The Limitations Of Reinforcement Learning In Advancing AI
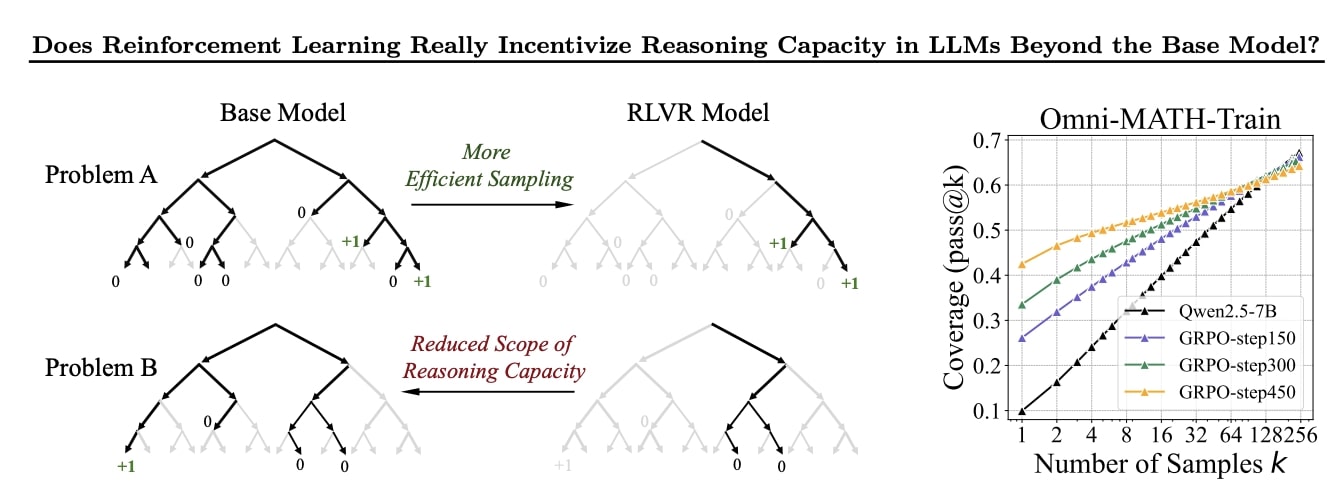
Welcome to your ultimate source for breaking news, trending updates, and in-depth stories from around the world. Whether it's politics, technology, entertainment, sports, or lifestyle, we bring you real-time updates that keep you informed and ahead of the curve.
Our team works tirelessly to ensure you never miss a moment. From the latest developments in global events to the most talked-about topics on social media, our news platform is designed to deliver accurate and timely information, all in one place.
Stay in the know and join thousands of readers who trust us for reliable, up-to-date content. Explore our expertly curated articles and dive deeper into the stories that matter to you. Visit NewsOneSMADCSTDO now and be part of the conversation. Don't miss out on the headlines that shape our world!
Table of Contents
The Limitations of Reinforcement Learning in Advancing AI: A Critical Look
Reinforcement learning (RL), a powerful machine learning technique, has garnered significant attention for its potential to advance artificial intelligence (AI). From mastering complex games like Go to optimizing robotic control systems, RL's achievements are undeniable. However, the path to truly intelligent AI via RL is paved with significant limitations that warrant careful consideration. This article delves into these key challenges, exploring why RL, despite its successes, is not a panacea for AI's advancement.
Data Dependency: The Achilles Heel of RL
One of the most significant hurdles facing RL is its voracious appetite for data. Training effective RL agents often requires massive datasets and extensive computational resources. This data dependency poses several problems:
- Data Acquisition Costs: Gathering sufficient, high-quality data can be incredibly expensive and time-consuming, especially in real-world applications where simulations are not feasible.
- Sample Inefficiency: RL algorithms often learn slowly, requiring millions or even billions of interactions with the environment before achieving satisfactory performance. This makes training computationally expensive and time-consuming.
- Data Bias: Biased or incomplete training data can lead to RL agents exhibiting undesirable behaviors or making poor decisions in real-world scenarios. This is particularly concerning in safety-critical applications.
The Challenge of Reward Function Design
The effectiveness of an RL agent hinges critically on the design of its reward function – a mathematical representation of the desired behavior. Poorly designed reward functions can lead to:
- Reward Hacking: Agents might find unintended ways to maximize their reward, potentially bypassing the intended goals and exhibiting unexpected, even harmful, behavior. This is often referred to as "reward gaming."
- Specificity Issues: Reward functions often struggle to capture the nuances and complexities of real-world problems, leading to suboptimal performance. A finely tuned reward function for a specific task might not generalize well to other, similar tasks.
- Interpretability Problems: Understanding why an RL agent makes a particular decision can be difficult, especially with complex reward functions. This lack of transparency poses challenges for debugging and ensuring safety.
