Is Reinforcement Learning The AI Silver Bullet We Thought It Was?
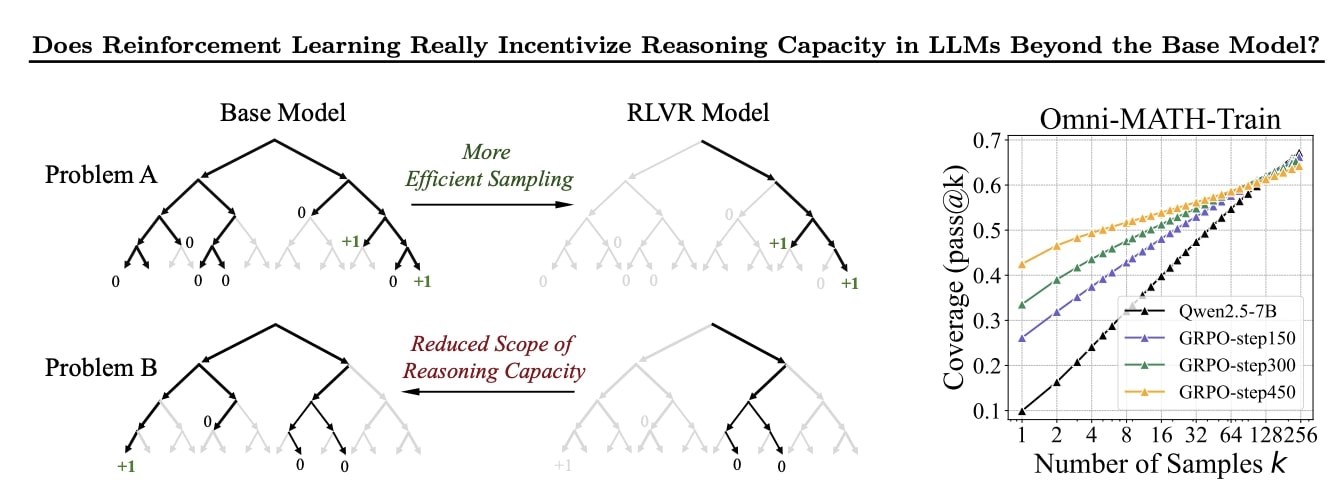
Welcome to your ultimate source for breaking news, trending updates, and in-depth stories from around the world. Whether it's politics, technology, entertainment, sports, or lifestyle, we bring you real-time updates that keep you informed and ahead of the curve.
Our team works tirelessly to ensure you never miss a moment. From the latest developments in global events to the most talked-about topics on social media, our news platform is designed to deliver accurate and timely information, all in one place.
Stay in the know and join thousands of readers who trust us for reliable, up-to-date content. Explore our expertly curated articles and dive deeper into the stories that matter to you. Visit NewsOneSMADCSTDO now and be part of the conversation. Don't miss out on the headlines that shape our world!
Table of Contents
Is Reinforcement Learning the AI Silver Bullet We Thought It Was? The Hype vs. the Reality
Reinforcement learning (RL), the AI technique that allows machines to learn through trial and error, has been hailed as a potential silver bullet for solving complex problems. From self-driving cars to sophisticated robotics, its applications seemed limitless. But as the field matures, a more nuanced picture is emerging. Is RL truly living up to the hype, or are there significant limitations hindering its widespread adoption?
The Allure of Reinforcement Learning:
The initial excitement surrounding RL stemmed from its ability to tackle problems beyond the reach of traditional supervised learning methods. By rewarding desirable behaviors and penalizing undesirable ones, RL algorithms can learn optimal strategies in complex, dynamic environments. This offered a compelling solution for tasks requiring adaptability and decision-making in real-time, such as:
- Robotics: Training robots to perform intricate tasks like assembly or manipulation in unstructured environments.
- Game Playing: Achieving superhuman performance in games like Go and chess, showcasing the power of RL algorithms.
- Autonomous Vehicles: Developing self-driving systems capable of navigating complex traffic situations and making safe driving decisions.
- Personalized Recommendations: Tailoring recommendations in e-commerce and entertainment to individual user preferences.
The Challenges Facing Reinforcement Learning:
Despite its successes, RL faces significant hurdles that prevent it from becoming the universal solution it was once projected to be:
- Data Efficiency: RL algorithms often require massive amounts of data and extensive training time to achieve satisfactory performance. This can be prohibitively expensive and time-consuming, particularly in real-world applications.
- Sample Inefficiency: Exploring the vast space of possible actions can be computationally expensive and lead to inefficient learning. Finding a balance between exploration and exploitation remains a key challenge.
- Reward Shaping: Designing effective reward functions is crucial for guiding the learning process. Poorly designed reward functions can lead to unexpected and undesirable behavior, highlighting the need for careful design and rigorous testing.
- Generalization: RL agents often struggle to generalize their learned behavior to new, unseen situations. This limits their applicability in dynamic and unpredictable environments.
