Evaluating The Actual Impact Of Reinforcement Learning On AI
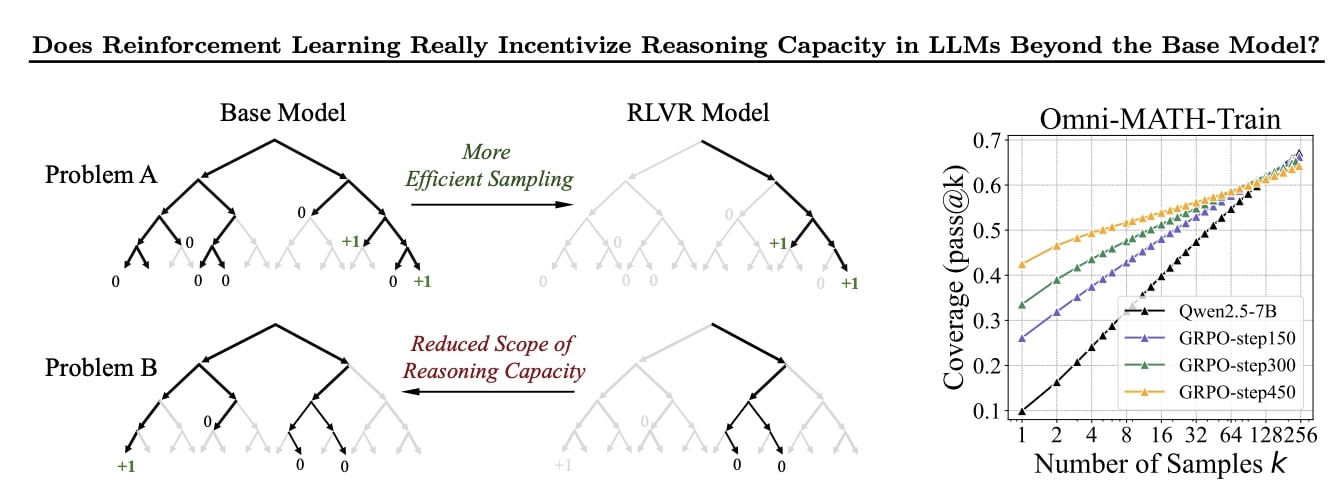
Welcome to your ultimate source for breaking news, trending updates, and in-depth stories from around the world. Whether it's politics, technology, entertainment, sports, or lifestyle, we bring you real-time updates that keep you informed and ahead of the curve.
Our team works tirelessly to ensure you never miss a moment. From the latest developments in global events to the most talked-about topics on social media, our news platform is designed to deliver accurate and timely information, all in one place.
Stay in the know and join thousands of readers who trust us for reliable, up-to-date content. Explore our expertly curated articles and dive deeper into the stories that matter to you. Visit NewsOneSMADCSTDO now and be part of the conversation. Don't miss out on the headlines that shape our world!
Table of Contents
Evaluating the Actual Impact of Reinforcement Learning on AI: Hype vs. Reality
Reinforcement learning (RL), a subfield of machine learning, has captured the imagination of the tech world. Promising breakthroughs in robotics, game playing, and even scientific discovery, it's often hailed as the key to unlocking truly intelligent AI. But how much of this is hype, and how much is reality? This article dives deep into the actual impact of RL on the AI landscape, separating the exciting possibilities from the current limitations.
Reinforcement learning, at its core, involves training an agent to make decisions in an environment by rewarding desirable actions and penalizing undesirable ones. This iterative process allows the agent to learn optimal strategies through trial and error. While conceptually elegant, the practical application presents several significant challenges.
The Triumphs of Reinforcement Learning: Real-World Applications
Despite its challenges, RL has undeniably achieved impressive feats. Consider these successes:
- Game Playing: DeepMind's AlphaGo, which defeated a world champion Go player, is a prime example. This victory showcased the potential of RL to master complex strategic games previously considered insurmountable for AI. Subsequent iterations, like AlphaZero, further demonstrated RL's versatility by mastering chess and shogi with minimal prior knowledge.
- Robotics: RL is increasingly used to train robots for complex tasks, such as manipulation, locomotion, and navigation. From warehouse automation to surgical robotics, the potential for improved efficiency and precision is vast. However, challenges remain in translating simulated successes to the real world.
- Resource Optimization: RL algorithms are being employed to optimize resource allocation in various fields, including energy grids, traffic management, and supply chain logistics. By learning optimal strategies for resource distribution, RL can lead to significant cost savings and increased efficiency.
The Limitations of Reinforcement Learning: Addressing the Challenges
While the successes are noteworthy, it's crucial to acknowledge the limitations that hinder widespread adoption:
- Data Requirements: RL algorithms often require massive amounts of data for training, which can be expensive and time-consuming to acquire. This data dependency limits the applicability of RL to scenarios where sufficient data is readily available.
- Sample Inefficiency: RL agents can be notoriously sample inefficient, meaning they require many trials to learn effectively. This can make training computationally expensive and slow, particularly for complex tasks.
- Safety and Robustness: Ensuring the safety and robustness of RL agents is a critical concern. Unpredictable behavior and potential for catastrophic failures can arise if the agent encounters unforeseen situations or exploits vulnerabilities in the environment. This necessitates robust safety mechanisms and careful consideration of potential risks.
