Reinforcement Learning In AI: Assessing Its Actual Contribution To Model Advancement
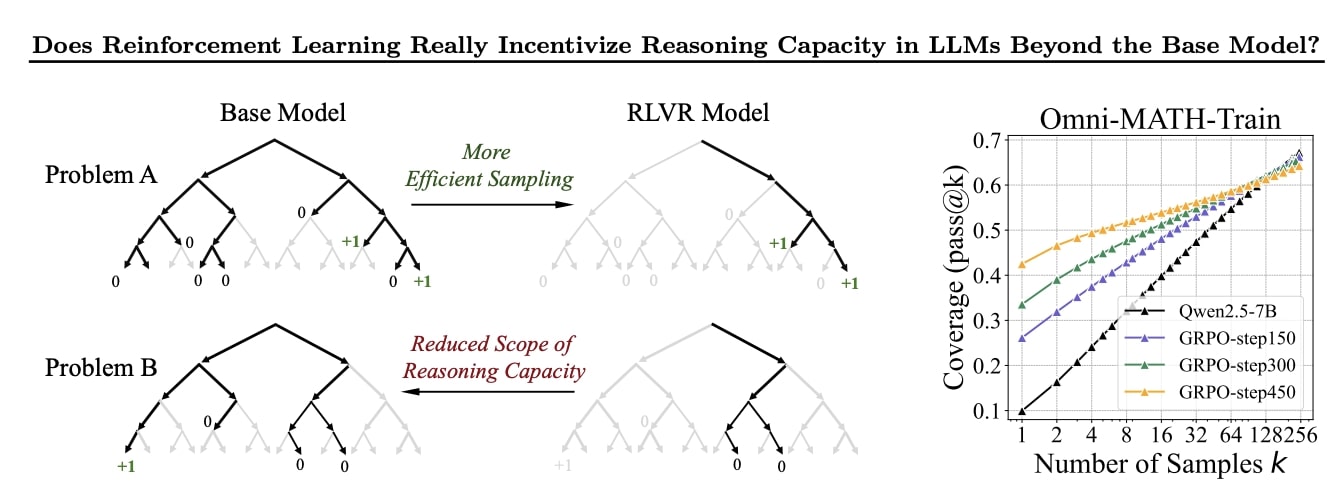
Welcome to your ultimate source for breaking news, trending updates, and in-depth stories from around the world. Whether it's politics, technology, entertainment, sports, or lifestyle, we bring you real-time updates that keep you informed and ahead of the curve.
Our team works tirelessly to ensure you never miss a moment. From the latest developments in global events to the most talked-about topics on social media, our news platform is designed to deliver accurate and timely information, all in one place.
Stay in the know and join thousands of readers who trust us for reliable, up-to-date content. Explore our expertly curated articles and dive deeper into the stories that matter to you. Visit NewsOneSMADCSTDO now and be part of the conversation. Don't miss out on the headlines that shape our world!
Table of Contents
Reinforcement Learning in AI: Assessing its Actual Contribution to Model Advancement
Reinforcement learning (RL), a subfield of machine learning, has generated significant buzz in recent years. Promising breakthroughs in complex problem-solving, from game playing to robotics, have led many to hail RL as a revolutionary force in artificial intelligence. But beyond the hype, what is the actual contribution of RL to overall model advancement? This article delves into the successes and limitations of RL, assessing its true impact on the AI landscape.
Reinforcement learning algorithms learn through trial and error. Unlike supervised learning, which relies on labeled data, RL agents interact with an environment, receiving rewards or penalties based on their actions. This iterative process allows the agent to optimize its behavior and achieve a specific goal. This approach has proven remarkably effective in certain domains.
H2: RL's Triumphs: Where it Shines Bright
-
Game Playing: DeepMind's AlphaGo, AlphaZero, and MuZero are prime examples of RL's success. These agents achieved superhuman performance in complex games like Go, chess, and shogi, demonstrating the power of RL to master intricate strategic environments. These victories, however, often rely on massive computational resources and carefully designed reward functions.
-
Robotics: RL is increasingly used to train robots for complex tasks, such as manipulation, locomotion, and navigation. RL algorithms can enable robots to adapt to unpredictable environments and learn new skills without explicit programming. This opens up exciting possibilities in areas like manufacturing, healthcare, and exploration.
-
Personalized Recommendations: RL can optimize recommendation systems by learning user preferences and predicting future behavior. By dynamically adjusting recommendations based on user interactions, RL can improve user engagement and satisfaction. This application highlights the potential of RL in personalized experiences across various platforms.
H2: The Challenges and Limitations of RL
Despite its successes, RL faces significant challenges that limit its broader applicability:
-
Sample Inefficiency: RL algorithms often require vast amounts of data and interactions with the environment to learn effectively. This can be computationally expensive and time-consuming, especially in real-world scenarios where data collection can be difficult or costly.
