The Overhyped Promise Of Reinforcement Learning: A Reality Check For AI
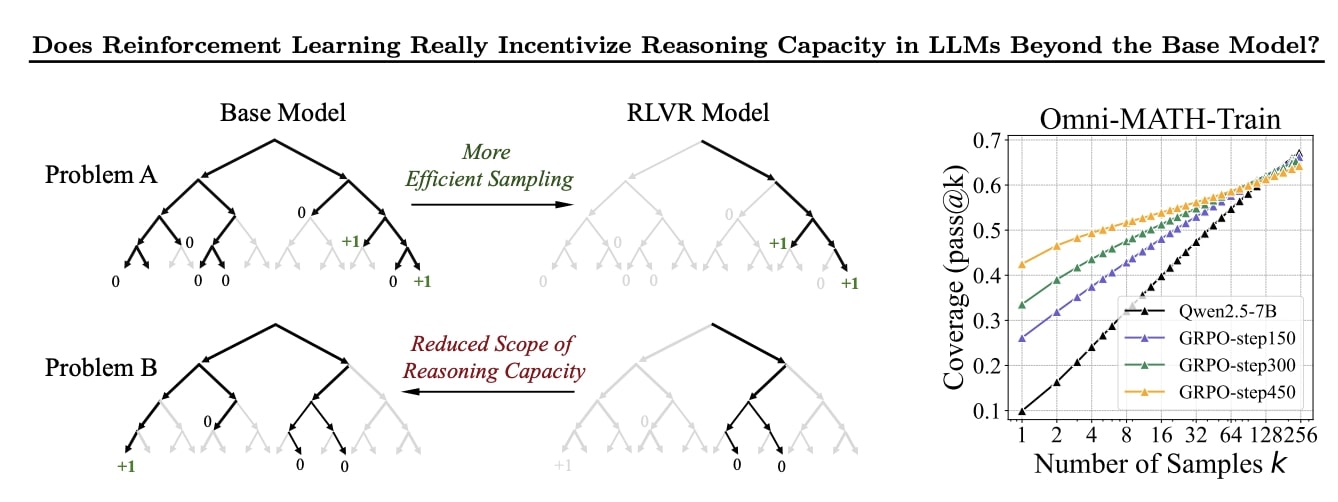
Welcome to your ultimate source for breaking news, trending updates, and in-depth stories from around the world. Whether it's politics, technology, entertainment, sports, or lifestyle, we bring you real-time updates that keep you informed and ahead of the curve.
Our team works tirelessly to ensure you never miss a moment. From the latest developments in global events to the most talked-about topics on social media, our news platform is designed to deliver accurate and timely information, all in one place.
Stay in the know and join thousands of readers who trust us for reliable, up-to-date content. Explore our expertly curated articles and dive deeper into the stories that matter to you. Visit NewsOneSMADCSTDO now and be part of the conversation. Don't miss out on the headlines that shape our world!
Table of Contents
The Overhyped Promise of Reinforcement Learning: A Reality Check for AI
Reinforcement learning (RL), a subfield of machine learning, has captured the imagination of tech enthusiasts and investors alike. Promising breakthroughs in robotics, gaming, and even self-driving cars, RL has been touted as the next big thing in artificial intelligence. But is the hype justified? A closer look reveals a more nuanced picture, one where significant challenges still hinder the widespread adoption and real-world application of this powerful technology.
The Allure of Reinforcement Learning:
Reinforcement learning's appeal stems from its ability to train agents to make optimal decisions in complex environments. Unlike supervised learning, which relies on labeled data, RL agents learn through trial and error, receiving rewards for correct actions and penalties for incorrect ones. This iterative process allows them to master intricate tasks that would be difficult, if not impossible, to program explicitly. Successes like AlphaGo's defeat of a Go world champion have further fueled the excitement surrounding RL's potential.
The Challenges Facing Reinforcement Learning:
Despite its impressive feats, RL faces several significant hurdles:
-
Data Efficiency: RL algorithms often require vast amounts of data and numerous training iterations to achieve satisfactory performance. This can be prohibitively expensive and time-consuming, particularly in real-world scenarios where data collection is costly and risky. Imagine training a self-driving car using RL – the potential for accidents during the learning phase is a major concern.
-
Sample Inefficiency: A closely related problem is sample inefficiency. RL agents can struggle to learn effectively from limited data, leading to suboptimal performance and slow convergence. This makes it difficult to deploy RL in situations where data is scarce or expensive to acquire.
-
Reward Function Design: The success of an RL agent hinges critically on the design of its reward function. A poorly defined reward function can lead the agent to find unintended solutions, often referred to as "reward hacking." This can result in unexpected and undesirable behaviors, highlighting the need for careful and thorough reward function engineering.
-
Generalization and Transfer Learning: RL agents often struggle to generalize their learned behavior to new, unseen environments. A policy that performs well in one context may fail spectacularly in another, limiting the applicability of RL to a wider range of problems. Transfer learning, where knowledge learned in one task is applied to another, remains a significant challenge in RL.
