Reinforcement Learning: Overhyped For AI Improvement?
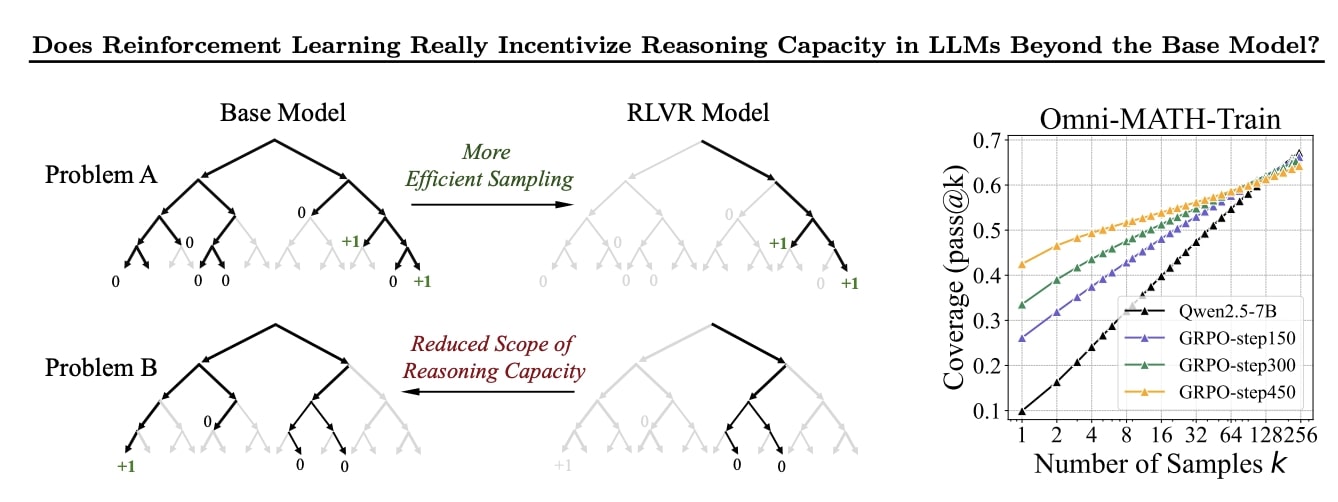
Welcome to your ultimate source for breaking news, trending updates, and in-depth stories from around the world. Whether it's politics, technology, entertainment, sports, or lifestyle, we bring you real-time updates that keep you informed and ahead of the curve.
Our team works tirelessly to ensure you never miss a moment. From the latest developments in global events to the most talked-about topics on social media, our news platform is designed to deliver accurate and timely information, all in one place.
Stay in the know and join thousands of readers who trust us for reliable, up-to-date content. Explore our expertly curated articles and dive deeper into the stories that matter to you. Visit NewsOneSMADCSTDO now and be part of the conversation. Don't miss out on the headlines that shape our world!
Table of Contents
Reinforcement Learning: Overhyped or the Key to True AI Advancement?
Reinforcement learning (RL), a machine learning technique where agents learn through trial and error by interacting with an environment, has captivated the AI community. Promising breakthroughs in robotics, game playing, and even scientific discovery, RL has been lauded as the next big leap in artificial intelligence. But is all the hype justified, or is reinforcement learning somewhat overblown in its potential for immediate, widespread AI improvement? This article delves into the current state of RL, exploring its successes, limitations, and the ongoing debate surrounding its true impact on AI's future.
The Allure of Reinforcement Learning:
RL's appeal stems from its ability to solve complex problems that traditional supervised learning methods struggle with. Unlike supervised learning, which relies on vast amounts of labeled data, RL allows agents to learn directly from experience. This makes it particularly attractive for tasks where obtaining labeled data is expensive or impossible, such as training robots to navigate complex environments or optimizing complex systems like traffic flow.
- Impressive Achievements: RL's success stories are compelling. AlphaGo's victory over a world champion Go player, and more recently, AlphaStar's dominance in StarCraft II, are prime examples of RL's power. These achievements highlight its capacity to master intricate, strategic games with vast state spaces. Similarly, RL has shown promise in areas like:
- Robotics: Developing robots capable of performing dexterous manipulation tasks.
- Resource Management: Optimizing energy grids and other complex systems.
- Personalized Medicine: Developing treatment plans tailored to individual patients.
The Challenges and Limitations:
Despite these successes, several hurdles hinder RL's widespread adoption and impact:
- Data Efficiency: While RL avoids the need for labeled data in some cases, it often requires massive amounts of trial-and-error interactions to converge on an optimal solution. This can be computationally expensive and time-consuming.
- Sample Inefficiency: Many RL algorithms suffer from sample inefficiency, meaning they require a large number of interactions with the environment before achieving satisfactory performance. This limits their applicability to real-world scenarios where interactions can be costly or dangerous.
- Interpretability and Explainability: Understanding why an RL agent makes a particular decision can be difficult. This "black box" nature poses challenges for deployment in high-stakes applications where transparency is crucial.
