Re-evaluating Reinforcement Learning's Impact On AI: A Sober Assessment
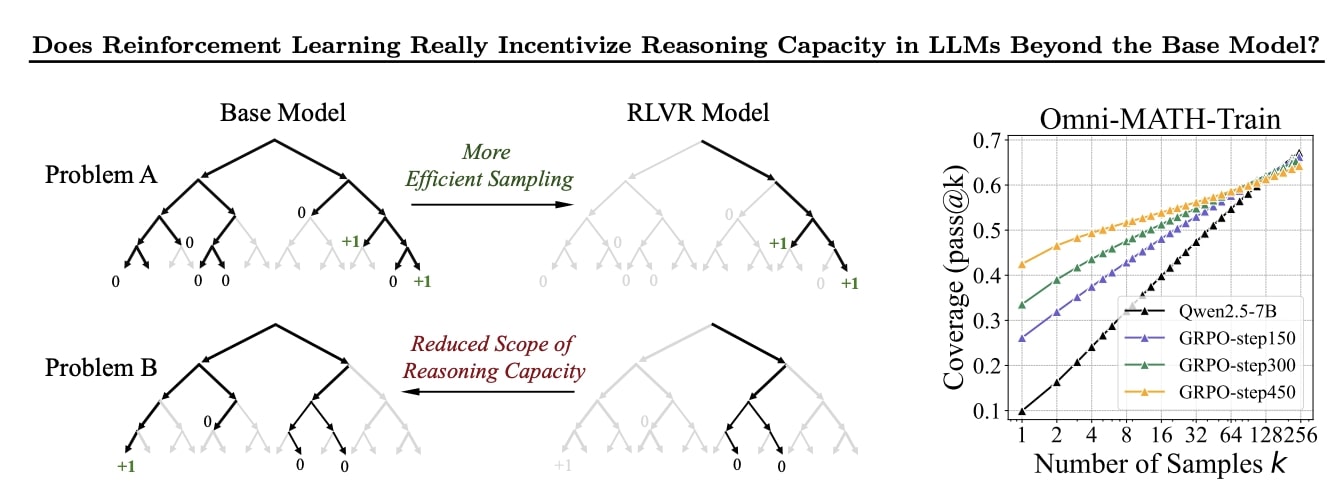
Welcome to your ultimate source for breaking news, trending updates, and in-depth stories from around the world. Whether it's politics, technology, entertainment, sports, or lifestyle, we bring you real-time updates that keep you informed and ahead of the curve.
Our team works tirelessly to ensure you never miss a moment. From the latest developments in global events to the most talked-about topics on social media, our news platform is designed to deliver accurate and timely information, all in one place.
Stay in the know and join thousands of readers who trust us for reliable, up-to-date content. Explore our expertly curated articles and dive deeper into the stories that matter to you. Visit NewsOneSMADCSTDO now and be part of the conversation. Don't miss out on the headlines that shape our world!
Table of Contents
Re-evaluating Reinforcement Learning's Impact on AI: A Sober Assessment
Reinforcement learning (RL), a powerful machine learning technique, has been hailed as a key driver of artificial intelligence advancements. From mastering complex games like Go to optimizing robotic control systems, RL's successes are undeniable. However, a more sober assessment reveals both its remarkable potential and significant limitations, necessitating a re-evaluation of its true impact on the broader AI landscape.
This article delves into the current state of RL, exploring its triumphs, challenges, and future directions. We’ll move beyond the hype to offer a balanced perspective on this crucial AI technology.
The Triumphs of Reinforcement Learning: Beyond the Game Board
RL's impact is evident across diverse fields. Its ability to learn optimal strategies through trial and error has yielded impressive results:
- Game Playing: DeepMind's AlphaGo famously defeated a world champion Go player, demonstrating RL's capacity to conquer games of immense complexity. This success showcased RL's potential for solving problems previously considered intractable.
- Robotics: RL algorithms are increasingly used to train robots to perform complex tasks, from manipulating objects to navigating challenging environments. This promises advancements in manufacturing, logistics, and even personal robotics.
- Resource Optimization: In areas like energy management and traffic control, RL algorithms can learn to optimize resource allocation, leading to increased efficiency and reduced costs. This has implications for sustainable development and smart cities.
- Personalized Medicine: RL shows promise in personalized medicine by learning optimal treatment strategies based on individual patient data. This could revolutionize healthcare by tailoring treatments to specific needs.
The Challenges and Limitations: A Realistic Perspective
Despite its successes, RL faces considerable challenges hindering its widespread adoption and full potential realization:
- Data Requirements: RL algorithms typically require massive amounts of data for training, often obtained through extensive simulations or real-world interactions. This can be computationally expensive and time-consuming.
- Sample Inefficiency: RL algorithms can be notoriously sample inefficient, meaning they require a large number of training iterations to achieve satisfactory performance. This limits their applicability in situations where data acquisition is costly or dangerous.
- Reward Function Design: Defining appropriate reward functions is crucial for effective RL training. Poorly designed reward functions can lead to unintended and undesirable behaviors, a phenomenon known as reward hacking.
