Reinforcement Learning's Limitations: Why It Doesn't Fundamentally Advance AI.
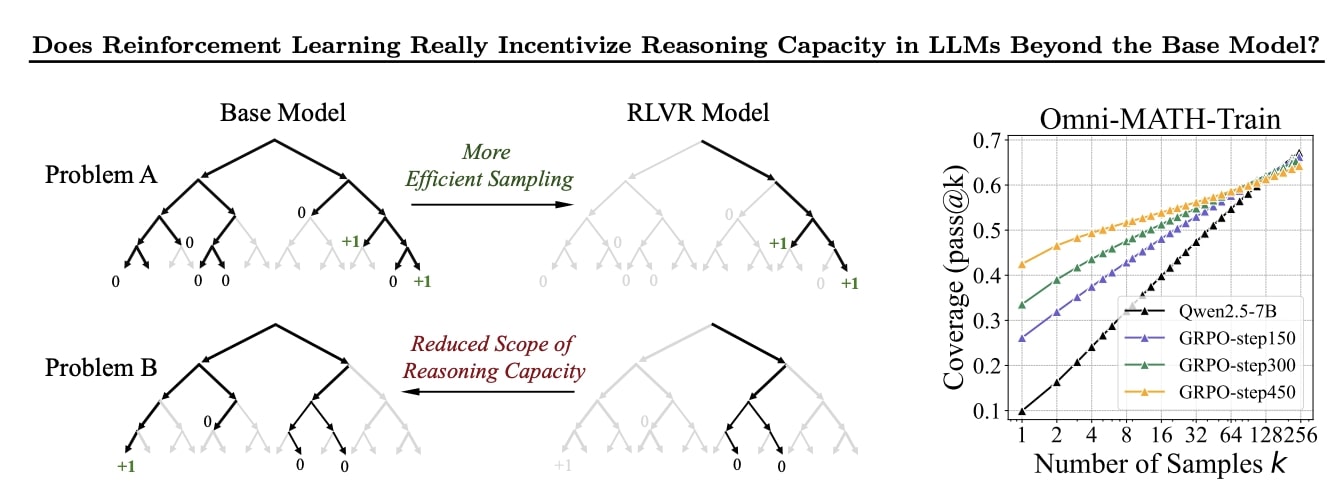
Welcome to your ultimate source for breaking news, trending updates, and in-depth stories from around the world. Whether it's politics, technology, entertainment, sports, or lifestyle, we bring you real-time updates that keep you informed and ahead of the curve.
Our team works tirelessly to ensure you never miss a moment. From the latest developments in global events to the most talked-about topics on social media, our news platform is designed to deliver accurate and timely information, all in one place.
Stay in the know and join thousands of readers who trust us for reliable, up-to-date content. Explore our expertly curated articles and dive deeper into the stories that matter to you. Visit NewsOneSMADCSTDO now and be part of the conversation. Don't miss out on the headlines that shape our world!
Table of Contents
Reinforcement Learning's Limitations: Why It Doesn't Fundamentally Advance AI
Reinforcement learning (RL), a hot topic in artificial intelligence (AI), has delivered impressive results in areas like game playing and robotics. AlphaGo's victory over a Go world champion remains a landmark achievement. However, the hype surrounding RL often overshadows its significant limitations, hindering its potential to fundamentally advance AI beyond narrow applications. This article delves into these limitations, exploring why RL, while powerful, falls short of being a truly transformative force in general AI.
The Data Dependency Dilemma: A Mountain of Samples Needed
One of the most significant drawbacks of RL is its immense data hunger. Unlike supervised learning, which leverages labeled datasets, RL algorithms learn through trial and error, requiring countless interactions with the environment to converge on optimal strategies. This necessitates enormous computational resources and time, making it impractical for many real-world scenarios. For example, training a robot to navigate a complex environment safely requires countless simulations or real-world interactions, each potentially leading to costly failures. This data dependency severely limits its scalability and applicability in domains where data acquisition is expensive or dangerous.
The Sample Inefficiency Problem: Slow and Steady Doesn't Always Win
RL algorithms often suffer from sample inefficiency. They require a massive number of trials to learn even relatively simple tasks. This inefficiency contrasts sharply with human learning, where we can often generalize from limited examples. This lack of efficiency translates to longer training times and higher computational costs, making RL unsuitable for many time-sensitive applications. The need for such extensive exploration before exploitation significantly hampers its progress.
The Generalization Gap: Mastering One Game Doesn't Mean Mastering All
Another key limitation is the difficulty of generalizing learned knowledge across different environments or tasks. An RL agent trained to excel at playing Atari games might struggle to adapt to a completely new game, even if the underlying principles are similar. This generalization gap highlights the lack of transfer learning capabilities inherent in many current RL algorithms. True AI should be able to leverage prior knowledge to learn new skills more efficiently, a feat that remains a significant challenge for RL.
