Is Reinforcement Learning The AI Revolution We Expected? A Critical Analysis.
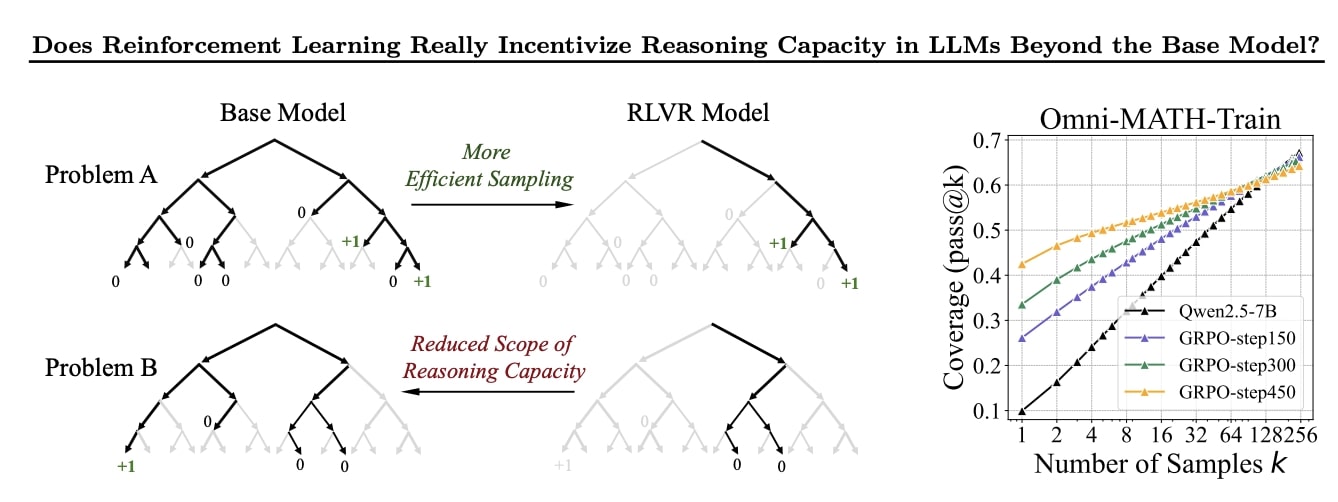
Welcome to your ultimate source for breaking news, trending updates, and in-depth stories from around the world. Whether it's politics, technology, entertainment, sports, or lifestyle, we bring you real-time updates that keep you informed and ahead of the curve.
Our team works tirelessly to ensure you never miss a moment. From the latest developments in global events to the most talked-about topics on social media, our news platform is designed to deliver accurate and timely information, all in one place.
Stay in the know and join thousands of readers who trust us for reliable, up-to-date content. Explore our expertly curated articles and dive deeper into the stories that matter to you. Visit NewsOneSMADCSTDO now and be part of the conversation. Don't miss out on the headlines that shape our world!
Table of Contents
Is Reinforcement Learning the AI Revolution We Expected? A Critical Analysis
Reinforcement learning (RL), a subfield of artificial intelligence (AI) where agents learn to make optimal decisions through trial and error, has been touted as a potential game-changer. From self-driving cars to sophisticated robotics, the promise of RL is immense. But is it living up to the hype? This critical analysis delves into the achievements and limitations of RL, examining whether it's truly the AI revolution we anticipated.
The Hype Around Reinforcement Learning
The initial excitement surrounding RL stemmed from its impressive achievements in complex game environments. DeepMind's AlphaGo, which defeated a world champion Go player using RL, captured the world's imagination. This victory demonstrated the potential of RL to solve problems previously considered intractable for AI. Subsequently, AlphaZero, a more generalized RL agent, mastered Go, chess, and shogi with superhuman proficiency, further fueling the belief that RL was a revolutionary technology poised to transform numerous industries.
Real-World Applications and Limitations
While RL has shown promise in specific areas, its widespread adoption faces significant challenges:
-
Data Requirements: RL algorithms typically require vast amounts of data for training. Gathering and labeling this data can be expensive, time-consuming, and sometimes impossible in real-world scenarios. This data hunger limits its applicability in domains with limited data availability.
-
Sample Inefficiency: RL agents can be notoriously sample inefficient, meaning they require a large number of trials to learn effectively. This inefficiency translates to significant computational costs and longer training times, hindering its deployment in time-sensitive applications.
-
Safety and Robustness: RL agents learn through trial and error, which can lead to unpredictable and potentially unsafe behavior, especially in real-world settings where mistakes can have serious consequences. Ensuring the safety and robustness of RL agents remains a major research hurdle.
-
Interpretability and Explainability: Understanding why an RL agent makes a specific decision is often difficult. The complex nature of many RL algorithms makes it challenging to interpret their internal decision-making processes, raising concerns about transparency and accountability. This lack of explainability is a significant barrier to its adoption in high-stakes domains like healthcare and finance.
