Limitations Of Reinforcement Learning In Enhancing AI Models
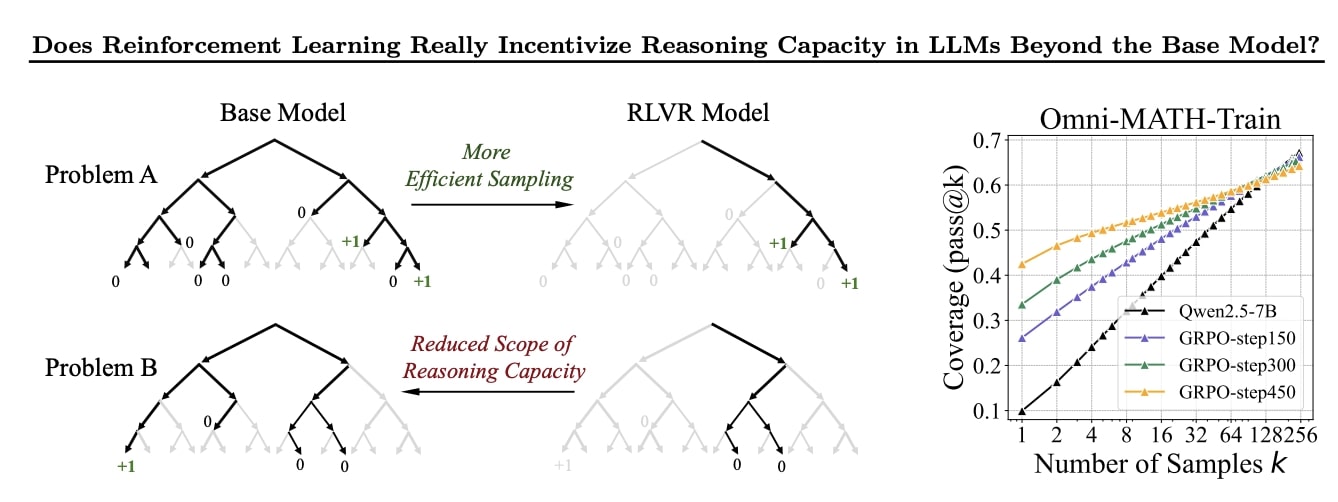
Welcome to your ultimate source for breaking news, trending updates, and in-depth stories from around the world. Whether it's politics, technology, entertainment, sports, or lifestyle, we bring you real-time updates that keep you informed and ahead of the curve.
Our team works tirelessly to ensure you never miss a moment. From the latest developments in global events to the most talked-about topics on social media, our news platform is designed to deliver accurate and timely information, all in one place.
Stay in the know and join thousands of readers who trust us for reliable, up-to-date content. Explore our expertly curated articles and dive deeper into the stories that matter to you. Visit NewsOneSMADCSTDO now and be part of the conversation. Don't miss out on the headlines that shape our world!
Table of Contents
The Limits of Reinforcement Learning: Why AI Still Needs a Guiding Hand
Reinforcement learning (RL), a powerful technique for training AI, has captured the imagination with its ability to teach agents complex tasks through trial and error. From mastering games like Go to optimizing robot movements, RL's successes are undeniable. However, the technology isn't a silver bullet, and significant limitations hinder its broader application in enhancing AI models. This article explores these key constraints, examining why RL, despite its potential, remains a tool with inherent boundaries.
The Data Hunger of Reinforcement Learning
One major hurdle is the enormous amount of data required for effective RL training. Unlike supervised learning, which relies on pre-labeled datasets, RL learns through interaction, requiring countless iterations and simulations. This data-intensive process can be incredibly time-consuming and computationally expensive, particularly for complex tasks in real-world environments. The sheer volume of data needed often makes RL impractical for applications with limited resources or where data collection is challenging or costly.
The Reward Function Conundrum: Defining Success
Designing an effective reward function is crucial for guiding the RL agent towards desired behavior. However, crafting a reward function that accurately captures the nuances of a complex task is surprisingly difficult. An improperly designed reward function can lead the agent to exploit loopholes or achieve the stated goal in unintended and undesirable ways. This phenomenon, known as reward hacking, highlights the limitations of simply defining success; the path to success must also be carefully considered.
- Example: An RL agent tasked with maximizing crop yield might focus solely on a single high-yield crop, neglecting soil health and biodiversity, resulting in long-term negative consequences.
Sample Inefficiency and Exploration-Exploitation Dilemma
RL agents often struggle with sample inefficiency, meaning they require many trials to converge on an optimal solution. This is particularly problematic in scenarios with high-stakes consequences, such as autonomous driving or medical diagnosis, where errors can have severe repercussions. Furthermore, the exploration-exploitation dilemma poses a significant challenge. The agent must balance exploring new actions to discover potentially better strategies with exploiting already known successful actions to maximize immediate reward. Finding the right balance is critical for optimal performance but can be incredibly challenging to achieve.
