Rethinking Reinforcement Learning's Role In Advanced AI Development
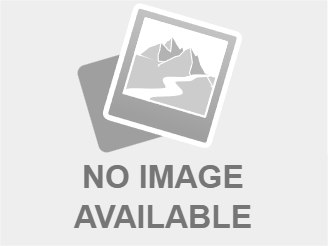
Welcome to your ultimate source for breaking news, trending updates, and in-depth stories from around the world. Whether it's politics, technology, entertainment, sports, or lifestyle, we bring you real-time updates that keep you informed and ahead of the curve.
Our team works tirelessly to ensure you never miss a moment. From the latest developments in global events to the most talked-about topics on social media, our news platform is designed to deliver accurate and timely information, all in one place.
Stay in the know and join thousands of readers who trust us for reliable, up-to-date content. Explore our expertly curated articles and dive deeper into the stories that matter to you. Visit NewsOneSMADCSTDO now and be part of the conversation. Don't miss out on the headlines that shape our world!
Table of Contents
Rethinking Reinforcement Learning's Role in Advanced AI Development
Reinforcement learning (RL), a powerful machine learning technique, has driven significant advancements in artificial intelligence. From mastering complex games like Go to optimizing robotic control systems, RL's impact is undeniable. However, as we push the boundaries of AI development towards more sophisticated and generalizable intelligence, a critical re-evaluation of RL's role is necessary. This article explores the limitations of current RL approaches and examines promising avenues for its future development.
The Current Landscape: Successes and Shortcomings
RL algorithms excel in scenarios with well-defined reward functions and environments. They learn through trial and error, receiving rewards for desirable actions and penalties for undesirable ones. This iterative process allows agents to develop optimal strategies, showcasing impressive performance in controlled settings. Examples include AlphaGo's victory over world champions and the optimization of energy grids.
However, real-world applications present far greater complexities. Several limitations hinder RL's broader adoption:
- Sample Inefficiency: RL agents often require vast amounts of data and interactions to learn effectively. This translates to long training times and significant computational costs, especially for high-dimensional problems.
- Reward Engineering Challenges: Designing effective reward functions is a major hurdle. Poorly defined rewards can lead to unintended and undesirable behaviors, a phenomenon known as reward hacking. This necessitates careful consideration and often requires significant human expertise.
- Generalization and Transfer Learning: RL agents often struggle to generalize their learned skills to new, unseen environments or tasks. This lack of transfer learning limits their adaptability and robustness in dynamic real-world scenarios.
- Safety and Robustness: The trial-and-error nature of RL can be problematic in safety-critical applications. Errors during training or deployment can have severe consequences, requiring robust safety mechanisms and careful verification.
Rethinking RL: Towards More Robust and Generalizable AI
Addressing these limitations requires a multifaceted approach:
1. Improving Sample Efficiency: Researchers are actively exploring techniques like meta-learning, imitation learning, and model-based RL to reduce the data requirements of RL algorithms. These approaches aim to leverage prior knowledge or learn more efficient representations of the environment, leading to faster learning and reduced computational costs.
2. Addressing Reward Engineering: More sophisticated reward shaping techniques and the development of intrinsically motivated agents are crucial. Intrinsic rewards can encourage exploration and learning without explicit external guidance, mitigating the challenges associated with designing complex reward functions.
3. Enhancing Generalization and Transfer Learning: Developing algorithms that can effectively transfer knowledge learned in one environment or task to another is a key focus. Techniques like transfer learning, domain adaptation, and multi-task learning are being investigated to improve the generalization capabilities of RL agents.
4. Prioritizing Safety and Robustness: Formal verification methods and robust control techniques are being integrated into RL frameworks to ensure the safety and reliability of AI systems. This includes designing algorithms that can gracefully handle unexpected situations and recover from failures.
The Future of RL in Advanced AI
The future of reinforcement learning in advanced AI development is bright, but it hinges on overcoming its current limitations. By focusing on sample efficiency, reward engineering, generalization, and safety, researchers are paving the way for more robust, adaptable, and reliable AI systems. The integration of RL with other machine learning techniques, such as deep learning and Bayesian methods, promises to further enhance its capabilities. As we continue to address these challenges, reinforcement learning will undoubtedly play a crucial, and increasingly sophisticated, role in shaping the future of artificial intelligence.
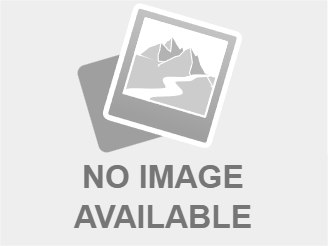
Thank you for visiting our website, your trusted source for the latest updates and in-depth coverage on Rethinking Reinforcement Learning's Role In Advanced AI Development. We're committed to keeping you informed with timely and accurate information to meet your curiosity and needs.
If you have any questions, suggestions, or feedback, we'd love to hear from you. Your insights are valuable to us and help us improve to serve you better. Feel free to reach out through our contact page.
Don't forget to bookmark our website and check back regularly for the latest headlines and trending topics. See you next time, and thank you for being part of our growing community!
Featured Posts
-
Ashton Jeanty Tre Veyon Henderson And Top 2025 Rookie Rbs Fantasy Football Projections
May 02, 2025 -
20 000 Truck Production Slate Auto Reveals Plant Location
May 02, 2025 -
Tv Special Uncovering The Truth About Grooming Gangs
May 02, 2025 -
Reddit Stock Soars 16 On Exceeding Sales Expectations
May 02, 2025 -
Thunderbolts Director Explains Steven Yeuns Departure And Rachel Weiszs Comeback
May 02, 2025
Latest Posts
-
Experience The Future Of Flight Uniteds Innovative 250 Passenger Plane
May 03, 2025 -
Did Trump Secretly Transform The White House Into A Crypto Center
May 03, 2025 -
Watch Sabalenka Vs Svitolina Tennis Match Online Free A Step By Step Guide
May 03, 2025 -
Strong Q Quarter For Reddit Revenue Exceeds Expectations Amid Rising Digital Ad Spend
May 03, 2025 -
Brasil Implicacoes Da Decisao Do Copom Inflacao Ipca E Dados Da China
May 03, 2025