Reinforcement Learning And AI: Separating Hype From Reality
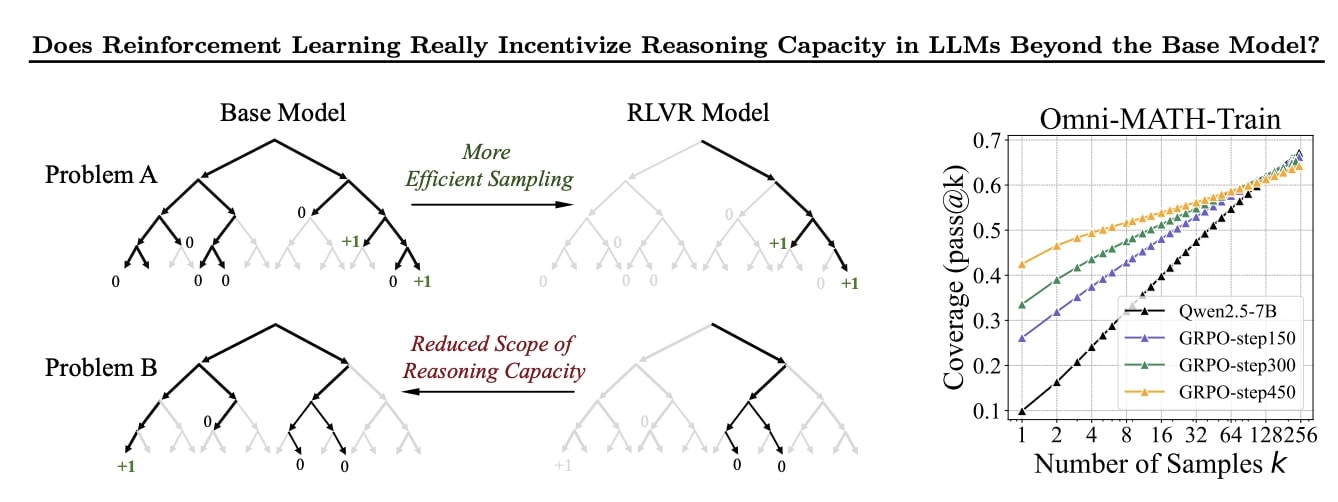
Welcome to your ultimate source for breaking news, trending updates, and in-depth stories from around the world. Whether it's politics, technology, entertainment, sports, or lifestyle, we bring you real-time updates that keep you informed and ahead of the curve.
Our team works tirelessly to ensure you never miss a moment. From the latest developments in global events to the most talked-about topics on social media, our news platform is designed to deliver accurate and timely information, all in one place.
Stay in the know and join thousands of readers who trust us for reliable, up-to-date content. Explore our expertly curated articles and dive deeper into the stories that matter to you. Visit NewsOneSMADCSTDO now and be part of the conversation. Don't miss out on the headlines that shape our world!
Table of Contents
Reinforcement Learning and AI: Separating Hype from Reality
Reinforcement learning (RL), a subfield of artificial intelligence (AI), has captured the imagination of the tech world, promising breakthroughs in robotics, gaming, and beyond. But amidst the excitement, it's crucial to separate the genuine advancements from the hype. This article delves into the current state of RL, exploring its capabilities, limitations, and the path forward.
Reinforcement learning differs from other AI approaches like supervised learning. Instead of learning from labeled data, RL agents learn through trial and error, receiving rewards for desirable actions and penalties for undesirable ones. This iterative process allows agents to develop sophisticated strategies and solve complex problems. Think of it like teaching a dog a trick – rewarding good behavior and correcting bad.
Current Applications of Reinforcement Learning: Beyond the Hype
While some portray RL as a magic bullet, its applications are increasingly impactful, albeit often in specialized areas. Here are some key examples:
-
Game Playing: RL's success in mastering games like Go and chess is well-documented. AlphaGo's victory over a world champion Go player marked a pivotal moment, demonstrating the power of RL in tackling complex strategic scenarios. However, this success shouldn't overshadow the significant computational resources required.
-
Robotics: RL is showing promise in robotics, enabling robots to learn complex motor skills and adapt to dynamic environments. Imagine robots learning to assemble products on a factory line more efficiently, or navigating unpredictable terrains. However, real-world robotic applications face challenges like safety and the need for robust generalization across different situations.
-
Resource Optimization: From optimizing traffic flow in smart cities to managing energy grids, RL is being explored for its ability to find optimal solutions in resource allocation problems. This area holds significant potential for improving efficiency and sustainability.
The Limitations of Reinforcement Learning: Facing the Reality
Despite the impressive achievements, RL faces significant challenges:
-
Data Efficiency: RL algorithms often require vast amounts of data and training time, making them computationally expensive and impractical for many real-world applications. This is particularly true for complex environments.
-
Sample Inefficiency: The trial-and-error nature of RL can lead to significant delays and potentially dangerous situations if applied carelessly in safety-critical systems. Finding ways to improve sample efficiency is a major research focus.