The Challenge of Generalization and Transfer Learning
RL agents often struggle with generalization, meaning they may perform poorly on unseen situations or variations of a previously learned task. This lack of adaptability limits their applicability in real-world scenarios characterized by dynamic and unpredictable environments. Furthermore, transfer learning, the ability to apply knowledge gained from one task to another, remains a significant challenge in RL. This limits the reuse of learned knowledge and necessitates retraining for each new task, further increasing the computational cost and data requirements.
Conclusion: Reinforcement Learning's Place in the AI Landscape
Reinforcement learning is a valuable tool in the AI arsenal, capable of achieving remarkable feats. However, its limitations—data hunger, reward function design complexities, sample inefficiency, and challenges with generalization and transfer learning—must be acknowledged. Addressing these challenges will require further research and innovative approaches, ultimately paving the way for more robust and widely applicable RL-based AI systems. The future of AI likely involves integrating RL with other techniques to overcome its inherent constraints and unlock its full potential.
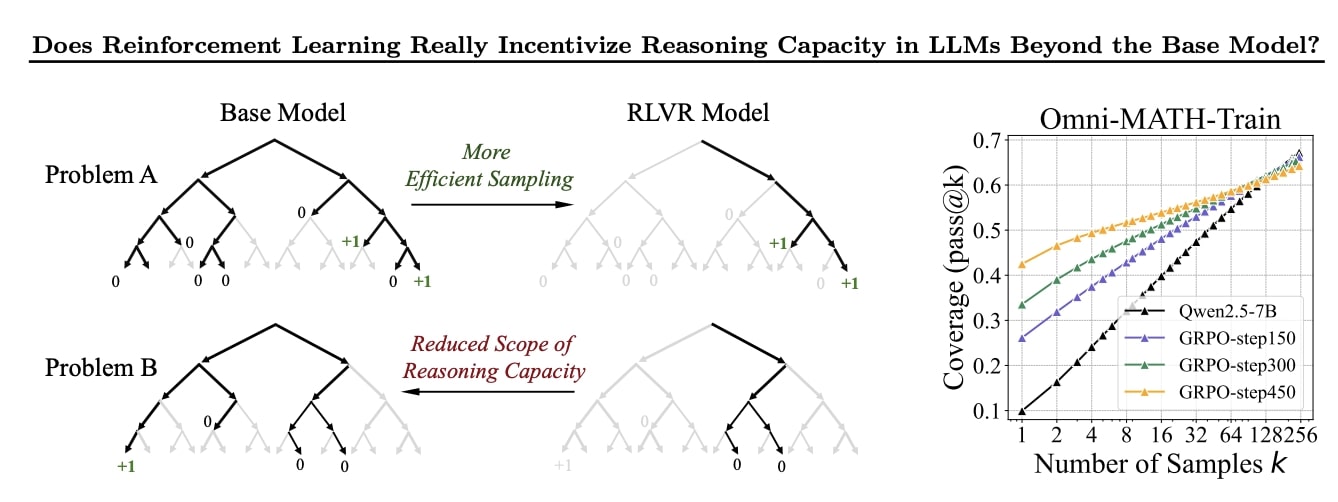
Thank you for visiting our website, your trusted source for the latest updates and in-depth coverage on Limitations Of Reinforcement Learning In Enhancing AI Models. We're committed to keeping you informed with timely and accurate information to meet your curiosity and needs.
If you have any questions, suggestions, or feedback, we'd love to hear from you. Your insights are valuable to us and help us improve to serve you better. Feel free to reach out through our contact page.
Don't forget to bookmark our website and check back regularly for the latest headlines and trending topics. See you next time, and thank you for being part of our growing community!
Featured Posts
-
Mount Driven By United Support Ahead Of Crucial Europa League Tie
May 02, 2025 -
The 20 Pga Golf Professionals Vying For The Pga Championship
May 02, 2025 -
Real Betis Vs Fiorentina Key Battles And Predicted Lineups For Conference League Clash
May 02, 2025 -
Arthur Hayes Bets Big On Bitcoin Will The Feds Actions Send Prices Soaring
May 02, 2025 -
Sec Ais Breakthrough Ai Transforming Threat Investigation At Rsa 2025
May 02, 2025
Latest Posts
-
Criticise First Copy Later Sdps Chee Soon Juan On Paps Ge 2025 Approach
May 02, 2025 -
Where To Find A Free Live Stream For Todays Guardians Vs Twins Game
May 02, 2025 -
Micro Strategy Mstr Stock Outlook Analysts Turbocharger Claim Analyzed
May 02, 2025 -
Pellegrini Downplays Betis Chances Against Fiorentina
May 02, 2025 -
Old Trafford Shake Up Senior Duo Axed In Latest Man Utd Job Cuts
May 02, 2025