- Explainability and Interpretability: Understanding why an RL agent makes a particular decision can be challenging. The complex nature of many RL algorithms makes it difficult to interpret their internal workings, hindering trust and hindering debugging. This "black box" nature limits the applicability of RL in high-stakes domains where transparency is crucial.
The Future of Reinforcement Learning: Navigating the Path Ahead
The future of reinforcement learning hinges on addressing these limitations. Active research areas include:
- Developing more sample-efficient algorithms: This will allow RL to be applied to more complex tasks with limited data.
- Improving safety and robustness: Developing techniques to ensure reliable and predictable behavior in real-world scenarios is paramount.
- Enhancing explainability and interpretability: Making RL agents more transparent will build trust and facilitate wider adoption.
- Transfer learning: Enabling an agent to transfer knowledge learned in one environment to another will significantly reduce training time and data requirements.
In conclusion, while the hype surrounding reinforcement learning is undeniable, its real-world impact is still evolving. While significant progress has been made, addressing the challenges related to data requirements, sample efficiency, safety, and explainability is crucial for unlocking its full potential. The future of AI likely involves a sophisticated interplay between RL and other machine learning techniques, paving the way for more robust, efficient, and trustworthy intelligent systems.
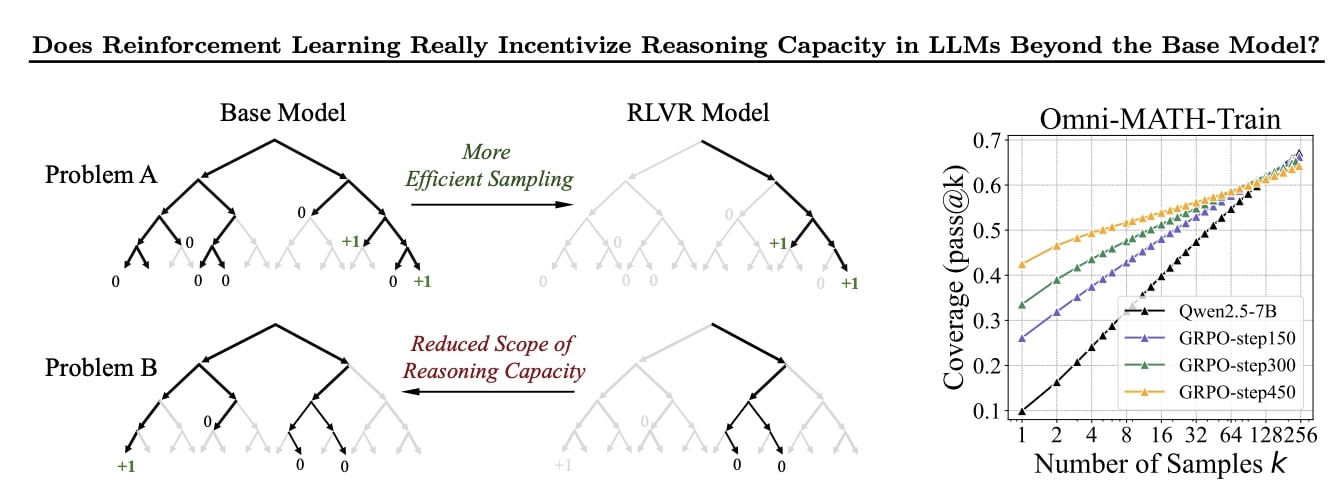
Thank you for visiting our website, your trusted source for the latest updates and in-depth coverage on Evaluating The Actual Impact Of Reinforcement Learning On AI. We're committed to keeping you informed with timely and accurate information to meet your curiosity and needs.
If you have any questions, suggestions, or feedback, we'd love to hear from you. Your insights are valuable to us and help us improve to serve you better. Feel free to reach out through our contact page.
Don't forget to bookmark our website and check back regularly for the latest headlines and trending topics. See you next time, and thank you for being part of our growing community!
Featured Posts
-
Forbidden Stories The Perilous Pursuit Of Truth In Ukraines War Zone
Apr 30, 2025 -
Study Ai Shows Promise In Guide Dog Puppy Assessment
Apr 30, 2025 -
Amazon Report Sparks Trumps Reported Call To Bezos Sources Say
Apr 30, 2025 -
Analyzing The Data Effectiveness Of Trump And Bidens Facebook Ads Targeting Older Women
Apr 30, 2025 -
Nuno Mendes To Man United Transfer Update Following 29m Psg Move
Apr 30, 2025
Latest Posts
-
Justice Gorsuch Scolds Litigator In Unprecedented Supreme Court Exchange
Apr 30, 2025 -
Arsenal Psg Rematch Luis Enrique Urges Team To Rewrite The Narrative
Apr 30, 2025 -
Mertens Falls To Sabalenka In Three Sets Belarusians Ninth Straight Win
Apr 30, 2025 -
Disability Rights Face Supreme Court Scrutiny Will Landmark Ruling Emerge
Apr 30, 2025 -
Ftc V Meta Live Updates On Instagram And Whats App Case
Apr 30, 2025