Reinforcement Learning: Overhyped For AI Model Improvement?
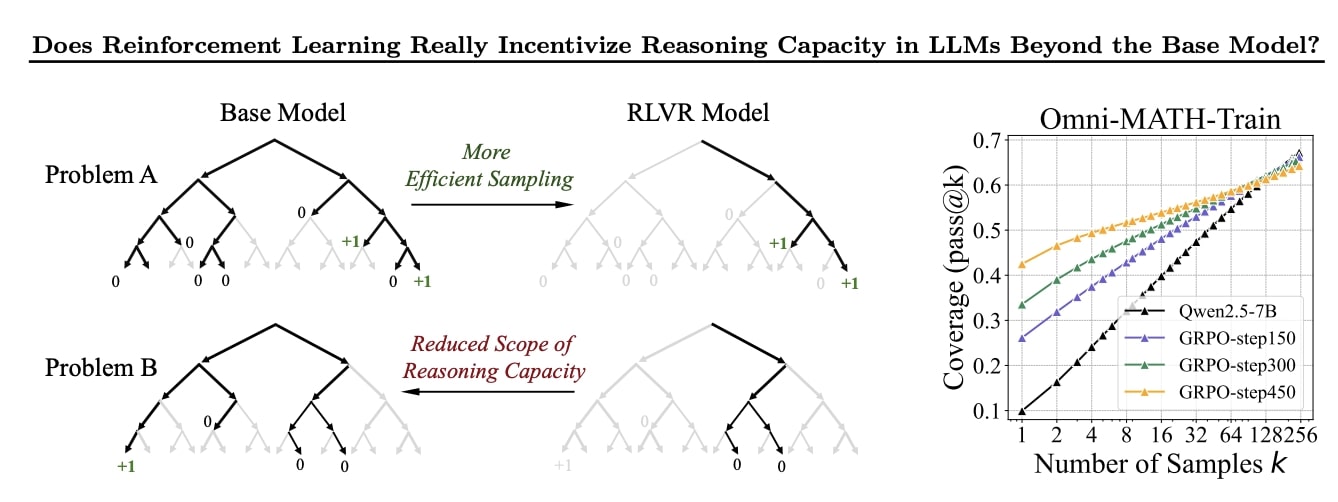
Welcome to your ultimate source for breaking news, trending updates, and in-depth stories from around the world. Whether it's politics, technology, entertainment, sports, or lifestyle, we bring you real-time updates that keep you informed and ahead of the curve.
Our team works tirelessly to ensure you never miss a moment. From the latest developments in global events to the most talked-about topics on social media, our news platform is designed to deliver accurate and timely information, all in one place.
Stay in the know and join thousands of readers who trust us for reliable, up-to-date content. Explore our expertly curated articles and dive deeper into the stories that matter to you. Visit NewsOneSMADCSTDO now and be part of the conversation. Don't miss out on the headlines that shape our world!
Table of Contents
Reinforcement Learning: Overhyped for AI Model Improvement?
Reinforcement learning (RL), a powerful machine learning technique, has garnered significant attention as a potential game-changer for improving AI models. But is all the hype justified? While RL offers exciting possibilities, a closer look reveals both its remarkable strengths and its significant limitations, prompting the question: is RL currently overhyped for AI model improvement?
The Allure of Reinforcement Learning:
RL's appeal stems from its ability to train agents to achieve complex goals in dynamic environments. Unlike supervised learning, which relies on labeled datasets, RL agents learn through trial and error, receiving rewards for desirable actions and penalties for undesirable ones. This iterative process allows agents to master intricate tasks, from playing games like Go and chess to controlling robots and optimizing complex systems. This capacity for adaptation and problem-solving in unpredictable scenarios makes RL a compelling approach for various AI applications.
Real-World Applications Demonstrating RL's Power:
The success stories of RL are undeniable. Consider these examples:
- Robotics: RL algorithms have enabled robots to perform intricate tasks like assembling products, navigating complex environments, and even mastering delicate manipulation techniques.
- Game Playing: DeepMind's AlphaGo, trained using RL, famously defeated a world champion Go player, showcasing RL's potential in tackling complex strategic games.
- Resource Management: RL is being explored to optimize energy grids, manage traffic flow, and improve resource allocation in various industries.
- Personalized Recommendations: RL algorithms can personalize user experiences by learning individual preferences and adapting recommendations accordingly.
The Challenges and Limitations of RL:
Despite its successes, RL faces significant hurdles that limit its widespread adoption for general AI model improvement:
- Data Efficiency: RL algorithms typically require vast amounts of data and extensive training time, which can be computationally expensive and time-consuming. This makes RL less practical for applications with limited data availability.
- Reward Function Design: Defining a suitable reward function is crucial for RL's success. An improperly designed reward function can lead to unintended and undesirable behavior from the agent, a problem known as reward hacking.
- Sample Inefficiency: RL agents often struggle with sample inefficiency, meaning they require a large number of interactions with the environment to learn effectively. This can be particularly problematic in real-world scenarios where interactions are costly or risky.
