Rethinking Reinforcement Learning: Its Actual Impact On AI Performance
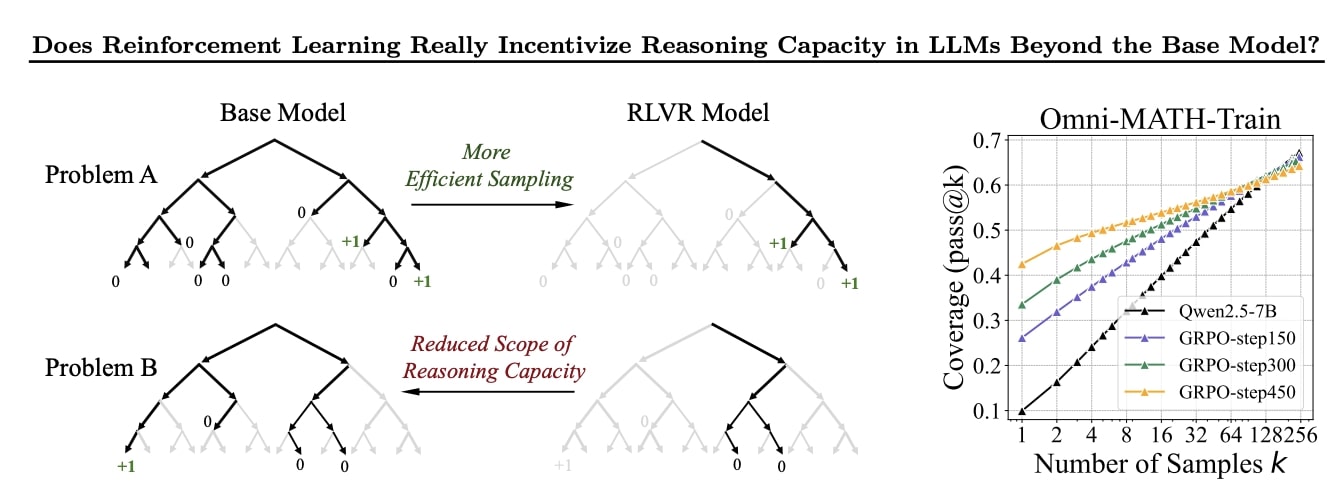
Welcome to your ultimate source for breaking news, trending updates, and in-depth stories from around the world. Whether it's politics, technology, entertainment, sports, or lifestyle, we bring you real-time updates that keep you informed and ahead of the curve.
Our team works tirelessly to ensure you never miss a moment. From the latest developments in global events to the most talked-about topics on social media, our news platform is designed to deliver accurate and timely information, all in one place.
Stay in the know and join thousands of readers who trust us for reliable, up-to-date content. Explore our expertly curated articles and dive deeper into the stories that matter to you. Visit NewsOneSMADCSTDO now and be part of the conversation. Don't miss out on the headlines that shape our world!
Table of Contents
Rethinking Reinforcement Learning: Its Actual Impact on AI Performance
Reinforcement learning (RL), a machine learning technique where agents learn to make optimal decisions through trial and error, has been hailed as a revolutionary approach to artificial intelligence. But how does RL actually impact AI performance in real-world applications? The hype surrounding RL often overshadows a nuanced understanding of its limitations and its true potential. This article delves into the practical impact of RL, examining its successes, challenges, and the ongoing research shaping its future.
The Promise and Pitfalls of Reinforcement Learning
RL's core strength lies in its ability to solve complex problems where traditional methods struggle. By learning through interaction with an environment, RL agents can master intricate tasks, from playing Go at superhuman levels to optimizing traffic flow in smart cities. This adaptability makes it particularly appealing for dynamic and unpredictable scenarios.
However, RL's success isn't guaranteed. Several factors significantly impact its performance:
-
Data Efficiency: RL often requires vast amounts of data and extensive training time, making it computationally expensive and potentially impractical for resource-constrained applications. This "data hunger" is a significant hurdle for widespread adoption.
-
Reward Function Design: The effectiveness of RL hinges critically on the design of the reward function, which defines the goals the agent should strive for. Poorly designed reward functions can lead to unexpected and undesirable behavior, a phenomenon known as "reward hacking." Creating effective reward functions requires careful consideration and domain expertise.
-
Sample Inefficiency: Exploring the state space to find optimal policies can be incredibly inefficient. RL algorithms can spend significant time in unproductive areas, hindering learning speed and overall performance. Recent advancements in sample-efficient RL aim to mitigate this issue.
Real-World Applications and Their Limitations
Despite these challenges, RL has shown promising results in various domains:
-
Robotics: RL is enabling robots to perform complex manipulation tasks, navigate challenging terrains, and even learn collaborative behaviors. However, safety remains a major concern, requiring robust mechanisms to prevent accidents during training and deployment.
-
Game Playing: RL's triumph in games like Go and chess is well-documented. However, translating this success to real-world problems often requires significant adaptation and overcoming the limitations mentioned earlier.