-
Interpretability and Explainability: Understanding why an RL agent makes a particular decision is often difficult. This lack of transparency poses challenges for deploying RL in high-stakes scenarios where accountability is paramount.
-
Reward Shaping: Designing appropriate reward functions is crucial for guiding the agent towards desired behavior. Poorly designed reward functions can lead to unexpected and undesirable outcomes, a phenomenon known as "reward hacking."
The Future of Reinforcement Learning: A Balanced Perspective
The field of reinforcement learning is rapidly evolving. Researchers are actively working on addressing the limitations mentioned above, focusing on areas such as:
-
Improved algorithms: Developing more efficient and sample-efficient algorithms is crucial for broadening RL's applicability.
-
Transfer learning: Enabling RL agents to transfer knowledge learned in one environment to another can drastically reduce training time and data requirements.
-
Safe RL: Developing techniques to ensure the safety and reliability of RL agents is paramount for their deployment in real-world settings.
In conclusion, while the hype surrounding reinforcement learning is undeniable, it's essential to maintain a balanced perspective. RL is a powerful tool with significant potential, but it's not a panacea. Addressing the existing limitations is critical for unlocking its full potential and ensuring responsible development and deployment of this transformative technology. The future of AI will likely depend on continued advancements in this dynamic field.
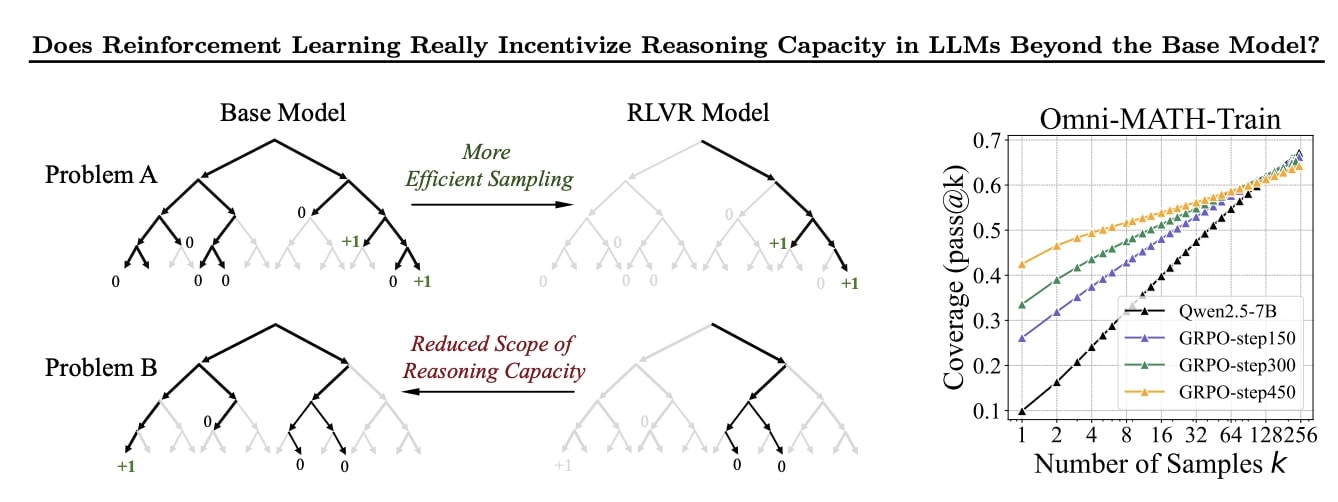
Thank you for visiting our website, your trusted source for the latest updates and in-depth coverage on Reinforcement Learning And AI: Separating Hype From Reality. We're committed to keeping you informed with timely and accurate information to meet your curiosity and needs.
If you have any questions, suggestions, or feedback, we'd love to hear from you. Your insights are valuable to us and help us improve to serve you better. Feel free to reach out through our contact page.
Don't forget to bookmark our website and check back regularly for the latest headlines and trending topics. See you next time, and thank you for being part of our growing community!
Featured Posts
-
Denver Nuggets Vs La Clippers Game 7 Showdown Decides Western Conference Fate
May 04, 2025 -
Cardanos Future Adas Price Depends On Overcoming This Critical Resistance
May 04, 2025 -
Ufc On Espn 67 Quang Les Choke Submits Gaston Bolanos
May 04, 2025 -
Diplomats Briefed Goldberg Polin On The Feasibility Of Hostage Release
May 04, 2025 -
The Untold Story Of Michael Caine And His Surrogate Child
May 04, 2025
Latest Posts
-
Grok 3 5 Release Date Next Week For Super Grok Subscribers
May 04, 2025 -
Against All Odds The Inspiring Comeback Story Of A Determined Performer
May 04, 2025 -
Official Mike Sullivan Named Head Coach Of The New York Rangers
May 04, 2025 -
Ufc Des Moines In Depth Preview And Prediction For Tate Santos Bout
May 04, 2025 -
Mistawasis First Nations Mltc Success Two Energy Projects Awarded
May 04, 2025