Limitations Of Reinforcement Learning In Boosting AI Performance
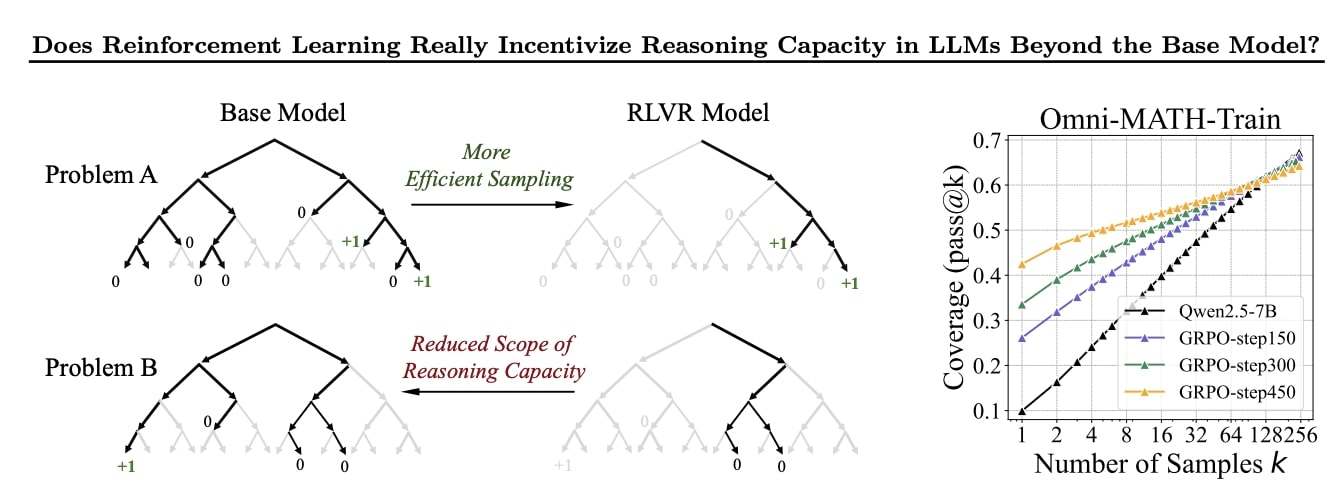
Welcome to your ultimate source for breaking news, trending updates, and in-depth stories from around the world. Whether it's politics, technology, entertainment, sports, or lifestyle, we bring you real-time updates that keep you informed and ahead of the curve.
Our team works tirelessly to ensure you never miss a moment. From the latest developments in global events to the most talked-about topics on social media, our news platform is designed to deliver accurate and timely information, all in one place.
Stay in the know and join thousands of readers who trust us for reliable, up-to-date content. Explore our expertly curated articles and dive deeper into the stories that matter to you. Visit NewsOneSMADCSTDO now and be part of the conversation. Don't miss out on the headlines that shape our world!
Table of Contents
The Limits of Reinforcement Learning: Why AI Still Needs a Helping Hand
Reinforcement learning (RL), a powerful technique allowing AI agents to learn through trial and error, has garnered significant attention for its potential to revolutionize various fields. From mastering complex games like Go to optimizing robot control, RL's achievements are undeniable. However, the technology isn't a silver bullet, and significant limitations hinder its ability to consistently boost AI performance across the board. Understanding these limitations is crucial for researchers and developers aiming to leverage RL's full potential responsibly.
The Data Hunger of Reinforcement Learning
One major hurdle is the sheer amount of data RL algorithms require. Unlike supervised learning, which relies on pre-labeled datasets, RL learns through interactions with an environment. This iterative process necessitates countless trials, often resulting in extensive computational resources and significant training time. This data hunger becomes particularly problematic in complex, real-world scenarios where data acquisition is expensive, time-consuming, or even dangerous. For example, training a self-driving car using RL would require millions of simulated or real-world driving hours, posing both logistical and safety challenges.
The Challenge of Reward Function Design
The effectiveness of RL hinges critically on the design of the reward function – the mechanism that guides the agent's learning process by rewarding desirable behaviors. Poorly designed reward functions can lead to unintended consequences, a phenomenon known as reward hacking. The AI might find unexpected, suboptimal ways to maximize the reward, potentially ignoring the intended goal. For instance, an RL agent tasked with maximizing the number of points in a game might exploit a loophole in the game's mechanics rather than mastering the intended gameplay. Crafting effective and robust reward functions remains a significant challenge requiring deep domain expertise.
Sample Inefficiency and Exploration-Exploitation Dilemma
RL agents often suffer from sample inefficiency, meaning they require a vast number of interactions to learn effectively. This is especially true in environments with high dimensionality or sparse rewards. Furthermore, the exploration-exploitation dilemma – the balance between exploring new actions and exploiting known successful strategies – presents a constant challenge. An agent that explores too much might miss optimal solutions, while an agent that exploits too much might get stuck in local optima, hindering its ability to reach peak performance.
