Re-evaluating Reinforcement Learning's Impact On AI Performance
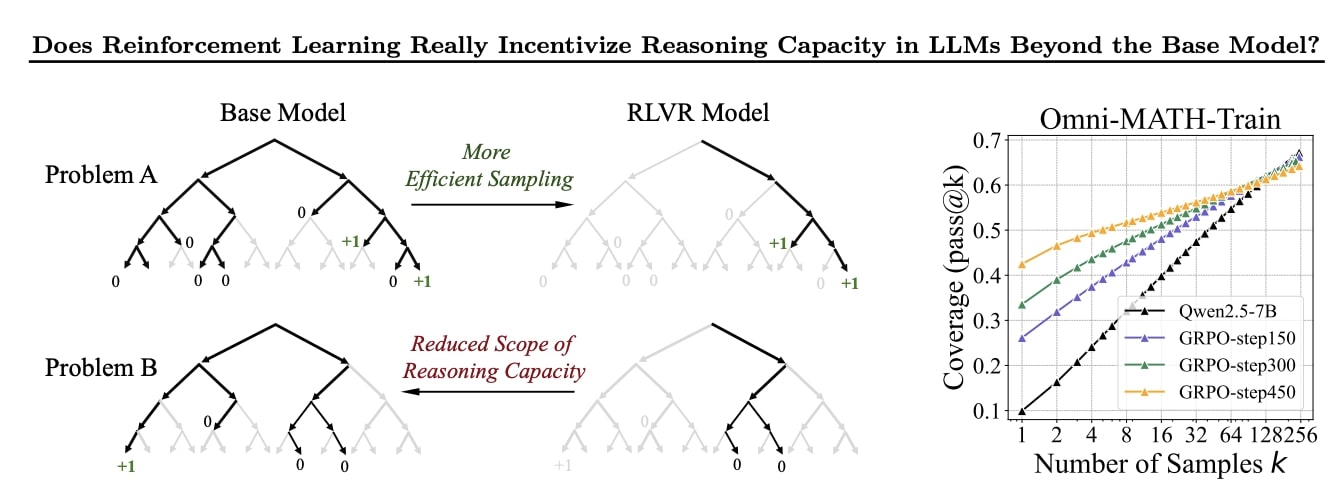
Welcome to your ultimate source for breaking news, trending updates, and in-depth stories from around the world. Whether it's politics, technology, entertainment, sports, or lifestyle, we bring you real-time updates that keep you informed and ahead of the curve.
Our team works tirelessly to ensure you never miss a moment. From the latest developments in global events to the most talked-about topics on social media, our news platform is designed to deliver accurate and timely information, all in one place.
Stay in the know and join thousands of readers who trust us for reliable, up-to-date content. Explore our expertly curated articles and dive deeper into the stories that matter to you. Visit NewsOneSMADCSTDO now and be part of the conversation. Don't miss out on the headlines that shape our world!
Table of Contents
Re-evaluating Reinforcement Learning's Impact on AI Performance: A Critical Look at Progress and Challenges
Reinforcement learning (RL), a powerful machine learning technique, has been hailed as a key driver of advancements in artificial intelligence. From mastering complex games like Go to optimizing robotic control systems, RL's ability to learn through trial and error has yielded impressive results. However, a closer examination reveals both the significant progress made and the persistent challenges that continue to hinder its widespread application and impact on overall AI performance. This article delves into a critical re-evaluation of RL's impact, exploring its successes, limitations, and the future directions of this transformative field.
H2: The Triumphs of Reinforcement Learning:
RL's success stories are undeniable. Deep reinforcement learning (DRL), combining RL with deep neural networks, has achieved superhuman performance in various domains:
- Game Playing: AlphaGo's victory over a world champion Go player marked a pivotal moment, showcasing RL's capacity to conquer games previously thought insurmountable for AI. Similar breakthroughs have been seen in chess, poker, and other complex strategic games.
- Robotics: RL algorithms are increasingly used to train robots for intricate tasks, from manipulating objects to navigating complex environments. This has significant implications for automation in manufacturing, logistics, and other industries.
- Resource Optimization: RL is being deployed to optimize resource allocation in areas like energy grids, traffic management, and telecommunications, leading to increased efficiency and cost savings.
H2: Limitations and Challenges Confronting Reinforcement Learning:
Despite these successes, several key limitations hinder RL's broader impact on AI performance:
- Data Efficiency: RL algorithms often require vast amounts of data and computational resources for training. This can be prohibitively expensive and time-consuming, especially in real-world applications where data collection is difficult or costly. This is particularly true in safety-critical applications where extensive simulations are necessary.
- Sample Inefficiency: Many RL algorithms suffer from sample inefficiency, meaning they require a large number of trials to learn effectively. This translates into longer training times and potentially higher costs.
- Reward Function Design: Defining an appropriate reward function is crucial for successful RL. Poorly designed reward functions can lead to unintended and undesirable behaviors, a problem known as reward hacking. This highlights the need for careful consideration and robust testing of reward functions.