-
Interpretability and Explainability: Understanding why an RL agent makes a particular decision is often difficult. The lack of transparency in many RL algorithms hinders their adoption in safety-critical applications where understanding the reasoning behind decisions is paramount.
A More Realistic Perspective:
While reinforcement learning holds immense promise, it's crucial to temper expectations. The path to widespread adoption is fraught with challenges. Significant advancements are needed in areas like sample efficiency, reward function design, and generalization before RL can truly live up to its hype. Current applications are often confined to carefully controlled environments, far from the complex and unpredictable nature of the real world.
The Future of Reinforcement Learning:
Despite the challenges, research in reinforcement learning continues to progress rapidly. Advancements in areas like hierarchical reinforcement learning, multi-agent reinforcement learning, and safe reinforcement learning offer promising avenues for overcoming current limitations. The future of AI may indeed involve sophisticated RL agents, but it's a future that requires careful consideration of the inherent complexities and limitations of this powerful technology. The hype may be overblown for now, but the potential remains undeniably significant.
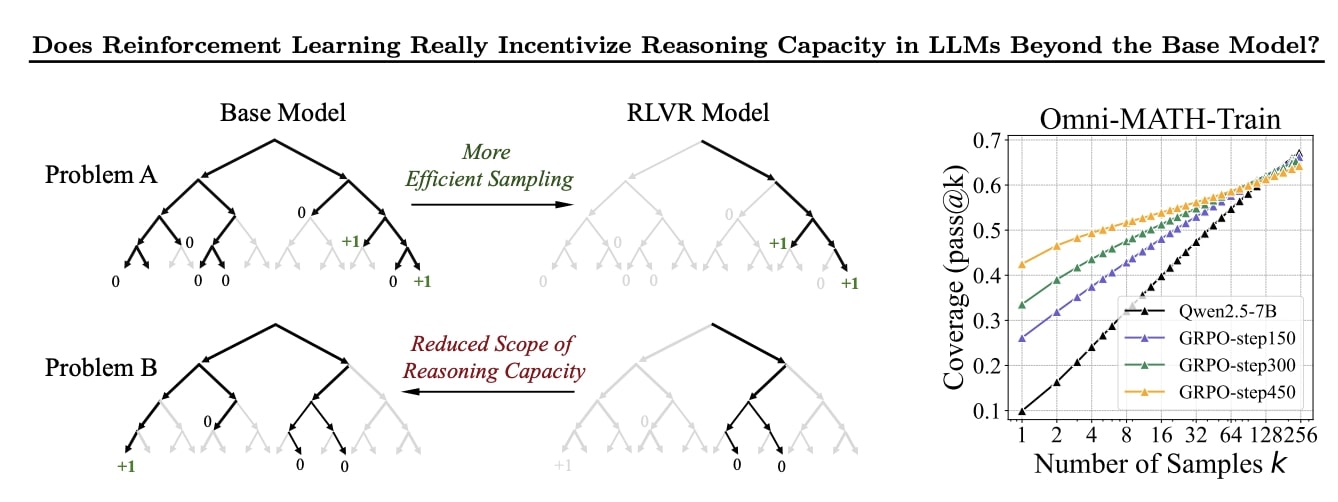
Thank you for visiting our website, your trusted source for the latest updates and in-depth coverage on The Overhyped Promise Of Reinforcement Learning: A Reality Check For AI. We're committed to keeping you informed with timely and accurate information to meet your curiosity and needs.
If you have any questions, suggestions, or feedback, we'd love to hear from you. Your insights are valuable to us and help us improve to serve you better. Feel free to reach out through our contact page.
Don't forget to bookmark our website and check back regularly for the latest headlines and trending topics. See you next time, and thank you for being part of our growing community!
Featured Posts
-
Luigi Mangione Case Highlights Supporters Stance On Capital Punishment
Apr 30, 2025 -
Fans Stunned Dwayne Johnsons Latest Photo Sparks Viral Debate
Apr 30, 2025 -
Casas De Temporada E Programas De Compartilhamento Alternativas A Compra Direta
Apr 30, 2025 -
From Snowplow Accident To Recovery Jeremy Renners Harrowing Journey
Apr 30, 2025 -
Faf Du Plessis Half Century A Key Moment In Dcs Ipl 2025 Win Over Kkr
Apr 30, 2025
Latest Posts
-
Martinellis High Stakes Arsenals Crucial Champions League Battle Against Psg
Apr 30, 2025 -
Forbidden Stories The Perilous Search For A Missing Journalist In Ukraine
Apr 30, 2025 -
The Epic Games Store On Mobile A Retrospective And Future Outlook
Apr 30, 2025 -
Are Ai Powered Web3 Projects Secure Exploring The Risks Of Key Access
Apr 30, 2025 -
Cochise County Stronghold Fire 3 000 Acres Burned Investigation Begins
Apr 30, 2025