Evaluating The Impact Of Reinforcement Learning On AI Model Performance
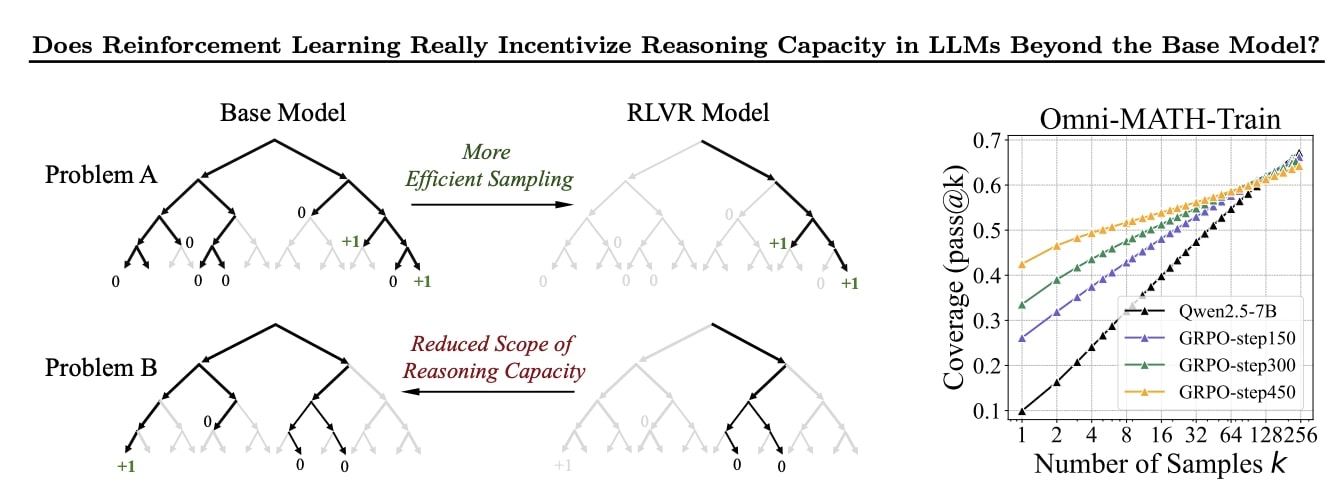
Welcome to your ultimate source for breaking news, trending updates, and in-depth stories from around the world. Whether it's politics, technology, entertainment, sports, or lifestyle, we bring you real-time updates that keep you informed and ahead of the curve.
Our team works tirelessly to ensure you never miss a moment. From the latest developments in global events to the most talked-about topics on social media, our news platform is designed to deliver accurate and timely information, all in one place.
Stay in the know and join thousands of readers who trust us for reliable, up-to-date content. Explore our expertly curated articles and dive deeper into the stories that matter to you. Visit NewsOneSMADCSTDO now and be part of the conversation. Don't miss out on the headlines that shape our world!
Table of Contents
Evaluating the Impact of Reinforcement Learning on AI Model Performance: A New Era of Intelligent Systems?
Reinforcement learning (RL) is rapidly transforming the landscape of artificial intelligence, pushing the boundaries of what AI models can achieve. But how significant is its impact on overall model performance? This article delves into the evolving relationship between RL and AI performance, exploring its benefits, limitations, and the ongoing research shaping its future.
The Rise of Reinforcement Learning in AI
Reinforcement learning, a subfield of machine learning, focuses on training AI agents to make optimal decisions in an environment. Unlike supervised learning, which relies on labeled data, RL agents learn through trial and error, receiving rewards for desirable actions and penalties for undesirable ones. This iterative process allows agents to adapt and improve their performance over time, leading to increasingly sophisticated AI systems.
Key Benefits of Integrating Reinforcement Learning:
- Improved Decision-Making: RL empowers AI models to make more informed and strategic decisions, particularly in complex and dynamic environments where traditional methods struggle. This is evident in applications like game playing (AlphaGo), robotics, and resource management.
- Enhanced Adaptability: RL agents are inherently adaptable. They can learn and adjust to changing conditions, making them more robust and resilient to unforeseen circumstances. This adaptability is crucial in real-world scenarios where environments are rarely static.
- Automation of Complex Tasks: RL facilitates the automation of tasks that are difficult or impossible to program explicitly. This opens up possibilities in areas like autonomous driving, personalized medicine, and supply chain optimization.
- Solving Previously Unsolvable Problems: Certain problems, particularly those involving sequential decision-making under uncertainty, are better tackled using RL. This leads to innovations in areas where traditional AI methods fall short.
Limitations and Challenges:
Despite its significant advantages, RL faces several challenges:
- Reward Function Design: Defining an appropriate reward function is crucial, yet often complex. A poorly designed reward function can lead the agent to achieve unintended or undesirable outcomes. This is known as the "reward hacking" problem.