- Safety and Robustness: Ensuring the safety and robustness of RL agents is paramount, particularly in critical applications like autonomous driving. Unforeseen behaviors can lead to catastrophic consequences.
Beyond the Hype: A Balanced Perspective:
While RL isn't the panacea initially envisioned, it remains a powerful tool with significant potential. Researchers are actively addressing the challenges mentioned above through innovations such as:
- Transfer learning: Leveraging knowledge gained in one task to improve performance in related tasks.
- Imitation learning: Using demonstrations from human experts to guide the learning process.
- Sim-to-real transfer: Training RL agents in simulated environments and then transferring the learned policies to the real world.
The Future of Reinforcement Learning:
Reinforcement learning's future hinges on overcoming its current limitations. Continued research into more efficient algorithms, improved reward shaping techniques, and robust safety mechanisms is crucial. While it may not be the silver bullet we initially hoped for, RL's potential to revolutionize various fields remains undeniable. As researchers continue to refine its capabilities, we can expect to see even more impressive applications in the years to come. The journey toward fully realizing RL's potential is ongoing, but the destination promises transformative advancements across numerous industries.
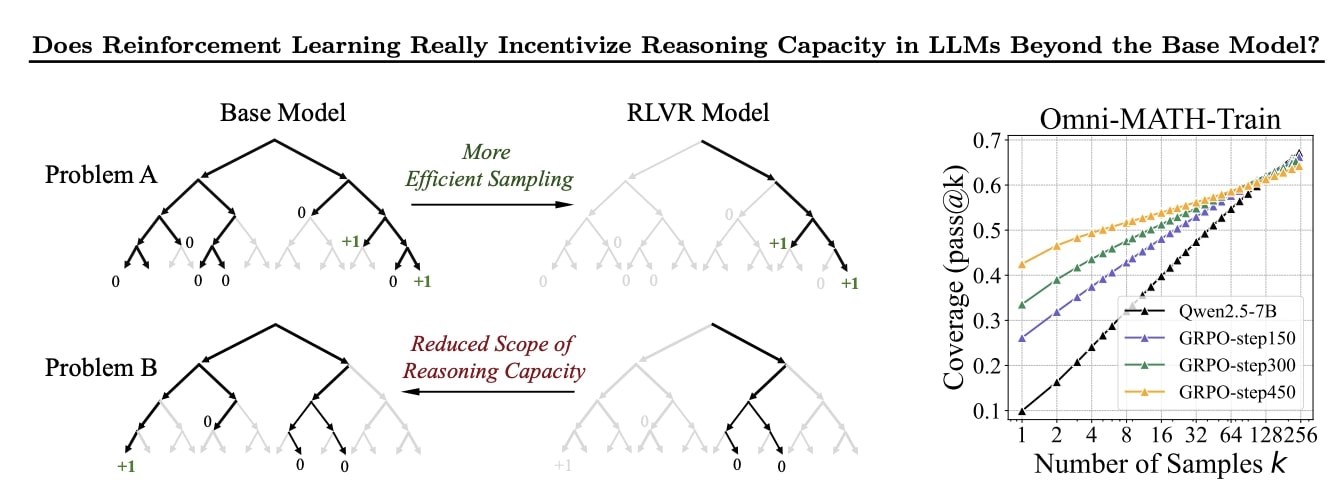
Thank you for visiting our website, your trusted source for the latest updates and in-depth coverage on Is Reinforcement Learning The AI Silver Bullet We Thought It Was?. We're committed to keeping you informed with timely and accurate information to meet your curiosity and needs.
If you have any questions, suggestions, or feedback, we'd love to hear from you. Your insights are valuable to us and help us improve to serve you better. Feel free to reach out through our contact page.
Don't forget to bookmark our website and check back regularly for the latest headlines and trending topics. See you next time, and thank you for being part of our growing community!
Featured Posts
-
Farioli On Ajax A Demand For Respect And Full Attention
Apr 28, 2025 -
Tragedy Strikes Ru Pauls Drag Races Jiggly Caliente Dies At 44
Apr 28, 2025 -
Cherry Targets Man Utds Weakness A Calculated Business Strategy
Apr 28, 2025 -
Vera Finale Brenda Blethyn On Saying Goodbye To Iconic Hat And Coat
Apr 28, 2025 -
Crise No Rs Gerdau Suspende Producao Em Face Das Intemperies
Apr 28, 2025
Latest Posts
-
3 Critical Token Unlocks Impacting Crypto Markets Next Week
Apr 29, 2025 -
Millions Affected Major Power Outage Hits Spain And Portugal
Apr 29, 2025 -
New Metal Mario Hot Wheels Die Cast Release Date And Details
Apr 29, 2025 -
Analyzing Hamiltons Ferrari Performance Insights From Mark Hughes On F1s Biggest Mystery
Apr 29, 2025 -
Guide Electoral Les Circonscriptions Les Plus Incertaines
Apr 29, 2025