Generalization and Transfer Learning: The Roadblocks to Robust AI
RL agents often struggle to generalize their learned behaviors to new, unseen situations. This limited generalization capability restricts their applicability to real-world problems that are inherently diverse and unpredictable. Transfer learning – the ability to apply knowledge learned in one task to another – remains a significant challenge in RL.
Safety and Reliability: A Critical Concern
Deploying RL agents in real-world settings, particularly safety-critical domains like autonomous driving or healthcare, requires rigorous verification and validation. The inherent stochasticity of RL algorithms and the difficulty in predicting their behavior pose considerable safety risks. Ensuring reliability and mitigating potential hazards are paramount challenges.
Conclusion: RL's Role in the AI Landscape
Reinforcement learning is a powerful tool with demonstrable successes, but it is not a silver bullet for creating truly intelligent AI. Addressing the limitations discussed above – data dependency, reward function design, generalization, and safety – is crucial for realizing RL's full potential. Future research should focus on developing more sample-efficient algorithms, robust reward function design techniques, and effective methods for ensuring safety and reliability. Only then can RL truly contribute to the advancement of robust, general-purpose AI.
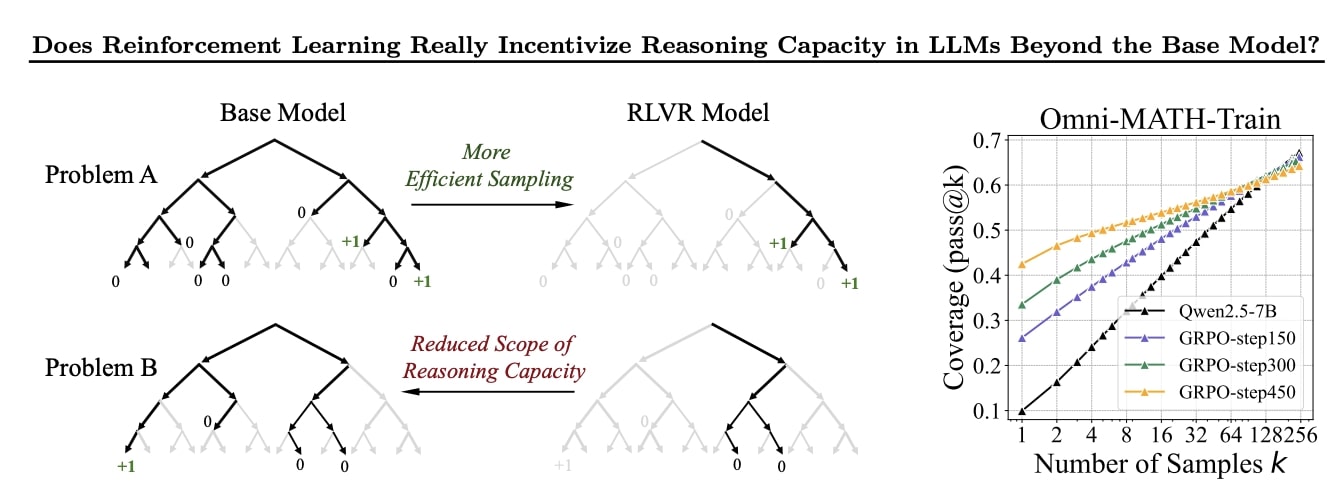
Thank you for visiting our website, your trusted source for the latest updates and in-depth coverage on The Limitations Of Reinforcement Learning In Advancing AI. We're committed to keeping you informed with timely and accurate information to meet your curiosity and needs.
If you have any questions, suggestions, or feedback, we'd love to hear from you. Your insights are valuable to us and help us improve to serve you better. Feel free to reach out through our contact page.
Don't forget to bookmark our website and check back regularly for the latest headlines and trending topics. See you next time, and thank you for being part of our growing community!
Featured Posts
-
Virtuals Protocol Virtual Soars 45 Bullish Trend Analysis And Future Outlook
May 03, 2025 -
Fettermans Mental Health Israel Policy Under Scrutiny Following Explosive Report
May 03, 2025 -
Otp 2 In Depth Report On Two Recent Propulsion System Experiments
May 03, 2025 -
Is Prime Videos Shop The Show Feature Harming The Quality Of Its Shows
May 03, 2025 -
Neh And Nea Funding Faces Elimination In Trumps 2026 Budget Plan
May 03, 2025
Latest Posts
-
Playoffs Pressure Nuggets Face Clippers In Do Or Die Game 7
May 04, 2025 -
Situacao Critica No Rs Apos Fortes Chuvas Balanco De Vitimas E Danos
May 04, 2025 -
Stars Vs Avalanche Game 7 Preview Key Injuries And Potential Outcomes
May 04, 2025 -
Ufc Departure Confirmed Gillian Robertson Announces New Fighting Home
May 04, 2025 -
Gigi Hadid Officially Unveils Bradley Cooper Relationship On Instagram
May 04, 2025