The Reward Shaping Challenge: Defining Success is Hard
Defining appropriate reward functions is crucial for RL success. A poorly designed reward function can lead the agent to find unintended shortcuts or exploit loopholes, resulting in suboptimal or even harmful behavior. Creating reward functions that accurately capture the desired behavior in complex environments is a significant challenge, often requiring significant human expertise and iterative refinement. This reward shaping problem underscores the significant human intervention required, limiting RL's autonomy.
The Explainability Issue: A Black Box Approach
Many RL algorithms operate as black boxes, making it difficult to understand the reasoning behind their decisions. This lack of transparency is a serious concern in critical applications like healthcare and autonomous driving, where understanding the rationale behind AI actions is paramount. The difficulty in interpreting the learned policies makes it challenging to debug errors, identify biases, and ensure safety and reliability.
Conclusion: A Powerful Tool, But Not the Ultimate Solution
Reinforcement learning has undoubtedly shown its potential in specific domains. However, its limitations, particularly its data dependency, sample inefficiency, generalization gap, reward shaping challenges, and lack of explainability, significantly hinder its capacity to drive fundamental advancements in artificial general intelligence (AGI). While RL remains a powerful tool in the AI arsenal, it's crucial to acknowledge its boundaries and explore alternative approaches to achieve the goal of creating truly intelligent and versatile AI systems. Further research focusing on addressing these limitations is essential for unlocking RL's full potential and paving the way for more robust and generalizable AI.
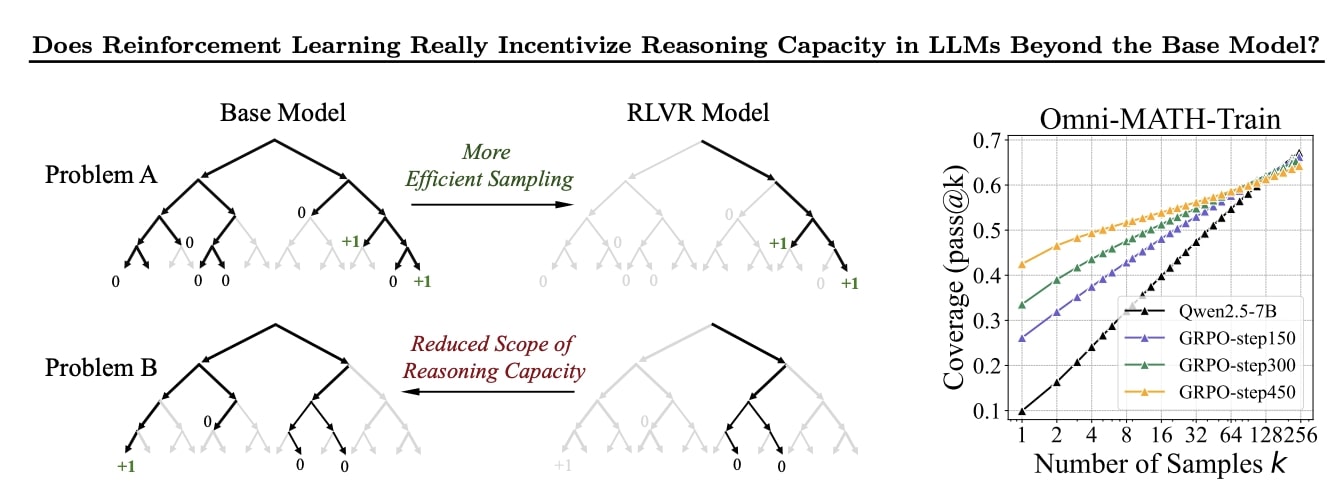
Thank you for visiting our website, your trusted source for the latest updates and in-depth coverage on Reinforcement Learning's Limitations: Why It Doesn't Fundamentally Advance AI.. We're committed to keeping you informed with timely and accurate information to meet your curiosity and needs.
If you have any questions, suggestions, or feedback, we'd love to hear from you. Your insights are valuable to us and help us improve to serve you better. Feel free to reach out through our contact page.
Don't forget to bookmark our website and check back regularly for the latest headlines and trending topics. See you next time, and thank you for being part of our growing community!
Featured Posts
-
Allegations Of Migrant Worker Exploitation Labor Ministry To Investigate Kmt Rally
Apr 29, 2025 -
Kohlis Kantara Recreation Teasing Rahul After Rcbs Delhi Victory
Apr 29, 2025 -
New Cmf Phone 2 Pro Announced Detailed Camera Specs And Features
Apr 29, 2025 -
Serie A Monday April 28th All The Action And Results
Apr 29, 2025 -
Boeings 737 Max And Other Aircraft Back In The Air After China Ban
Apr 29, 2025
Latest Posts
-
Improving Web Development Workflow With Googles Claybrook Ai
Apr 29, 2025 -
Investigation Into Large Scale Power Failure In Spain And Portugal Cyberattack Ruled Out
Apr 29, 2025 -
Election 2025 A Deep Dive Into Public Housing Policies
Apr 29, 2025 -
Virat Kohli And Kl Rahuls On Field Argument Umpires Decision At The Center
Apr 29, 2025 -
Rajasthan Royals Vs Gujarat Titans Afghanistans Growing Ipl Presence
Apr 29, 2025