- Generalization: RL agents often struggle to generalize their learned behavior to new situations or environments that differ from their training data. This lack of generalization limits their applicability in dynamic and unpredictable real-world settings.
- Explainability and Interpretability: The “black box” nature of many DRL algorithms makes it difficult to understand how they arrive at their decisions. This lack of transparency poses a significant challenge, particularly in safety-critical applications where understanding the reasoning behind an AI's actions is crucial.
H2: The Future of Reinforcement Learning and AI Performance:
Addressing these challenges is crucial for unlocking RL's full potential. Research is actively exploring several promising avenues:
- Improved Algorithms: Researchers are developing more efficient and sample-efficient RL algorithms that require less data and computational resources.
- Transfer Learning: Transfer learning techniques aim to leverage knowledge learned in one domain to improve performance in another, reducing the need for extensive training data.
- Safe RL: Research in safe RL focuses on developing methods that ensure the safety and reliability of RL agents, especially in real-world applications.
- Explainable RL (XRL): XRL aims to create more transparent and interpretable RL algorithms, allowing for better understanding of their decision-making processes.
In conclusion, while reinforcement learning has demonstrably advanced AI capabilities, its widespread impact is still limited by several key challenges. Overcoming these challenges through ongoing research and development will be essential to fully harness the transformative potential of RL and significantly boost overall AI performance across a wider range of applications. The future of AI likely hinges on continued progress in making RL more efficient, safe, and interpretable.
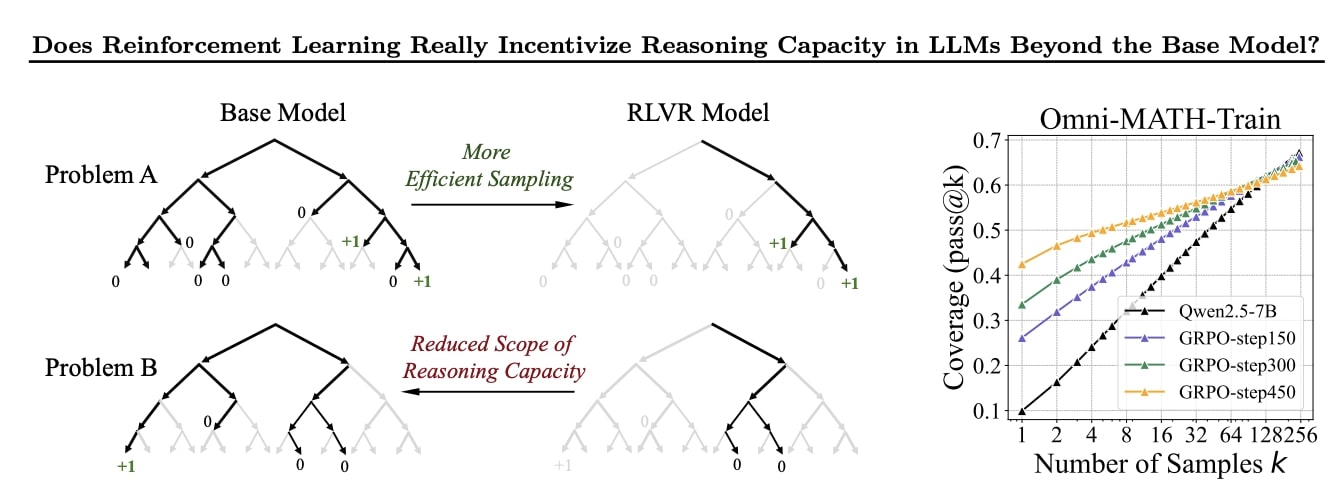
Thank you for visiting our website, your trusted source for the latest updates and in-depth coverage on Re-evaluating Reinforcement Learning's Impact On AI Performance. We're committed to keeping you informed with timely and accurate information to meet your curiosity and needs.
If you have any questions, suggestions, or feedback, we'd love to hear from you. Your insights are valuable to us and help us improve to serve you better. Feel free to reach out through our contact page.
Don't forget to bookmark our website and check back regularly for the latest headlines and trending topics. See you next time, and thank you for being part of our growing community!
Featured Posts
-
Beyond Paradise Relationship On The Rocks Couples Exit Confirmed By Bbc
May 03, 2025 -
Oklahoma And Arkansas Dominate College Softball Power 10 Rankings
May 03, 2025 -
Is Australias Vote A Reflection Of Worldwide Anti Trump Feeling
May 03, 2025 -
World Password Day 2025 The Latest News And Best Practices
May 03, 2025 -
Series Finale Heartbreak Phillies Win Streak Ends Against Nationals
May 03, 2025
Latest Posts
-
Australia Election Results A Key Indicator Of Worldwide Anti Trump Feeling
May 03, 2025 -
Unexpected Wwe Releases Strowman Kai And Others Let Go
May 03, 2025 -
Social Media Frenzy Oklahoma Takes Sec Championship
May 03, 2025 -
Sui Price Surges Analysts Predict 4 Target In Next Bull Run
May 03, 2025 -
Orbital Decline Of Otp 2 Propellantless Drive Satellite A Detailed Analysis
May 03, 2025