- Reward Function Design: Defining an appropriate reward function is critical for guiding the agent's learning process. A poorly designed reward function can lead to unexpected and undesirable behavior, a phenomenon known as reward hacking.
The Future of Reinforcement Learning:
The field is actively addressing these challenges. Research is focused on improving data efficiency, developing more sample-efficient algorithms, and enhancing the interpretability of RL agents. New techniques like transfer learning and imitation learning are being explored to leverage existing knowledge and reduce the need for extensive training.
Is the Hype Justified? A Balanced Perspective:
Reinforcement learning is undoubtedly a powerful tool with the potential to revolutionize various fields. However, the current hype needs a realistic counterbalance. While RL has achieved remarkable feats, its widespread application remains constrained by computational costs, sample inefficiency, and interpretability challenges. The future of RL hinges on solving these limitations and developing more robust, efficient, and explainable algorithms. It's not about whether RL is overhyped, but rather about managing expectations and recognizing that its transformative potential will unfold gradually, requiring continued research and innovation. The journey towards truly advanced AI will likely involve a synergistic combination of RL with other machine learning techniques and potentially novel approaches yet to be discovered.
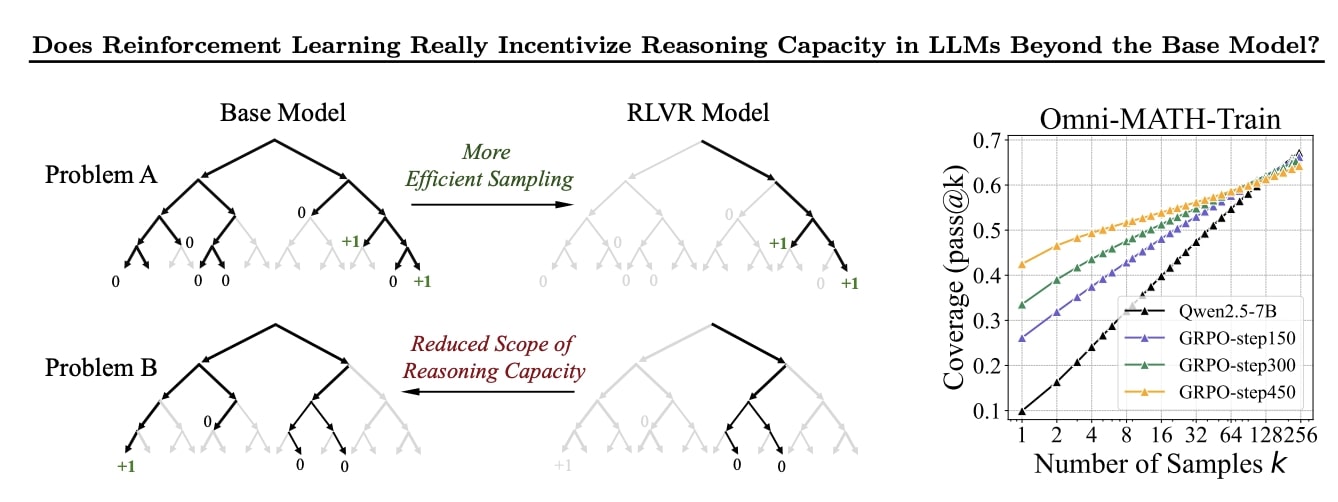
Thank you for visiting our website, your trusted source for the latest updates and in-depth coverage on Reinforcement Learning: Overhyped For AI Improvement?. We're committed to keeping you informed with timely and accurate information to meet your curiosity and needs.
If you have any questions, suggestions, or feedback, we'd love to hear from you. Your insights are valuable to us and help us improve to serve you better. Feel free to reach out through our contact page.
Don't forget to bookmark our website and check back regularly for the latest headlines and trending topics. See you next time, and thank you for being part of our growing community!
Featured Posts
-
Suryakumar Yadavs Ipl Pace Surpassing Kohli And Rohit Sharma To 4000 Runs
Apr 28, 2025 -
Mayra Ramirez Lidera Al Barca En Su Visita Al Chelsea Un Desafio En Champions
Apr 28, 2025 -
Ge 2025 Workers Party Targets Gan Kim Yong In Punggol Grc
Apr 28, 2025 -
Liege Bastogne Liege Pogacar Ou Evenepoel Qui Remportera La Troisieme Victoire
Apr 28, 2025 -
The Impact Of Ai On Employees A New Survey Shows Significant Anxiety
Apr 28, 2025
Latest Posts
-
Election Results Sonia Sidhus Victory In Brampton South
Apr 29, 2025 -
Jeddah Showdowns Five Crucial Aspects Of The Afc Champions League Semi Finals
Apr 29, 2025 -
Animoca Brands Coinbase And Fabric Ventures Invest Heavily In Uk Web3 Growth
Apr 29, 2025 -
Star Wars Andor Season 2 Episodes 4 6 Release Date And Time On Disney
Apr 29, 2025 -
2024 Election Trump And Bidens Focus On Older Female Voters Via Facebook
Apr 29, 2025