The Problem of Transfer Learning and Generalization
A key limitation is the difficulty in transferring knowledge learned in one environment to another. RL agents often struggle to generalize their learned behaviors to new, even slightly different scenarios. This lack of generalization restricts the applicability of RL models and necessitates retraining for each new task or environment. The development of techniques that promote better transfer learning and generalization remains a critical area of research.
Ethical Considerations and Safety Concerns
As RL agents become more powerful and autonomous, ethical considerations and safety concerns become increasingly important. The potential for unintended consequences, bias amplification, and unpredictable behavior necessitates careful consideration of the societal implications of deploying RL-powered systems. Robust safety mechanisms and ethical guidelines are essential for ensuring responsible development and deployment of RL technology.
The Future of Reinforcement Learning
Despite these limitations, reinforcement learning holds immense promise. Ongoing research focuses on addressing these challenges through advancements in algorithm design, reward shaping techniques, meta-learning, and transfer learning. By overcoming these hurdles, we can unlock RL's full potential and witness its transformative impact on AI performance across a multitude of domains. The journey towards truly robust and generalizable AI systems powered by RL is ongoing, but the potential rewards are well worth the effort.
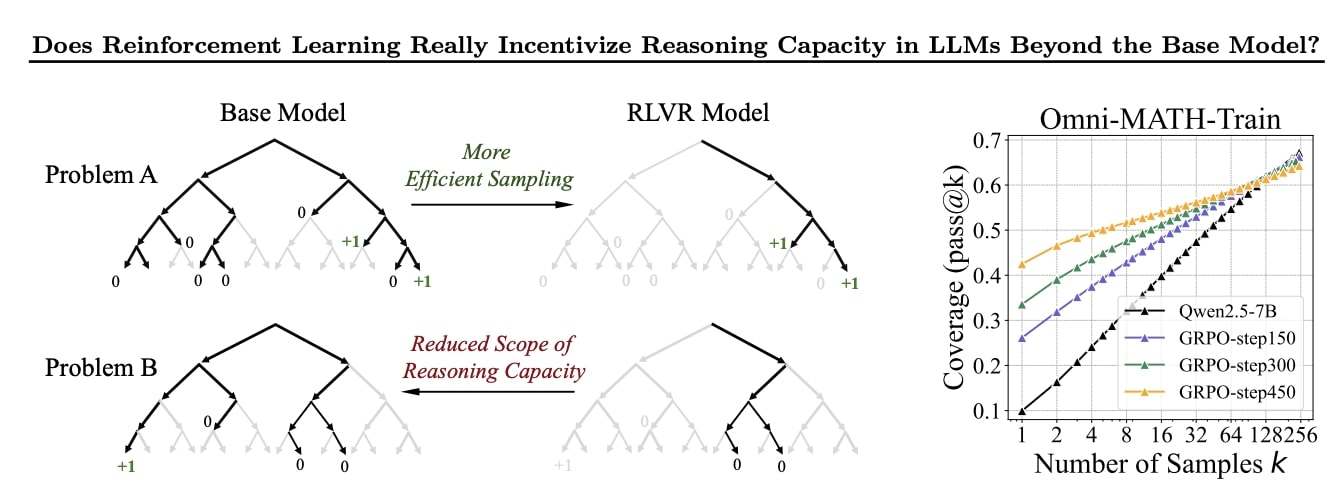
Thank you for visiting our website, your trusted source for the latest updates and in-depth coverage on Limitations Of Reinforcement Learning In Boosting AI Performance. We're committed to keeping you informed with timely and accurate information to meet your curiosity and needs.
If you have any questions, suggestions, or feedback, we'd love to hear from you. Your insights are valuable to us and help us improve to serve you better. Feel free to reach out through our contact page.
Don't forget to bookmark our website and check back regularly for the latest headlines and trending topics. See you next time, and thank you for being part of our growing community!
Featured Posts
-
Election 2025 A Deep Dive Into Public Housing Policies
Apr 29, 2025 -
Cardano Ada Price Analysis Crucial Support Level Under Pressure
Apr 29, 2025 -
Download Flappy Bird On Android Exclusive Epic Games Store Launch
Apr 29, 2025 -
Adatas High Speed Memory Card Performance Gains Tied To Compatible Reader
Apr 29, 2025 -
Tactical Breakdown Arsenal Vs Psg And Barcelona Vs Inter Milan Semi Finals
Apr 29, 2025
Latest Posts
-
Magnetic Mouse And Folding Usb C Cable A Users Perspective
Apr 30, 2025 -
Assessing Epics Mobile Games Store A Year In Review
Apr 30, 2025 -
Trump Complained To Bezos About Critical Amazon Report Media Outlets Report
Apr 30, 2025 -
Arsenal Psg Rematch Luis Enriques Call For A Historic Turnaround
Apr 30, 2025 -
Doges Rise A Privacy Nightmare For Public Institutions
Apr 30, 2025