- Interpretability and Explainability: Understanding why an RL agent makes a particular decision can be challenging, hindering its adoption in applications requiring transparency and accountability.
Is the Hype Justified? A Balanced Perspective:
Reinforcement learning is a powerful tool with the potential to significantly advance AI. However, its current limitations, particularly regarding data efficiency, reward function design, and sample inefficiency, suggest that the hype surrounding its immediate transformative impact might be somewhat inflated. While RL excels in specific domains, its broader application for general AI model improvement remains a significant challenge.
The Future of Reinforcement Learning:
Ongoing research focuses on addressing the limitations of RL, including developing more sample-efficient algorithms, improving reward function design techniques, and enhancing the interpretability of RL models. As these challenges are tackled, RL's potential to revolutionize various aspects of AI will undoubtedly become more realized. However, a more nuanced and realistic understanding of its current capabilities is crucial to avoid overselling its immediate transformative power. The future of RL is bright, but we should temper our expectations with a dose of pragmatic realism.
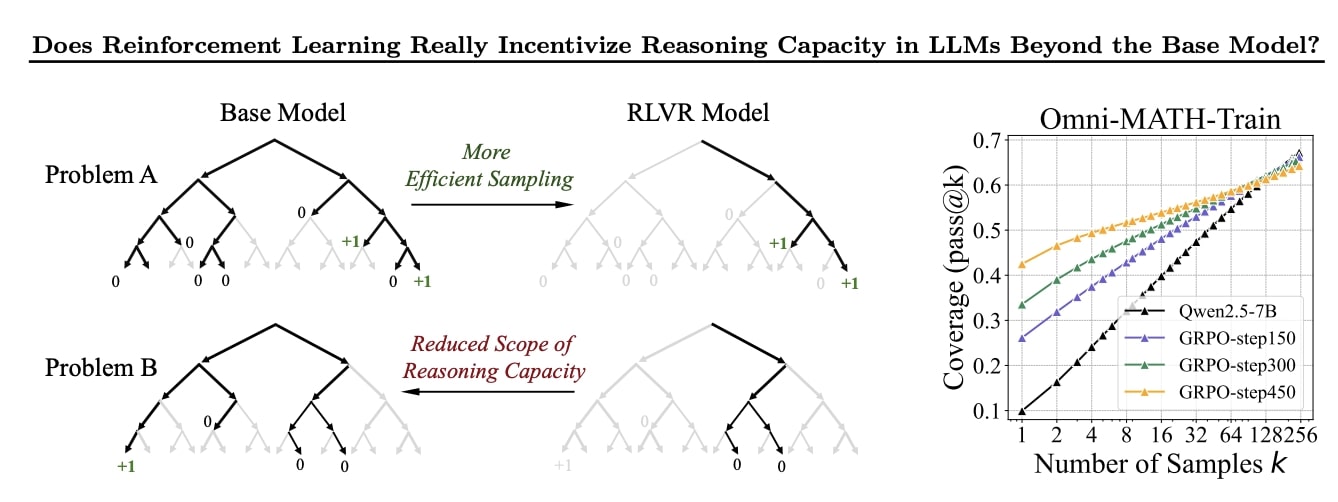
Thank you for visiting our website, your trusted source for the latest updates and in-depth coverage on Reinforcement Learning: Overhyped For AI Model Improvement?. We're committed to keeping you informed with timely and accurate information to meet your curiosity and needs.
If you have any questions, suggestions, or feedback, we'd love to hear from you. Your insights are valuable to us and help us improve to serve you better. Feel free to reach out through our contact page.
Don't forget to bookmark our website and check back regularly for the latest headlines and trending topics. See you next time, and thank you for being part of our growing community!
Featured Posts
-
From Unknown To Known The Transformative Power Of Complete Mars Mapping
Apr 29, 2025 -
Afghanistans Karim Janat Makes Ipl Debut Reaching Historic Landmark
Apr 29, 2025 -
Alleged Dji Mavic 4 Pro Images Surface Detailing Premium Design
Apr 29, 2025 -
Warren Buffett Y Apple La Venta Que Reduce Su Participacion En Un 13
Apr 29, 2025 -
Dicas Para Escolher A Melhor Cota De Casa Na Praia Ou No Campo Analise De Custos E Beneficios
Apr 29, 2025
Latest Posts
-
Honeyjoon What To Expect From The Azores Shot Film At Tribeca
Apr 30, 2025 -
Flappy Bird Returns To Mobile Exclusive Android Release On Epic Games Store
Apr 30, 2025 -
Update Case Closed No Charges In Death Of Former Nhl Player Adam Johnson
Apr 30, 2025 -
Follow Dc Vs Kkr Live Cricket Score Ball By Ball Updates
Apr 30, 2025 -
The Smashing Machine Trailer Dwayne Johnson As Ufc Legend Mark Kerr
Apr 30, 2025