- Sample Efficiency: RL algorithms often require a vast amount of data and training time, making them computationally expensive and potentially limiting their applicability.
- Generalization: RL agents may struggle to generalize their learned behaviors to new or unseen environments. This lack of generalization can hinder the deployment of RL models in real-world applications.
- Interpretability: Understanding the decision-making process of an RL agent can be challenging. This lack of interpretability raises concerns about transparency and accountability, particularly in high-stakes applications.
Measuring the Impact on AI Model Performance:
Evaluating the impact of RL on AI model performance requires a multifaceted approach. Researchers employ various metrics, including:
- Accuracy: Measuring the correctness of the agent's actions.
- Efficiency: Assessing the computational resources and time required for training and execution.
- Robustness: Evaluating the ability of the agent to handle unexpected situations and noisy data.
- Generalizability: Testing the agent's performance in different environments and scenarios.
Future Directions and Research:
Ongoing research is addressing the limitations of RL, focusing on:
- Developing more sample-efficient algorithms: Reducing the amount of data required for training.
- Improving reward function design techniques: Ensuring agents learn desirable behaviors.
- Enhancing the interpretability of RL models: Making the decision-making process more transparent.
- Exploring the integration of RL with other AI techniques: Combining the strengths of different approaches to create more powerful systems.
Conclusion:
Reinforcement learning is a transformative technology with the potential to significantly enhance the performance of AI models across various domains. While challenges remain, ongoing research and development are paving the way for a future where RL plays an even more crucial role in building intelligent and adaptable AI systems. The continuous evolution of RL promises exciting advancements and the potential to solve some of humanity's most pressing challenges.
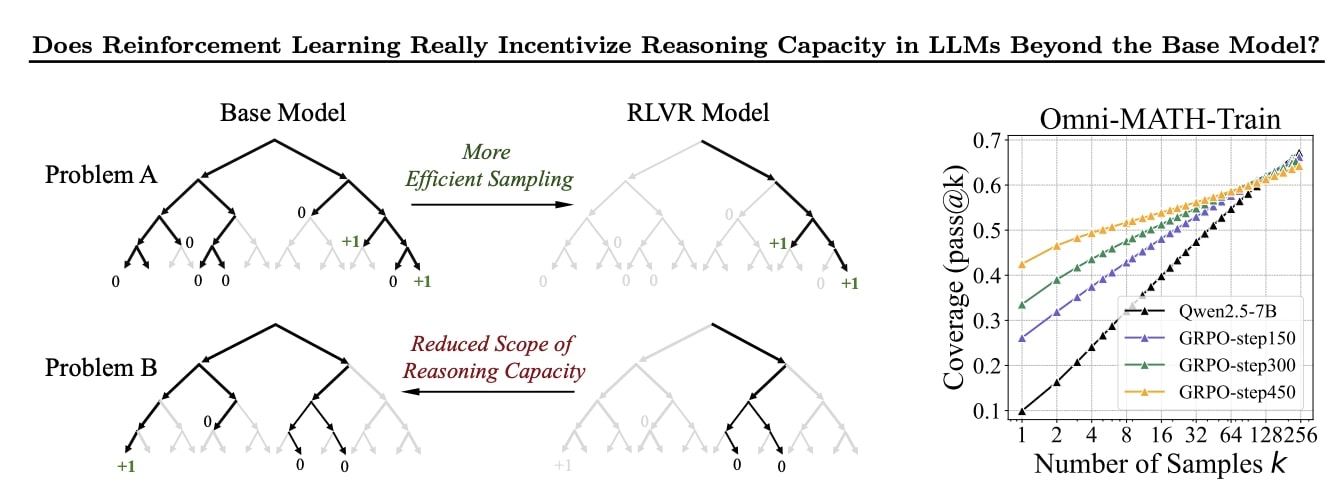
Thank you for visiting our website, your trusted source for the latest updates and in-depth coverage on Evaluating The Impact Of Reinforcement Learning On AI Model Performance. We're committed to keeping you informed with timely and accurate information to meet your curiosity and needs.
If you have any questions, suggestions, or feedback, we'd love to hear from you. Your insights are valuable to us and help us improve to serve you better. Feel free to reach out through our contact page.
Don't forget to bookmark our website and check back regularly for the latest headlines and trending topics. See you next time, and thank you for being part of our growing community!
Featured Posts
-
Snapdragon Cup Pre Season Match Confirmed At Old Trafford
May 02, 2025 -
Wsl Match Report Chelsea Defeat Man Utd Tullis Joyces Mistake Proves Crucial
May 02, 2025 -
Europa League Live Tottenhams Semi Final Clash Against Bodo Glimt Updates And Highlights
May 02, 2025 -
Whoopi Goldberg Silences Meghan Markle Discussion On Live Television
May 02, 2025 -
Fiorentina Vs Real Betis Pellegrini Predicts Tough Match
May 02, 2025