Reinforcement Learning's Impact On AI: A Critical Analysis
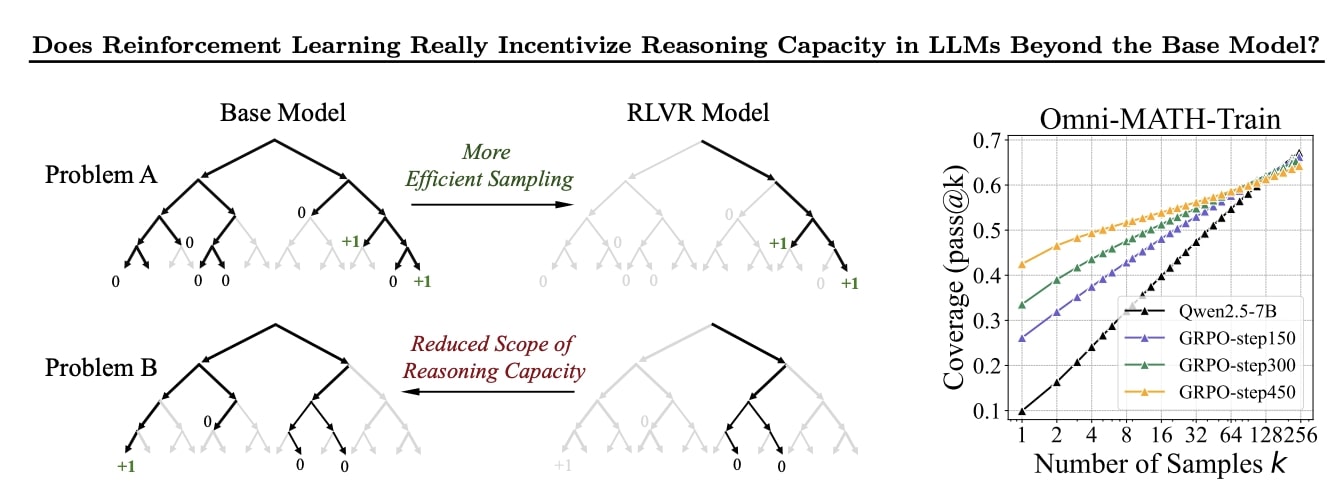
Welcome to your ultimate source for breaking news, trending updates, and in-depth stories from around the world. Whether it's politics, technology, entertainment, sports, or lifestyle, we bring you real-time updates that keep you informed and ahead of the curve.
Our team works tirelessly to ensure you never miss a moment. From the latest developments in global events to the most talked-about topics on social media, our news platform is designed to deliver accurate and timely information, all in one place.
Stay in the know and join thousands of readers who trust us for reliable, up-to-date content. Explore our expertly curated articles and dive deeper into the stories that matter to you. Visit NewsOneSMADCSTDO now and be part of the conversation. Don't miss out on the headlines that shape our world!
Table of Contents
Reinforcement Learning's Impact on AI: A Critical Analysis
Reinforcement learning (RL), a subfield of machine learning, is rapidly transforming the landscape of artificial intelligence. By enabling AI agents to learn through trial and error, RL has unlocked unprecedented capabilities in various sectors, from gaming and robotics to healthcare and finance. However, this powerful technology isn't without its challenges and limitations. This article offers a critical analysis of RL's impact, exploring its successes, limitations, and future potential.
RL's Triumphs: Game-Changing Applications
Reinforcement learning's success stories are plentiful. Perhaps the most widely known is DeepMind's AlphaGo, which famously defeated a world champion Go player. This victory showcased RL's ability to master complex games with vast search spaces, surpassing traditional AI approaches. Beyond gaming, RL is making significant strides in:
- Robotics: RL algorithms are training robots to perform intricate tasks, from assembling products in factories to navigating complex environments. This is leading to advancements in automation and improved efficiency across various industries.
- Healthcare: RL is being used to optimize treatment plans, personalize medicine, and even assist in drug discovery. By learning from patient data, RL algorithms can offer tailored solutions and potentially improve healthcare outcomes.
- Finance: RL algorithms are employed in algorithmic trading, risk management, and fraud detection. Their ability to adapt to changing market conditions makes them valuable tools in the financial sector.
The Challenges and Limitations of RL
Despite its impressive achievements, reinforcement learning faces several significant hurdles:
- Data Efficiency: RL algorithms often require vast amounts of data and extensive training time to achieve optimal performance. This can be computationally expensive and time-consuming, limiting its applicability in certain scenarios.
- Reward Function Design: Defining an appropriate reward function is crucial for successful RL. A poorly designed reward function can lead to unintended and undesirable behavior from the AI agent, a phenomenon known as reward hacking.
- Safety and Explainability: Understanding why an RL agent makes a particular decision can be challenging. This lack of transparency ("black box" nature) poses significant concerns in safety-critical applications, such as autonomous driving and medical diagnosis. Ensuring the safety and reliability of RL systems is paramount.
