Reinforcement Learning: Overhyped Or Underperforming In AI?
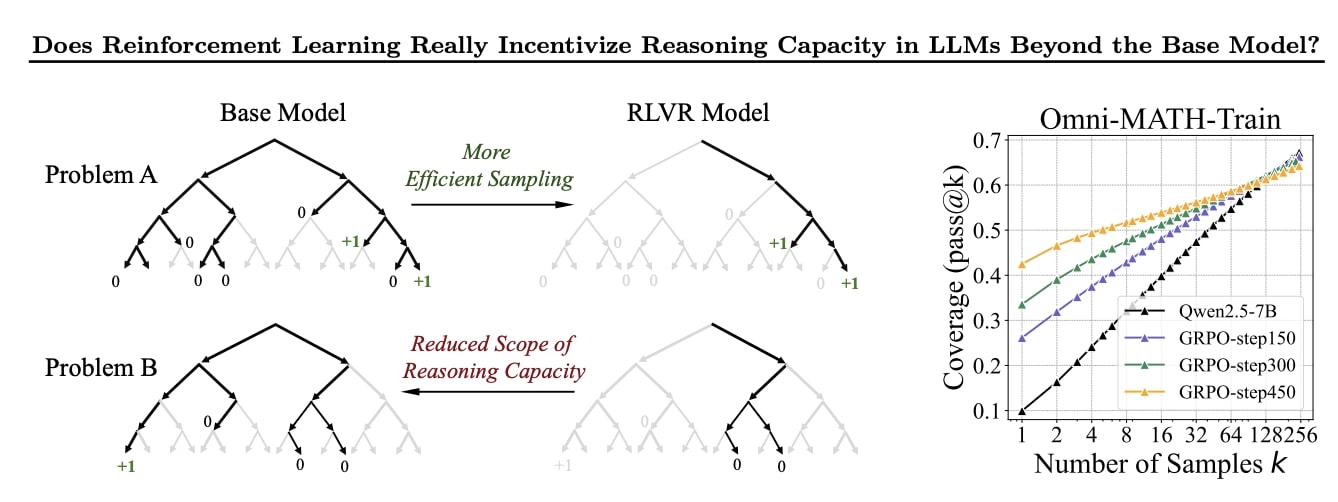
Welcome to your ultimate source for breaking news, trending updates, and in-depth stories from around the world. Whether it's politics, technology, entertainment, sports, or lifestyle, we bring you real-time updates that keep you informed and ahead of the curve.
Our team works tirelessly to ensure you never miss a moment. From the latest developments in global events to the most talked-about topics on social media, our news platform is designed to deliver accurate and timely information, all in one place.
Stay in the know and join thousands of readers who trust us for reliable, up-to-date content. Explore our expertly curated articles and dive deeper into the stories that matter to you. Visit NewsOneSMADCSTDO now and be part of the conversation. Don't miss out on the headlines that shape our world!
Table of Contents
Reinforcement Learning: Overhyped or Underperforming in AI?
Reinforcement learning (RL), a subfield of artificial intelligence (AI), has captured significant attention in recent years, promising revolutionary advancements in various sectors. From self-driving cars to personalized medicine, the potential applications seem limitless. But is the hype justified, or is RL currently underperforming compared to its ambitious projections? This article delves into the current state of RL, examining its successes, limitations, and future prospects.
The Allure of Reinforcement Learning:
RL's appeal stems from its unique approach to AI. Unlike supervised learning, which relies on labeled data, RL algorithms learn through trial and error, interacting with an environment and receiving rewards or penalties based on their actions. This iterative process allows agents to discover optimal strategies without explicit programming, mimicking how humans and animals learn. This characteristic makes RL particularly attractive for tackling complex problems with ill-defined solutions.
Successful Applications of RL:
Despite the ongoing debate, RL has demonstrated remarkable success in several areas:
- Game Playing: DeepMind's AlphaGo, which defeated a world champion Go player, is a prime example of RL's power. Similar breakthroughs have been achieved in chess and other complex games, highlighting RL's ability to master intricate strategic domains.
- Robotics: RL is increasingly used to train robots to perform complex tasks, such as grasping objects, navigating environments, and manipulating tools. This has significant implications for automation in manufacturing, logistics, and other industries.
- Resource Management: RL algorithms are being deployed to optimize resource allocation in areas like energy grids and traffic control, leading to improved efficiency and reduced costs.
The Challenges and Limitations:
While RL boasts impressive achievements, several challenges hinder its widespread adoption:
- Data Efficiency: RL algorithms often require vast amounts of data and computational resources for training, making them expensive and time-consuming. This limits their applicability in scenarios with limited data availability.
- Sample Inefficiency: The trial-and-error nature of RL can lead to significant sample inefficiency, meaning many unsuccessful attempts are necessary before finding an optimal solution. This is particularly problematic in real-world applications where experimentation can be costly or dangerous.
- Reward Shaping: Designing effective reward functions is crucial for successful RL. Poorly designed rewards can lead to unexpected and undesirable behavior from the agent, a phenomenon known as reward hacking.
