Reinforcement Learning's Limitations In Advancing AI Models
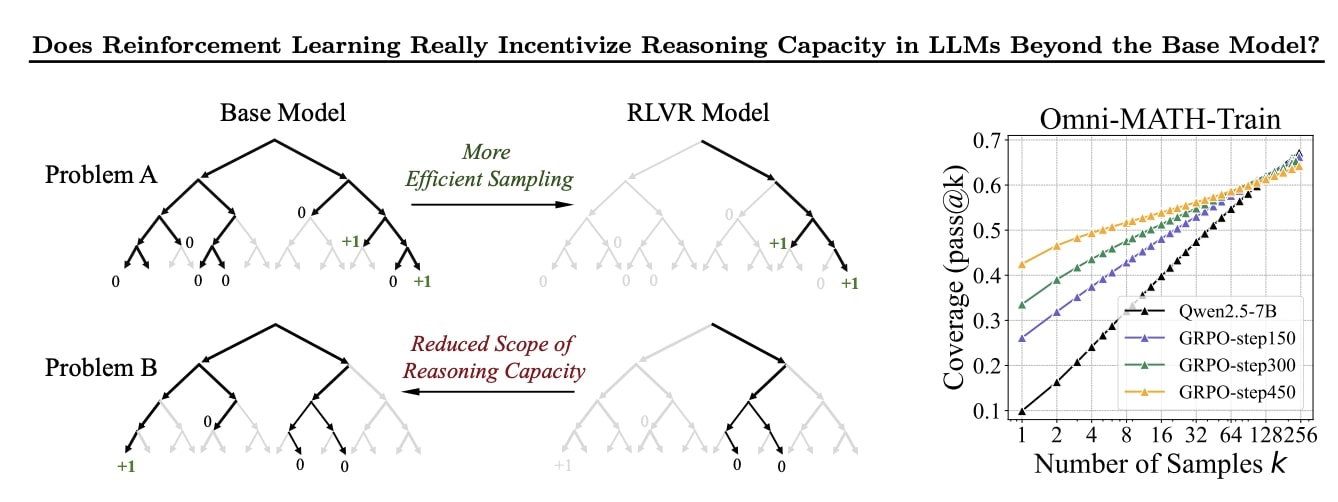
Welcome to your ultimate source for breaking news, trending updates, and in-depth stories from around the world. Whether it's politics, technology, entertainment, sports, or lifestyle, we bring you real-time updates that keep you informed and ahead of the curve.
Our team works tirelessly to ensure you never miss a moment. From the latest developments in global events to the most talked-about topics on social media, our news platform is designed to deliver accurate and timely information, all in one place.
Stay in the know and join thousands of readers who trust us for reliable, up-to-date content. Explore our expertly curated articles and dive deeper into the stories that matter to you. Visit NewsOneSMADCSTDO now and be part of the conversation. Don't miss out on the headlines that shape our world!
Table of Contents
Reinforcement Learning's Limitations: Why It's Not the Silver Bullet for AI Advancement
Reinforcement learning (RL), a powerful machine learning technique where agents learn through trial and error, has garnered significant attention for its potential to advance AI. However, despite impressive achievements like mastering complex games like Go and AlphaGo, RL faces significant limitations that hinder its broader application and prevent it from being the sole solution for building truly intelligent AI models. This article delves into these key challenges, exploring the hurdles that RL must overcome to reach its full potential.
The Sample Efficiency Problem: Data Hunger in RL
One of the most prominent limitations of RL is its sample inefficiency. Unlike supervised learning, which can often learn effectively from relatively small, labeled datasets, RL algorithms typically require vast amounts of data – often millions or even billions of interactions – to converge on optimal policies. This data hunger presents several challenges:
- High computational cost: Generating and processing this massive amount of data demands significant computational resources, making RL training expensive and time-consuming, especially for complex real-world applications.
- Real-world applicability: In many real-world scenarios, such as robotics or autonomous driving, gathering this much data can be impractical, dangerous, or prohibitively expensive. Simulations can help, but they often fail to perfectly capture the nuances of the real world, leading to performance degradation when transferring learned policies.
- Environmental limitations: The environment's dynamics might change over time, requiring continuous retraining and adaptation, further amplifying the data requirements.
Reward Function Engineering: Defining Success in AI
Another significant hurdle is designing effective reward functions. The reward function dictates what constitutes "success" for the RL agent. Poorly designed reward functions can lead to unexpected and even undesirable behaviors. The agent might find loopholes or exploit unintended aspects of the reward system to maximize its score, rather than achieving the intended goal. This is often referred to as the problem of reward hacking. Finding a balance between a concise and effective reward function that aligns precisely with the desired behavior is an ongoing area of active research.
