Reinforcement Learning's Impact On AI: Hype Vs. Reality
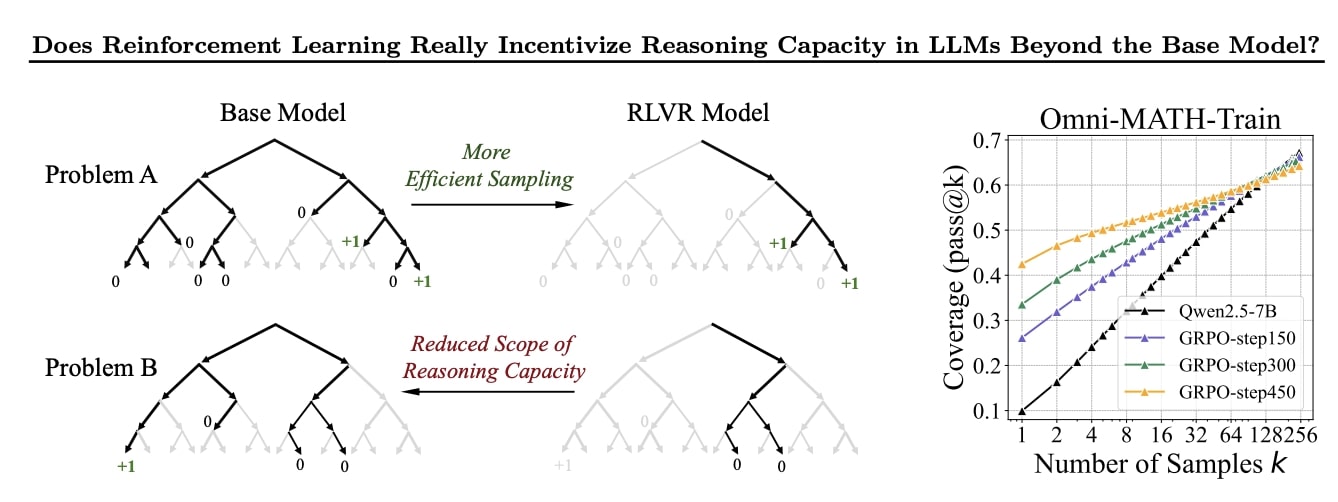
Welcome to your ultimate source for breaking news, trending updates, and in-depth stories from around the world. Whether it's politics, technology, entertainment, sports, or lifestyle, we bring you real-time updates that keep you informed and ahead of the curve.
Our team works tirelessly to ensure you never miss a moment. From the latest developments in global events to the most talked-about topics on social media, our news platform is designed to deliver accurate and timely information, all in one place.
Stay in the know and join thousands of readers who trust us for reliable, up-to-date content. Explore our expertly curated articles and dive deeper into the stories that matter to you. Visit NewsOneSMADCSTDO now and be part of the conversation. Don't miss out on the headlines that shape our world!
Table of Contents
Reinforcement Learning's Impact on AI: Hype vs. Reality
Reinforcement learning (RL), a subfield of machine learning, has captured the imagination of tech enthusiasts and industry experts alike. Promising breakthroughs in robotics, gaming, and beyond, RL has been lauded as the next big leap in artificial intelligence. But amidst the hype, what's the reality? Is RL truly revolutionizing AI, or is it simply over-hyped? This article delves into the current state of RL, examining its successes, limitations, and future potential.
The Allure of Reinforcement Learning
Reinforcement learning stands apart from other machine learning approaches by its focus on interaction and reward. Instead of learning from pre-labeled data, an RL agent learns through trial and error, receiving rewards for desirable actions and penalties for undesirable ones. This iterative process allows agents to learn complex behaviors and strategies in dynamic environments.
This paradigm has yielded impressive results:
- Mastering complex games: AlphaGo's victory over a world champion Go player remains a landmark achievement, showcasing RL's ability to conquer incredibly nuanced games. Similar successes have been seen in chess, poker, and video games like Dota 2 and StarCraft II.
- Robotics advancements: RL is driving progress in robotics, enabling robots to learn intricate manipulation tasks, navigate complex environments, and even exhibit collaborative behaviors.
- Personalized recommendations: From optimizing ad placements to suggesting products, RL algorithms are increasingly used to personalize user experiences and improve engagement.
- Autonomous driving: RL plays a crucial role in training autonomous vehicles to navigate unpredictable traffic situations and make safe driving decisions.
The Challenges and Limitations of RL
Despite its impressive achievements, RL faces significant hurdles:
- Data efficiency: RL agents often require vast amounts of data and extensive training time to achieve satisfactory performance. This can be computationally expensive and impractical for many real-world applications.
- Sample inefficiency: RL algorithms can struggle to learn efficiently from sparse rewards or delayed feedback, leading to slow learning and potentially suboptimal solutions.
- Safety and robustness: The trial-and-error nature of RL can lead to unpredictable and potentially unsafe behaviors, especially in critical applications like autonomous driving or medical robotics. Ensuring safety and robustness remains a major challenge.
- Explainability and interpretability: Understanding why an RL agent makes a particular decision can be difficult, hindering trust and acceptance in high-stakes applications. The "black box" nature of some RL algorithms limits their applicability in scenarios requiring transparency.