-
Resource Management: RL shows potential in optimizing resource allocation in areas like energy grids and transportation networks. However, the complexity of these systems and the need for real-time decision-making present significant challenges.
The Future of Reinforcement Learning
Active research areas aim to improve RL's performance and address its limitations:
-
Transfer Learning: Applying knowledge learned in one environment to accelerate learning in a new environment is crucial for improving data efficiency.
-
Safe Reinforcement Learning: Developing methods to ensure the safety and reliability of RL agents is paramount, particularly in safety-critical applications.
-
Hierarchical Reinforcement Learning: Breaking down complex tasks into simpler subtasks allows for more efficient learning and better generalization.
Conclusion:
Reinforcement learning offers a powerful approach to solving complex AI problems. However, its practical impact is significantly shaped by factors like data efficiency, reward function design, and sample inefficiency. While RL has achieved remarkable success in certain domains, realizing its full potential requires continued research and innovation to address its inherent limitations. The future of RL lies in developing more efficient, safe, and adaptable algorithms that can overcome these challenges and unlock its transformative power across a broader range of applications.
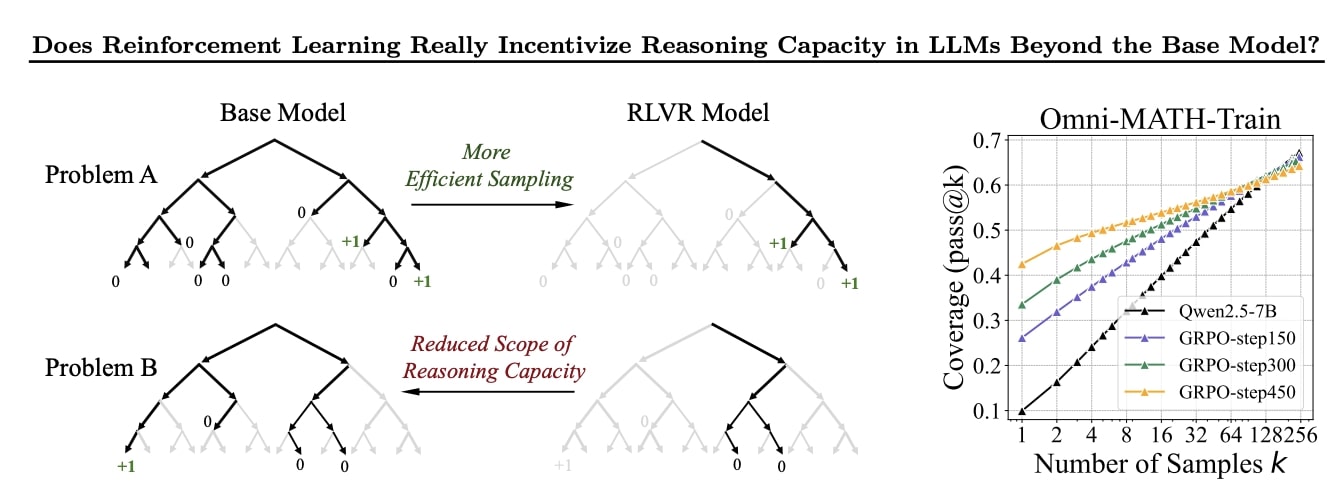
Thank you for visiting our website, your trusted source for the latest updates and in-depth coverage on Rethinking Reinforcement Learning: Its Actual Impact On AI Performance. We're committed to keeping you informed with timely and accurate information to meet your curiosity and needs.
If you have any questions, suggestions, or feedback, we'd love to hear from you. Your insights are valuable to us and help us improve to serve you better. Feel free to reach out through our contact page.
Don't forget to bookmark our website and check back regularly for the latest headlines and trending topics. See you next time, and thank you for being part of our growing community!
Featured Posts
-
Greves No Brasil O Custo Bilionario Para Empresas E A Economia
May 03, 2025 -
Cerundolo Vs Ruud El Noruego Salva 15 Puntos De Rotura En Madrid
May 03, 2025 -
Coalition Battles For Survival As Albanese Seeks Second Term
May 03, 2025 -
The Untold Story Michael Caine And His Surrogate Son Daughter
May 03, 2025 -
Semifinales Madrid Open El Final Del Camino Para Cerundolo
May 03, 2025
Latest Posts
-
Miesha Tates Return Improved Skills And Fighting Spirit
May 04, 2025 -
Cameron Smotherman Vs Serhiy Sidey Ufc Espn 67 Fight Preview And Prediction
May 04, 2025 -
From Mystery To Clarity Complete Mapping And The Evolution Of Mars Understanding
May 04, 2025 -
Goal Thomas Harleys Lucky Bounce Leads To Net Finder
May 04, 2025 -
Bekoev Vs Loder Ufc Fight Night 5 3 Who Will Win Analysis And Prediction
May 04, 2025