The Path Forward for Reinforcement Learning
Despite these limitations, RL continues to be an active area of research. Several promising avenues are being explored to address its shortcomings:
-
Improved Algorithms: Researchers are developing more sample-efficient and robust RL algorithms, aiming to reduce training time and improve safety.
-
Transfer Learning: Applying knowledge learned in one environment to a new environment can significantly reduce the data requirements for RL. This transfer learning approach holds immense potential for accelerating RL adoption.
-
Simulation Environments: Using realistic simulations to train RL agents allows for extensive experimentation without the risks associated with real-world deployments. This approach is crucial for improving safety and reducing the cost of training.
-
Explainable AI (XAI): Developments in XAI are aimed at making RL agents more transparent and understandable, improving trust and accountability.
Conclusion:
Reinforcement learning has undoubtedly made significant strides, showcasing impressive capabilities in controlled environments. However, its real-world application faces considerable challenges related to data requirements, sample efficiency, safety, and explainability. While not the immediate revolution some predicted, RL remains a powerful tool with immense potential. Continued research and innovation in areas like algorithm improvement, transfer learning, and XAI are crucial to unlock its full potential and address its limitations, paving the way for its broader adoption and a more impactful role in the future of AI.
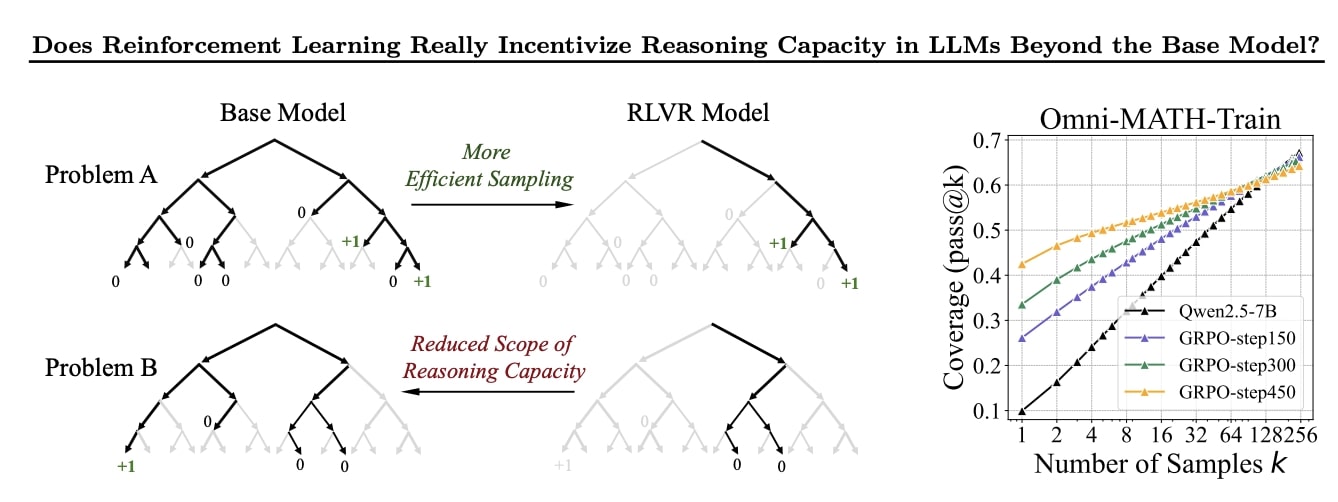
Thank you for visiting our website, your trusted source for the latest updates and in-depth coverage on Is Reinforcement Learning The AI Revolution We Expected? A Critical Analysis.. We're committed to keeping you informed with timely and accurate information to meet your curiosity and needs.
If you have any questions, suggestions, or feedback, we'd love to hear from you. Your insights are valuable to us and help us improve to serve you better. Feel free to reach out through our contact page.
Don't forget to bookmark our website and check back regularly for the latest headlines and trending topics. See you next time, and thank you for being part of our growing community!
Featured Posts
-
Otp 2 Satellite Analyzing The Reduced Orbital Decay Of A Propellantless Drive
Apr 29, 2025 -
Live Score Rr Vs Gt Ipl 2025 Match Gujarat Titans New Captain Takes Charge
Apr 29, 2025 -
One Plus 13 R Performance And Camera A Detailed Review
Apr 29, 2025 -
Trump Vs Biden Competing For The Older Women Demographic On Facebook
Apr 29, 2025 -
Billy Joel And Christie Brinkley Brinkley Publicly Identifies The Third Party In Their Marriage
Apr 29, 2025
Latest Posts
-
Billy Joels Infidelity Christie Brinkley Identifies The Woman Involved
Apr 29, 2025 -
Trump Triumphs Over Murphy The Role Of His Very Special Player
Apr 29, 2025 -
Hamiltons F1 Form Mark Hughes On The Reasons Behind Ferraris Recent Struggles
Apr 29, 2025 -
Five Critically Acclaimed Netflix Films Arriving May 2025
Apr 29, 2025 -
Will Foreign Interference Decide Singapores Next Election A Close Race Predicted
Apr 29, 2025