- Scalability: Scaling RL algorithms to complex, high-dimensional environments remains a significant challenge.
The Future of Reinforcement Learning
Despite these limitations, the future of RL looks bright. Ongoing research focuses on:
- Improving data efficiency: Researchers are exploring techniques like transfer learning and curriculum learning to reduce the data requirements for RL.
- Addressing safety concerns: Methods like reward shaping and safe exploration are being developed to ensure the safety and reliability of RL agents.
- Enhancing explainability: Work on developing more interpretable RL algorithms is ongoing, aiming to improve transparency and build trust.
- Developing more robust algorithms: Research is focused on creating RL algorithms that can handle noisy data, unexpected events, and adversarial attacks.
Conclusion: A Balanced Perspective
Reinforcement learning is a powerful tool with the potential to revolutionize numerous fields. However, it's crucial to maintain a balanced perspective, acknowledging both its impressive capabilities and its limitations. The hype surrounding RL is real, but its successful application requires careful consideration of its challenges and ongoing research to overcome them. The future of AI is undoubtedly intertwined with the progress of reinforcement learning, but realizing its full potential will demand continued innovation and a pragmatic approach.
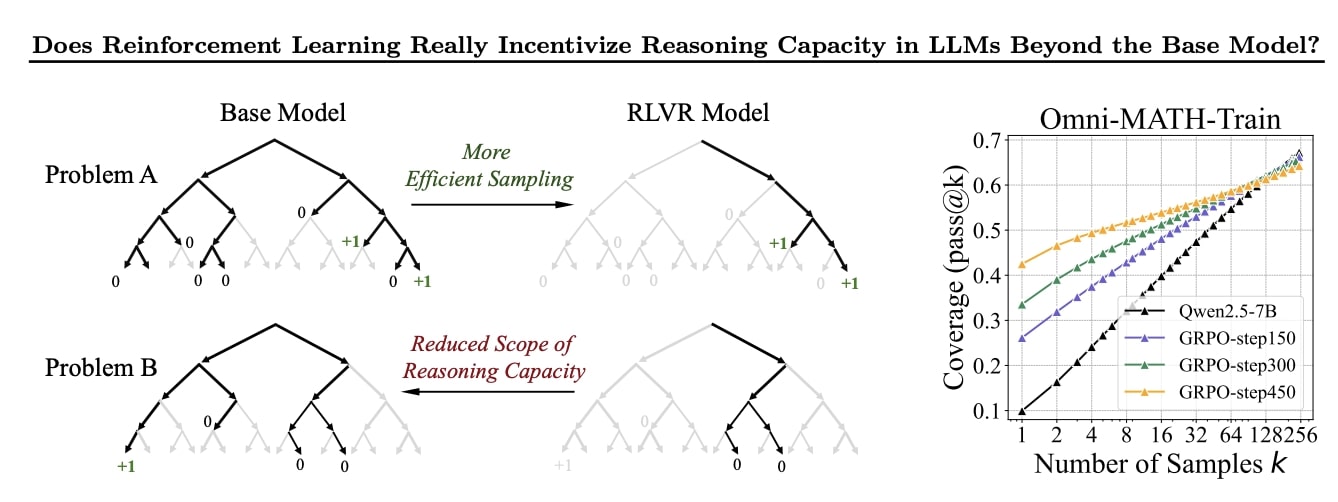
Thank you for visiting our website, your trusted source for the latest updates and in-depth coverage on Reinforcement Learning's Impact On AI: Hype Vs. Reality. We're committed to keeping you informed with timely and accurate information to meet your curiosity and needs.
If you have any questions, suggestions, or feedback, we'd love to hear from you. Your insights are valuable to us and help us improve to serve you better. Feel free to reach out through our contact page.
Don't forget to bookmark our website and check back regularly for the latest headlines and trending topics. See you next time, and thank you for being part of our growing community!
Featured Posts
-
Sharp Temperature Decline Alberta To Experience Near 15 Degree Temperature Fall
Apr 29, 2025 -
Ge 2025 Workers Partys Economic Policies Take Center Stage At Second Rally
Apr 29, 2025 -
Wfcu Centre Fire Forces Evacuation Voting Moved To New Location
Apr 29, 2025 -
Ge 2025 Good Faith Behind Allianz Income Deal Ntuc To Enhance Services Ng Chee Meng
Apr 29, 2025 -
Urgent Update Wfcu Centre Fire Prompts Full Evacuation Voting Changes
Apr 29, 2025
Latest Posts
-
Ms Vs Qg Live Score Todays Psl Match Playing Xi Toss Winner And Match Updates
Apr 30, 2025 -
Manchester United Pass On Nuno Mendes After 29 Million Psg Transfer
Apr 30, 2025 -
Urgent Officer Involved Shooting On Rideau Street Ottawa Details Emerge
Apr 30, 2025 -
The Rise Of Black Family Travel Exploring The Motivations And Impact
Apr 30, 2025 -
Matt Petgrave Criminal Charges Dropped Following Adam Johnsons Death
Apr 30, 2025