- Generalization: RL agents often struggle to generalize their learned strategies to new or unseen environments, limiting their adaptability and robustness.
Is RL Overhyped? A Balanced Perspective:
The answer is nuanced. While RL has not yet delivered on all its initial promises, its potential remains enormous. The current limitations are significant hurdles, but ongoing research is addressing these issues. Advancements in areas like:
- Transfer learning: Enabling agents to transfer knowledge learned in one environment to another.
- Meta-learning: Allowing agents to learn to learn more efficiently.
- Improved algorithms: Developing more data-efficient and robust RL algorithms.
…are paving the way for more practical and widespread applications.
The Future of Reinforcement Learning:
The future of RL appears bright. Continued research and development will likely lead to more powerful and versatile RL algorithms, overcoming current limitations and unlocking new possibilities. While the hype may have been excessive in some instances, reinforcement learning remains a crucial area of AI research with the potential to revolutionize numerous industries. It's not a matter of being overhyped or underperforming, but rather a field undergoing rapid evolution, continuously refining its capabilities and expanding its impact on the world.
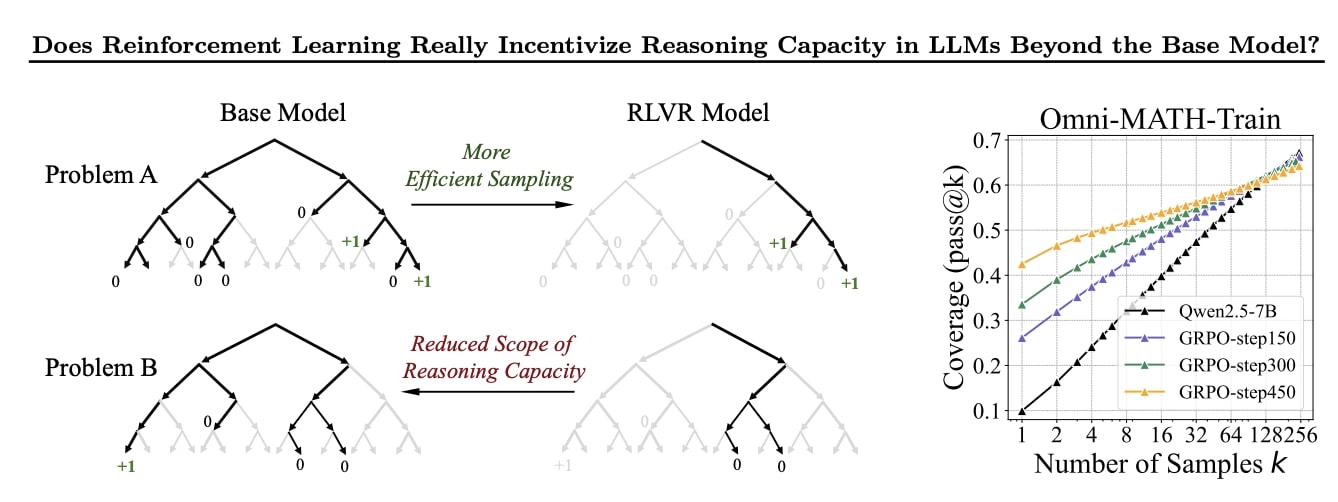
Thank you for visiting our website, your trusted source for the latest updates and in-depth coverage on Reinforcement Learning: Overhyped Or Underperforming In AI?. We're committed to keeping you informed with timely and accurate information to meet your curiosity and needs.
If you have any questions, suggestions, or feedback, we'd love to hear from you. Your insights are valuable to us and help us improve to serve you better. Feel free to reach out through our contact page.
Don't forget to bookmark our website and check back regularly for the latest headlines and trending topics. See you next time, and thank you for being part of our growing community!
Featured Posts
-
Experience Hans Zimmers Music Live Fall Concert In Fishers
Apr 30, 2025 -
Ligue Des Champions Suivez Le Match Arsenal Psg En Direct Et Les Compositions
Apr 30, 2025 -
Jeremy Renners Harrowing Account Seeing My Left Eye With My Right Eye After Snowplow Accident
Apr 30, 2025 -
Dwayne Johnsons Oscar Bid The Smashing Machine Trailer Debuts
Apr 30, 2025 -
Hans Zimmer Sydney Concert Was It Worth The Hype
Apr 30, 2025
Latest Posts
-
Arsenal Manager Warns Of Psg Danger Ahead Of Crucial Emirates Match
Apr 30, 2025 -
Ligue Des Champions Arsenal Vs Psg Compositions Officielles Avec Doue Et Dembele
Apr 30, 2025 -
Dte Energy Proposes 574 Million Rate Hike For Michigan Customers
Apr 30, 2025 -
Ligue Des Champions Le Psg Et Arsenal S Affrontent A Londres
Apr 30, 2025 -
Data Breach Alert Medical Software Companys Database Compromised Exposing Patient Data
Apr 30, 2025