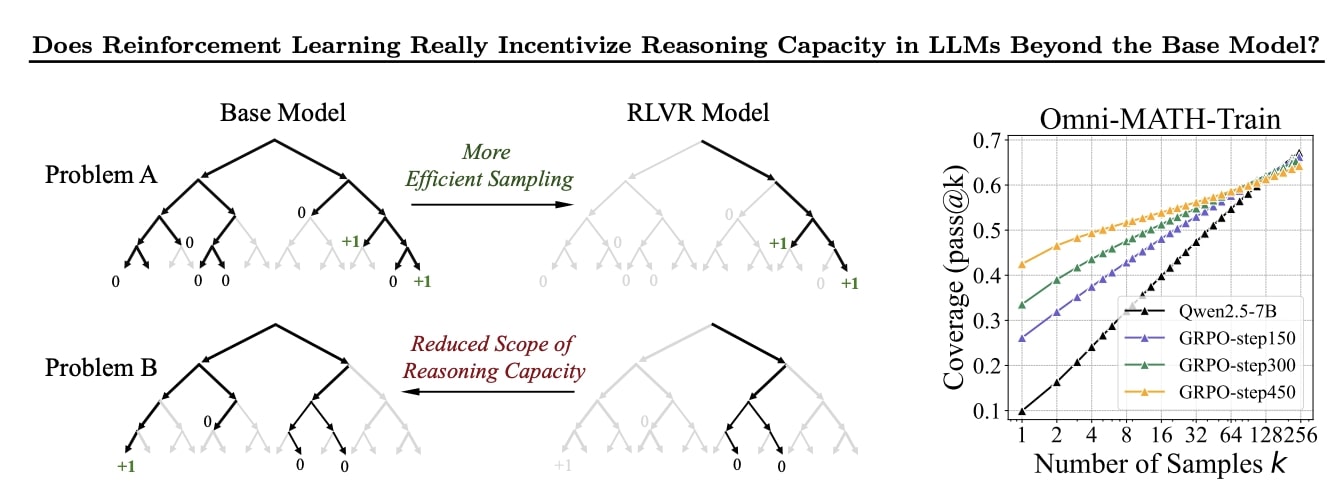
Welcome to your ultimate source for breaking news, trending updates, and in-depth stories from around the world. Whether it's politics, technology, entertainment, sports, or lifestyle, we bring you real-time updates that keep you informed and ahead of the curve.
Our team works tirelessly to ensure you never miss a moment. From the latest developments in global events to the most talked-about topics on social media, our news platform is designed to deliver accurate and timely information, all in one place.
Stay in the know and join thousands of readers who trust us for reliable, up-to-date content. Explore our expertly curated articles and dive deeper into the stories that matter to you. Visit NewsOneSMADCSTDO now and be part of the conversation. Don't miss out on the headlines that shape our world!
Table of Contents
Does Reinforcement Learning Truly Enhance AI Models? A Critical Analysis
Reinforcement learning (RL), a powerful machine learning technique, has garnered significant attention for its potential to enhance AI models across various domains. From mastering complex games like Go to optimizing robotic control systems, RL's ability to learn through trial and error seems revolutionary. But does it truly live up to the hype? This critical analysis delves into the strengths and limitations of RL, examining its real-world impact and future prospects.
Reinforcement learning differs significantly from supervised and unsupervised learning paradigms. Instead of relying on pre-labeled data or identifying patterns in unlabeled data, RL algorithms learn by interacting with an environment. An agent takes actions, receives rewards (or penalties), and learns to maximize its cumulative reward over time. This iterative process, often described as learning through trial and error, allows RL agents to solve complex problems that are difficult to tackle with other methods.
H2: The Strengths of Reinforcement Learning
- Adaptability and Generalization: RL excels in dynamic environments where rules change frequently. Unlike traditional algorithms that require extensive pre-programmed knowledge, RL agents adapt and learn new strategies on the fly. This adaptability makes them particularly well-suited for real-world applications, where unforeseen circumstances are common.
- Problem-Solving Capabilities: RL has demonstrated remarkable success in solving complex, high-dimensional problems, often exceeding human performance in specific tasks. Game playing (AlphaGo being a prime example), robotics control, and resource management are areas where RL has shown significant promise.
- Automation and Optimization: RL can automate complex processes and optimize performance in various systems. In areas like supply chain management or traffic optimization, RL algorithms can significantly improve efficiency and reduce costs.
H2: The Challenges and Limitations of Reinforcement Learning
Despite its potential, RL faces several significant challenges that hinder its widespread adoption:
- Data Efficiency: RL algorithms often require vast amounts of data and significant computational resources for training. This can be prohibitively expensive and time-consuming, especially in real-world applications where data acquisition can be challenging.
- Reward Function Design: Defining an appropriate reward function is crucial for successful RL training. A poorly designed reward function can lead to unexpected and undesirable behavior, a problem known as reward hacking. Crafting effective reward functions often requires significant expertise and careful consideration.
- Sample Inefficiency: RL algorithms can be notoriously sample inefficient, meaning they require a large number of interactions with the environment to learn effectively. This makes them unsuitable for applications where interactions are costly or dangerous.
