Security Risks Of Granting Key Access To AI Models In Web3
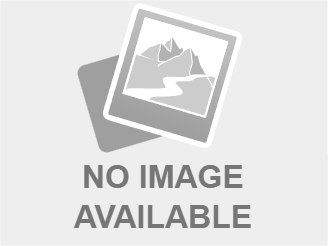
Welcome to your ultimate source for breaking news, trending updates, and in-depth stories from around the world. Whether it's politics, technology, entertainment, sports, or lifestyle, we bring you real-time updates that keep you informed and ahead of the curve.
Our team works tirelessly to ensure you never miss a moment. From the latest developments in global events to the most talked-about topics on social media, our news platform is designed to deliver accurate and timely information, all in one place.
Stay in the know and join thousands of readers who trust us for reliable, up-to-date content. Explore our expertly curated articles and dive deeper into the stories that matter to you. Visit NewsOneSMADCSTDO now and be part of the conversation. Don't miss out on the headlines that shape our world!
Table of Contents
Security Risks of Granting Key Access to AI Models in Web3: A Growing Concern
The decentralized promise of Web3 is intoxicating. But as Artificial Intelligence (AI) models become increasingly integrated into Web3 applications, a critical security vulnerability emerges: the risk associated with granting these models access to private keys and sensitive user data. This isn't just a theoretical threat; it represents a tangible and growing danger that could compromise the entire ecosystem.
The Allure of AI in Web3
AI offers significant potential benefits within the Web3 landscape. Decentralized Autonomous Organizations (DAOs) could leverage AI for enhanced decision-making, automating complex processes and improving efficiency. AI-powered tools can analyze vast blockchain datasets to identify trends, predict market movements, and even improve smart contract security. Furthermore, AI can personalize user experiences and enhance the accessibility of Web3 applications.
However, this integration introduces a significant security challenge. Many of these AI-driven features require access to private keys, transaction data, and other sensitive information to function effectively. This presents a clear attack vector for malicious actors.
Key Security Risks:
-
Private Key Compromise: Granting an AI model access to private keys, even with seemingly robust security measures, presents a major risk. A compromised AI model, whether through a vulnerability in its code or a targeted attack, could grant unauthorized access to user funds and assets. This could lead to significant financial losses and a devastating erosion of trust in the Web3 ecosystem.
-
Data Breaches and Leaks: AI models often process vast amounts of user data to learn and improve their performance. If this data is not properly secured, it becomes vulnerable to breaches and leaks. Sensitive information such as transaction history, financial details, and personal identifiers could fall into the wrong hands, leading to identity theft, fraud, and other serious consequences.
-
Algorithmic Manipulation: Sophisticated attackers might try to manipulate the AI model's algorithms to their advantage. This could involve injecting malicious code or exploiting vulnerabilities within the model's learning process to gain unauthorized control or manipulate the system's behavior for personal gain.
-
Lack of Transparency and Auditability: The complexity of AI models can make it difficult to fully understand their internal workings and ensure their security. This lack of transparency and auditability hinders the detection of potential vulnerabilities and makes it harder to identify and respond to attacks effectively.
Mitigation Strategies:
While the risks are significant, they are not insurmountable. Several strategies can help mitigate the security risks of integrating AI models into Web3:
-
Secure Enclaves and Hardware Security Modules (HSMs): Employing secure enclaves and HSMs can help protect private keys and sensitive data from unauthorized access, even if the AI model itself is compromised.
-
Homomorphic Encryption: This technique allows computations to be performed on encrypted data without decrypting it first, preserving data confidentiality even during AI model processing.
-
Federated Learning: This approach allows AI models to be trained on decentralized datasets without directly sharing the data itself, reducing the risk of data breaches.
-
Rigorous Auditing and Verification: Thorough audits and verification of AI models and their underlying code are crucial to identify and address potential security vulnerabilities.
Conclusion:
The integration of AI into Web3 presents both exciting opportunities and significant security challenges. Addressing these concerns proactively through robust security measures and a collaborative approach involving developers, security experts, and the broader Web3 community is crucial for ensuring the long-term security and success of this rapidly evolving ecosystem. Ignoring these risks could lead to widespread damage and severely hamper the adoption of Web3 technologies. The future of Web3 depends on a proactive and responsible approach to AI security.
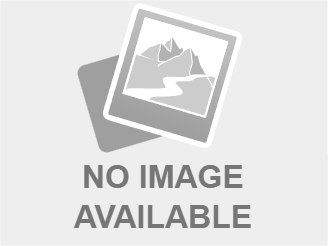
Thank you for visiting our website, your trusted source for the latest updates and in-depth coverage on Security Risks Of Granting Key Access To AI Models In Web3. We're committed to keeping you informed with timely and accurate information to meet your curiosity and needs.
If you have any questions, suggestions, or feedback, we'd love to hear from you. Your insights are valuable to us and help us improve to serve you better. Feel free to reach out through our contact page.
Don't forget to bookmark our website and check back regularly for the latest headlines and trending topics. See you next time, and thank you for being part of our growing community!
Featured Posts
-
Caitlin Clark Effect Indiana Fever Ticket Prices Skyrocket On Stub Hub
May 03, 2025 -
Apples Q Quarter Number Earnings To Suffer 900 Million Tariff Blow
May 03, 2025 -
Unprecedented Power Analyzing Aaron Judges Record Breaking Start
May 03, 2025 -
Legal Warning For Chef After Scathing Meghan Markle Cooking Show Review
May 03, 2025 -
Sec Ai And The Future Of Threat Investigation Highlights From Rsa 2025
May 03, 2025
Latest Posts
-
Watch Caitlin Clark Live Indiana Fever Vs Washington Mystics Tv Schedule And Start Time
May 03, 2025 -
Nea And Neh Funding Trump Administrations Proposed Cuts
May 03, 2025 -
Prime Video Expands Shop The Show A Boon For Business Or Bane For Storytelling
May 03, 2025 -
Long Road Ahead Padres Journey Begins In Pittsburgh
May 03, 2025 -
Ge 2025 What To Expect From Singapores 14th General Election
May 03, 2025