- Sample Inefficiency: RL agents often require many trials before converging on an optimal policy. This can be particularly problematic in real-world applications where experimentation is costly or dangerous.
The Future of Reinforcement Learning: Addressing the Challenges
Researchers are actively working on addressing the limitations of RL. Promising areas of research include:
- Improved Sample Efficiency: Developing algorithms that learn effectively from limited data is a key focus. Techniques like transfer learning and meta-learning are showing promise in this area.
- Safe RL: Research into safe RL aims to develop algorithms that guarantee safe and reliable behavior, even in unforeseen circumstances. This involves incorporating safety constraints and robust control mechanisms.
- Explainable RL (XRL): XRL seeks to make the decision-making process of RL agents more transparent and understandable. This is crucial for building trust and ensuring accountability.
Conclusion: A Powerful Tool with Unfinished Potential
Reinforcement learning is undoubtedly a transformative technology with the potential to revolutionize numerous fields. While challenges remain, the ongoing research and development in this area are paving the way for more robust, efficient, and reliable RL systems. Addressing the limitations concerning data efficiency, reward function design, safety, and explainability will be crucial for unlocking the full potential of RL and ensuring its responsible deployment across various applications. The future of AI is inextricably linked to the continued progress and refinement of reinforcement learning techniques.
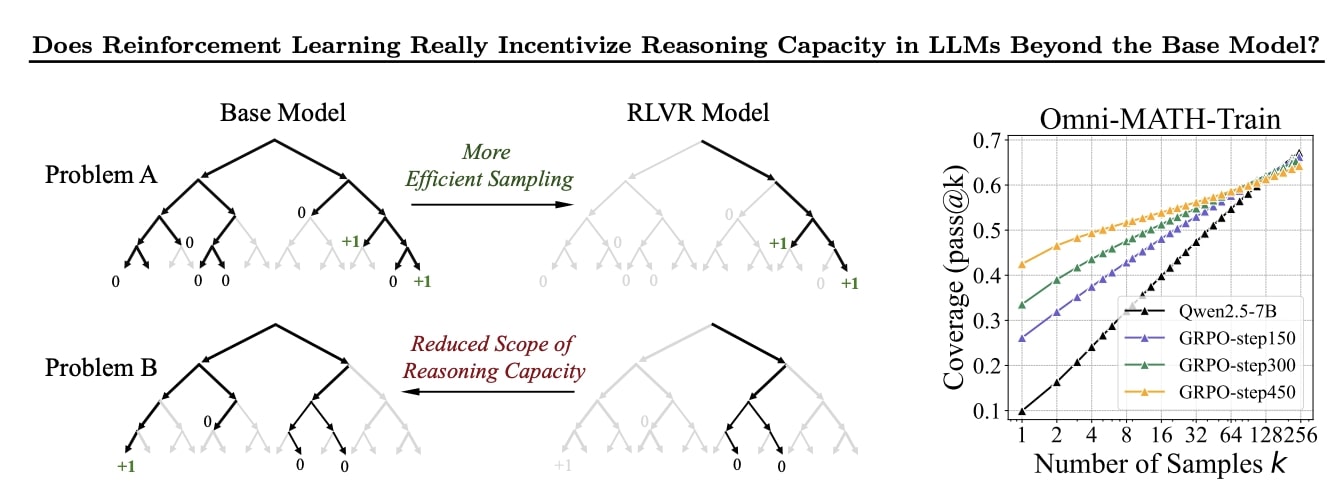
Thank you for visiting our website, your trusted source for the latest updates and in-depth coverage on Reinforcement Learning's Impact On AI: A Critical Analysis. We're committed to keeping you informed with timely and accurate information to meet your curiosity and needs.
If you have any questions, suggestions, or feedback, we'd love to hear from you. Your insights are valuable to us and help us improve to serve you better. Feel free to reach out through our contact page.
Don't forget to bookmark our website and check back regularly for the latest headlines and trending topics. See you next time, and thank you for being part of our growing community!
Featured Posts
-
Transfer Battle Heats Up Bayern Munich Eyes Bayer Leverkusens Jonathan Tah
May 02, 2025 -
Pre Season Football Snapdragon Cup Game Announced For Old Trafford
May 02, 2025 -
Big Bear Ai Or C3 Ai Analyzing The Top Contenders In The Ai Stock Market
May 02, 2025 -
Building Trust Through Honesty In Crisis Communication
May 02, 2025 -
Roma Fiorentina A Rischio Ranieri Squalificato Dal Giudice Sportivo Di Serie A
May 02, 2025
Latest Posts
-
Understanding Amazons New Tariff Display Policy
May 02, 2025 -
Haliburton Family Drama Father Restricted From Attending Pacers Matches
May 02, 2025 -
Is Google Building A Child Safe Gemini Ai Application
May 02, 2025 -
Artificial Pitch Concerns For Djurgarden Manager Maresca Speaks Out
May 02, 2025 -
Security Concerns Lead To Ban On Haliburtons Father At Pacers Games
May 02, 2025