-
Reward Design: Defining appropriate reward functions is crucial for successful RL. Poorly designed rewards can lead to unintended consequences and suboptimal behavior. Creating robust and reliable reward functions remains a significant challenge, particularly in complex and multifaceted environments.
-
Safety and Generalization: Ensuring the safety and reliability of RL agents is critical, especially in applications with real-world consequences. RL agents may exhibit unpredictable or unsafe behavior in unfamiliar situations, highlighting the need for robust generalization and safety mechanisms. Explainability of RL models also remains a significant hurdle, hindering broader adoption in sensitive domains.
-
Interpretability and Explainability: Understanding why an RL agent makes a particular decision is often difficult. This lack of transparency can limit the trustworthiness and acceptance of RL systems, especially in high-stakes applications. This is an active area of research, with researchers exploring techniques to improve the interpretability of RL models.
H2: The Future of RL and its Contribution to AI Advancement
Despite its limitations, RL remains a vital area of research and development in AI. Ongoing research focuses on addressing the challenges outlined above, including developing more sample-efficient algorithms, improving reward function design, enhancing safety and robustness, and increasing interpretability. Furthermore, hybrid approaches that combine RL with other machine learning techniques, such as supervised learning and imitation learning, hold significant promise.
In conclusion, while reinforcement learning has undeniably contributed to significant advancements in AI, particularly in specific domains like game playing and robotics, its broader impact is still developing. Addressing the challenges related to sample efficiency, reward design, safety, and interpretability is crucial for unlocking RL's full potential and realizing its transformative promise across a wider range of applications. The future of AI likely hinges on integrating and optimizing diverse learning paradigms, with RL playing a crucial, albeit nuanced, role.
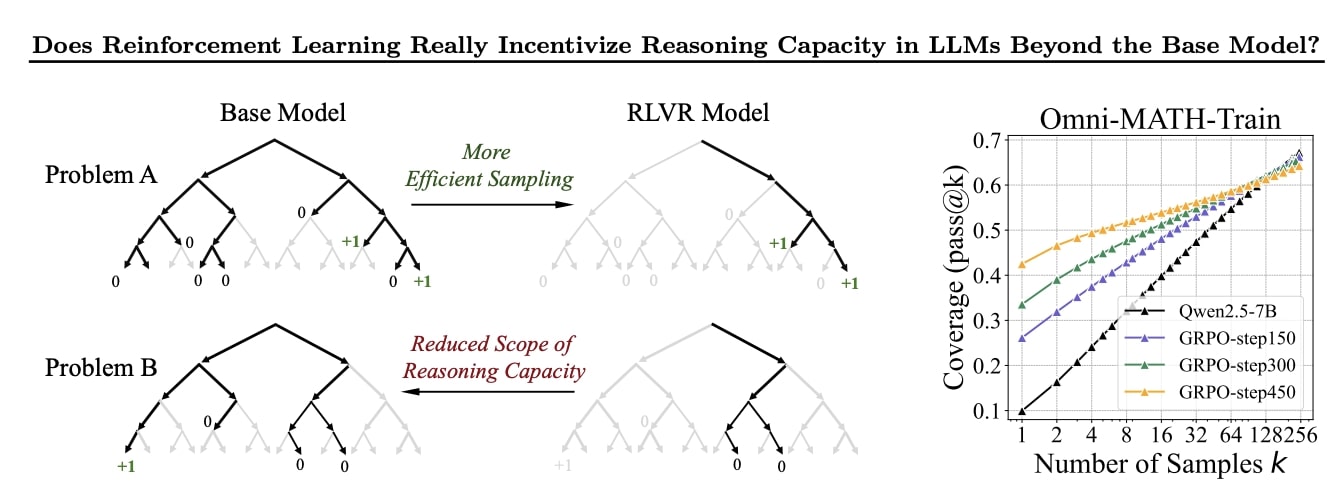
Thank you for visiting our website, your trusted source for the latest updates and in-depth coverage on Reinforcement Learning In AI: Assessing Its Actual Contribution To Model Advancement. We're committed to keeping you informed with timely and accurate information to meet your curiosity and needs.
If you have any questions, suggestions, or feedback, we'd love to hear from you. Your insights are valuable to us and help us improve to serve you better. Feel free to reach out through our contact page.
Don't forget to bookmark our website and check back regularly for the latest headlines and trending topics. See you next time, and thank you for being part of our growing community!
Featured Posts
-
Thunderbolts Director Reveals Hiroshima Influence On Voids Visuals
May 02, 2025 -
Electric Pickup Trucks The Next Big Ev Fight First Slate Auto And Isuzu Lead The Charge
May 02, 2025 -
Silence Before The Storm Navigating The Ge 2025 Cooling Off Period
May 02, 2025 -
Live Australias 2025 Federal Election Final Predictions And Voter Turnout
May 02, 2025 -
Fact Check Is Donald Trump Mistaking Calibri For Ms 13 Gang Tattoos
May 02, 2025