- Safety and Explainability: Ensuring the safety and explainability of RL agents is paramount, particularly in high-stakes applications. Understanding why an RL agent makes a particular decision is crucial for building trust and mitigating risks.
- Generalization: RL agents often struggle to generalize their learned behaviors to new, unseen situations. Improving generalization capabilities is essential for creating more robust and adaptable AI systems.
The Future of Reinforcement Learning: Addressing the Challenges
The future of RL hinges on addressing its limitations. Active research focuses on:
- Improving sample efficiency: New algorithms and techniques are being developed to reduce the amount of data required for training.
- Developing safer and more explainable RL agents: Research is underway to create RL agents whose decisions are more transparent and understandable.
- Addressing the challenge of reward function design: Methods for automatically learning or optimizing reward functions are being explored.
- Enhancing generalization capabilities: Techniques like transfer learning and meta-learning are being applied to improve the ability of RL agents to adapt to new situations.
Conclusion: A Balanced View of RL's Impact
Reinforcement learning represents a powerful tool in the AI arsenal, capable of achieving remarkable results in diverse domains. However, its limitations must be acknowledged and addressed to fully unlock its transformative potential. Ongoing research and development are crucial for overcoming these challenges and realizing the true impact of RL on the future of artificial intelligence. A balanced perspective, acknowledging both the successes and the limitations, is essential for responsible innovation and the ethical development of this powerful technology.
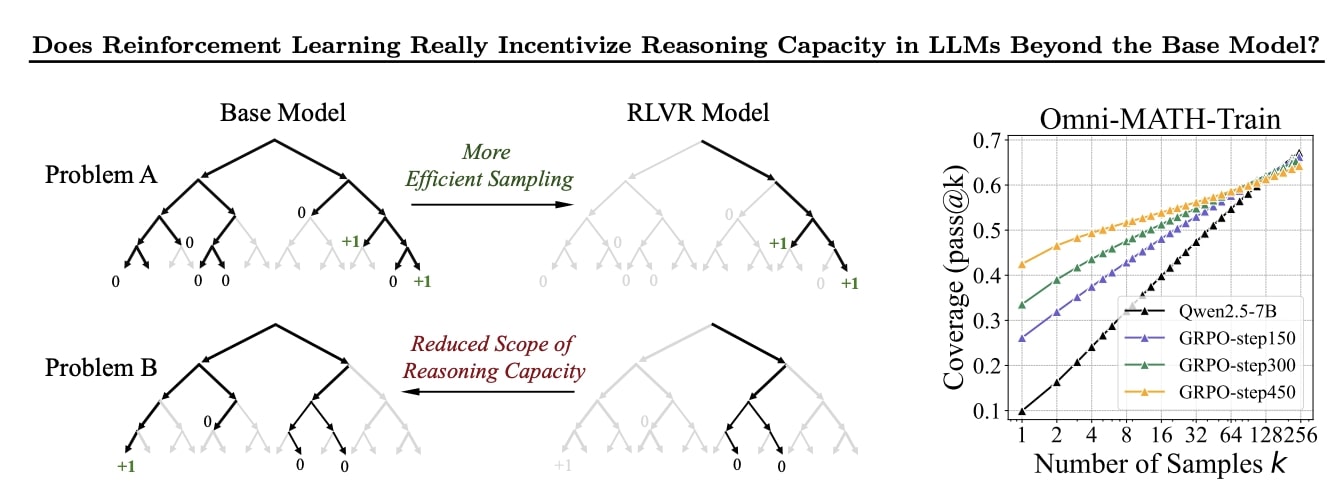
Thank you for visiting our website, your trusted source for the latest updates and in-depth coverage on Re-evaluating Reinforcement Learning's Impact On AI: A Sober Assessment. We're committed to keeping you informed with timely and accurate information to meet your curiosity and needs.
If you have any questions, suggestions, or feedback, we'd love to hear from you. Your insights are valuable to us and help us improve to serve you better. Feel free to reach out through our contact page.
Don't forget to bookmark our website and check back regularly for the latest headlines and trending topics. See you next time, and thank you for being part of our growing community!
Featured Posts
-
Garcia Haney Lopez Weigh In Results Nyc Fight Card Confirmed
May 03, 2025 -
Wwe Roster Shakeup Continues Predicting The Next Wave Of Releases
May 03, 2025 -
Steam Deck Vs Switch 2 Cd Projekt Red Endorses Nintendos Handheld For Cyberpunk 2077
May 03, 2025 -
Decentralization Redefining Eu Cloud Data Strategy And Security
May 03, 2025 -
Cerundolo Vs Ruud El Noruego Salva 15 Puntos De Rotura En Madrid
May 03, 2025
Latest Posts
-
Stablecoin Regulation The Impact Of The Us Genius Act
May 04, 2025 -
Three Avalanche Players Fuel Makar Led Game 6 Win
May 04, 2025 -
Playoffs On The Line Nuggets And Clippers Clash In Decisive Game 7
May 04, 2025 -
The Unabombers Brother A 27 Year Struggle For Reconciliation
May 04, 2025 -
Back On The Gold Coast Stephanie Gilmores Pursuit Of Surfing Success
May 04, 2025