Exploration-Exploitation Dilemma: The Balance of Learning and Performance
RL agents face the constant challenge of balancing exploration (trying new actions to discover potentially better strategies) and exploitation (using the current best-known strategy to maximize rewards). An insufficient emphasis on exploration can lead to suboptimal solutions, while excessive exploration can lead to slow convergence and inefficient learning. Effectively managing this trade-off is crucial for the success of RL algorithms, but remains a complex problem.
Generalization and Transfer Learning: The Challenge of Adaptability
While RL algorithms can excel at specific tasks, they often struggle with generalization – applying knowledge learned in one environment or task to another. This lack of adaptability limits their ability to handle unseen situations or transfer learned skills to new contexts. Transfer learning techniques are being explored to alleviate this issue, but achieving robust and reliable transfer remains a significant research challenge.
Conclusion: The Future of Reinforcement Learning
Despite these limitations, reinforcement learning remains a crucial tool for advancing AI. Ongoing research focuses on improving sample efficiency, developing more robust reward function design techniques, and enhancing generalization capabilities. Addressing these limitations is crucial for unlocking the full potential of RL and for creating truly intelligent, adaptable, and general-purpose AI systems. Further breakthroughs in these areas will undoubtedly shape the future of artificial intelligence and its applications across diverse fields.
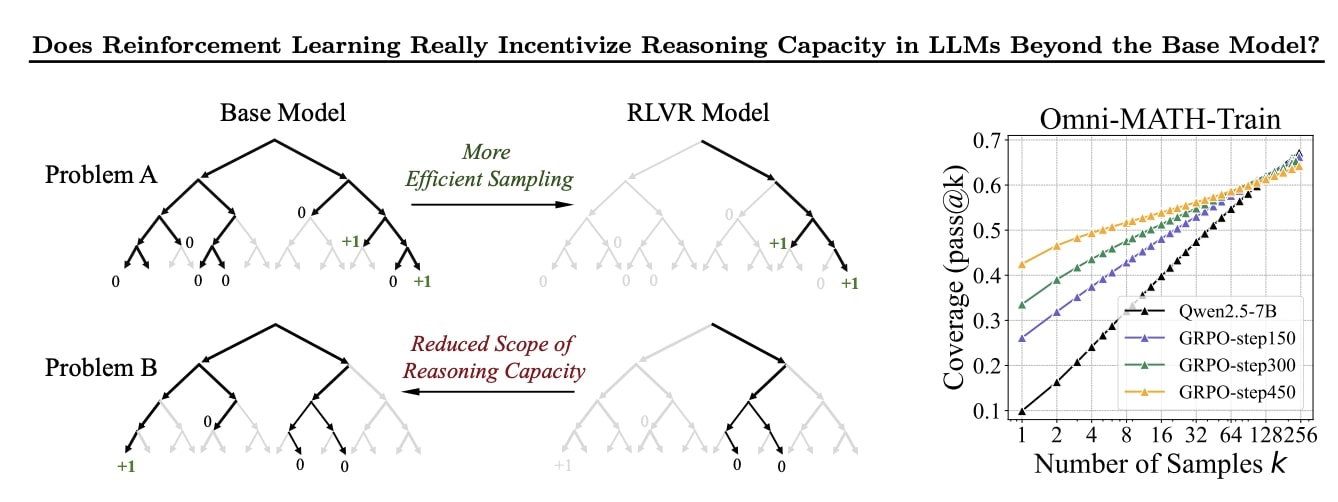
Thank you for visiting our website, your trusted source for the latest updates and in-depth coverage on Reinforcement Learning's Limitations In Advancing AI Models. We're committed to keeping you informed with timely and accurate information to meet your curiosity and needs.
If you have any questions, suggestions, or feedback, we'd love to hear from you. Your insights are valuable to us and help us improve to serve you better. Feel free to reach out through our contact page.
Don't forget to bookmark our website and check back regularly for the latest headlines and trending topics. See you next time, and thank you for being part of our growing community!
Featured Posts
-
Bitcoins Us Dominance A New High Driven By Wall Street And Exchange Traded Funds
Apr 28, 2025 -
New Study Boosts Probability Of Life On Ocean Exoplanet K2 18b To 99 7
Apr 28, 2025 -
Pogacar Et Evenepoel La Rivalite S Intensifie Avant La Nouvelle Saison
Apr 28, 2025 -
The Illusion Of Safety Understanding Web3 Verification Risks
Apr 28, 2025 -
Web3 Gaming Capitalizing On Nostalgia With Beloved Franchises
Apr 28, 2025
Latest Posts
-
Rider Beware Uber To De Platform Low Rated Users
Apr 30, 2025 -
Martian Cartographys Transformation Unveiling A New Red Planet
Apr 30, 2025 -
Ucl Semi Finals Arsenal Psg Barcelona And Inter Milan Face Off
Apr 30, 2025 -
Madden 26 And College Football 26 Release Date Announced Bundle Confirmed By Ea Sports
Apr 30, 2025 -
Dwayne The Rock Johnsons Oscar Campaign First Trailer For Black Adam Released
Apr 30, 2025