- Explainability and Interpretability: Understanding why an RL agent makes a particular decision can be difficult. The complex nature of many RL algorithms makes it challenging to interpret their internal workings, limiting their applicability in situations where transparency and accountability are paramount.
H2: Real-World Applications and Future Directions
Despite these limitations, RL is making significant inroads into various fields:
- Robotics: RL is used to train robots to perform complex tasks such as grasping objects, navigating cluttered environments, and collaborating with humans.
- Healthcare: RL algorithms are being developed to personalize treatment plans, optimize drug discovery, and improve healthcare resource allocation.
- Finance: RL is used for algorithmic trading, risk management, and portfolio optimization.
Future research in RL focuses on addressing its limitations: improving data efficiency, developing more robust reward function design techniques, and enhancing the explainability and interpretability of RL algorithms. The development of more efficient algorithms, particularly those leveraging transfer learning and meta-learning, holds significant promise for expanding the applicability of RL.
H2: Conclusion:
Reinforcement learning is a powerful tool with the potential to revolutionize AI. While it faces significant challenges, ongoing research and development are steadily addressing these limitations. The continued refinement of RL algorithms, coupled with increased computational power and access to larger datasets, suggests that its impact on AI and various industries will only continue to grow. However, a balanced perspective is crucial, recognizing both its immense potential and the significant hurdles that remain to be overcome before RL can be seamlessly integrated into a wider range of applications.
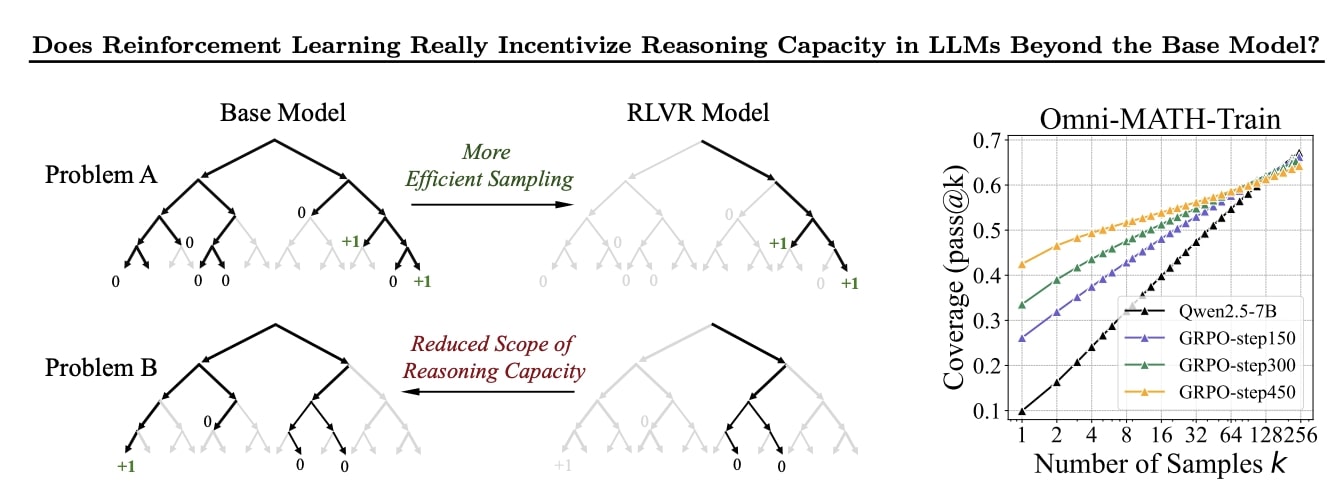
Thank you for visiting our website, your trusted source for the latest updates and in-depth coverage on Does Reinforcement Learning Truly Enhance AI Models? A Critical Analysis. We're committed to keeping you informed with timely and accurate information to meet your curiosity and needs.
If you have any questions, suggestions, or feedback, we'd love to hear from you. Your insights are valuable to us and help us improve to serve you better. Feel free to reach out through our contact page.
Don't forget to bookmark our website and check back regularly for the latest headlines and trending topics. See you next time, and thank you for being part of our growing community!
Featured Posts
-
A New Era In Mars Exploration The Impact Of Comprehensive Planetary Mapping
Apr 29, 2025 -
Neck Injury Forces Early Retirement For Steelers Ryan Watts
Apr 29, 2025 -
Ipl 2024 Karim Janat Joins Elite List Of Afghan Cricketers
Apr 29, 2025 -
Akash Madhwal The Reason Behind His Absence From Todays Mi Match
Apr 29, 2025 -
Hans Zimmers Sydney Concert A Live Review
Apr 29, 2025
Latest Posts
-
Update Lutnicks International Business Deal Hinges On Foreign Parliament
Apr 30, 2025 -
Tensions Rise Trump Confronts Bezos On Amazons Tariff Strategy
Apr 30, 2025 -
3 000 Acre Stronghold Fire Increased Fire Behavior Expected
Apr 30, 2025 -
Paramount Streaming Guide Everything Coming In May 2025
Apr 30, 2025 -
Strong Winds Fan Wildfire Southeast Of Tucson Leading To Mandatory Evacuations
Apr 30, 2025