The Reality Check: Reinforcement Learning And AI Performance
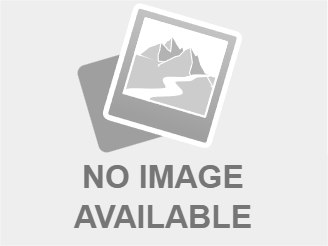
Welcome to your ultimate source for breaking news, trending updates, and in-depth stories from around the world. Whether it's politics, technology, entertainment, sports, or lifestyle, we bring you real-time updates that keep you informed and ahead of the curve.
Our team works tirelessly to ensure you never miss a moment. From the latest developments in global events to the most talked-about topics on social media, our news platform is designed to deliver accurate and timely information, all in one place.
Stay in the know and join thousands of readers who trust us for reliable, up-to-date content. Explore our expertly curated articles and dive deeper into the stories that matter to you. Visit NewsOneSMADCSTDO now and be part of the conversation. Don't miss out on the headlines that shape our world!
Table of Contents
The Reality Check: Reinforcement Learning and AI Performance – Hype vs. Reality
Artificial intelligence (AI) is rapidly transforming industries, and reinforcement learning (RL) is at the forefront of this revolution. Promising superhuman performance in complex tasks, RL has captured the imagination of researchers and investors alike. But how does the reality of RL's performance stack up against the hype? This article delves into the current state of RL, exploring its successes, limitations, and the crucial factors impacting its actual performance.
Reinforcement learning, a subfield of machine learning, focuses on training AI agents to make optimal decisions in dynamic environments. Unlike supervised learning, which relies on labeled data, RL agents learn through trial and error, receiving rewards for desirable actions and penalties for undesirable ones. This iterative process allows agents to master intricate tasks, leading to breakthroughs in areas like game playing, robotics, and resource management.
H2: Triumphs of Reinforcement Learning:
RL has achieved remarkable feats. DeepMind's AlphaGo, which defeated a world champion Go player, stands as a testament to RL's power. Similarly, AlphaStar's mastery of StarCraft II demonstrated the ability of RL to conquer complex, real-time strategy games. These successes are not isolated incidents. RL is also driving progress in:
- Robotics: Improving robot dexterity, navigation, and manipulation skills in dynamic environments.
- Resource Management: Optimizing energy grids, traffic flow, and supply chains for increased efficiency.
- Personalized Medicine: Developing treatment plans tailored to individual patient needs.
- Finance: Creating sophisticated trading algorithms and risk management strategies.
H2: The Limitations and Challenges of RL:
Despite its impressive achievements, RL faces significant hurdles that prevent widespread, seamless deployment.
- Data Efficiency: RL algorithms often require vast amounts of data and training time, making them computationally expensive and potentially unsustainable.
- Sample Inefficiency: Exploration vs. exploitation remains a critical challenge. Balancing the need to explore different actions with the need to exploit known effective strategies is crucial but often difficult to optimize.
- Generalization: RL agents often struggle to generalize their learned behavior to new, unseen environments or tasks. This limits their adaptability and robustness.
- Safety and Robustness: Ensuring the safe and reliable operation of RL agents, especially in critical applications, is paramount but remains a significant challenge. Unforeseen consequences and catastrophic failures are potential risks.
- Interpretability and Explainability: Understanding why an RL agent makes a specific decision is often difficult, hindering trust and deployment in high-stakes scenarios. This "black box" nature limits its applicability in certain domains.
H2: Factors Affecting Real-World RL Performance:
Several factors influence the real-world performance of RL systems:
- Reward Function Design: A poorly designed reward function can lead to unintended and undesirable behavior. Careful consideration is crucial for aligning AI agent actions with desired outcomes.
- Environment Modeling: Accurate and realistic environment modeling is essential for effective training. Inaccuracies can lead to poor generalization and unexpected failures in real-world deployments.
- Computational Resources: The computational demands of RL can be substantial, limiting its accessibility and scalability.
H2: The Future of Reinforcement Learning:
Despite these challenges, the future of RL is bright. Ongoing research focuses on:
- Improving data efficiency: Developing algorithms that learn more effectively from limited data.
- Enhanced generalization: Creating agents capable of adapting to new and unforeseen situations.
- Addressing safety and robustness concerns: Developing techniques to ensure the safe and reliable operation of RL systems.
- Improving interpretability and explainability: Making RL agents more transparent and understandable.
In conclusion, while reinforcement learning has achieved remarkable milestones, it's crucial to temper expectations. The journey towards truly intelligent, adaptable, and safe RL agents is ongoing. Addressing the current limitations will be key to unlocking the full potential of this transformative technology and bridging the gap between hype and reality.
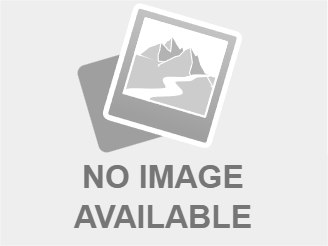
Thank you for visiting our website, your trusted source for the latest updates and in-depth coverage on The Reality Check: Reinforcement Learning And AI Performance. We're committed to keeping you informed with timely and accurate information to meet your curiosity and needs.
If you have any questions, suggestions, or feedback, we'd love to hear from you. Your insights are valuable to us and help us improve to serve you better. Feel free to reach out through our contact page.
Don't forget to bookmark our website and check back regularly for the latest headlines and trending topics. See you next time, and thank you for being part of our growing community!
Featured Posts
-
Jordan Travis Retirement Analyzing The Impact On The New York Jets
May 01, 2025 -
El Inter De Milan Y La Presion Interna Cuatro Jugadores Del Barca Intentaron Influir En Flick
May 01, 2025 -
Hansi Flicks Champions League Decision Barcelonas Uefa Rule Dilemma Resolved
May 01, 2025 -
The Gravity Of The Threat Decoding They Will Unleash Hell On Us
May 01, 2025 -
Manchester United Vs Chelsea Womens Super League Live Stream And Match Preview
May 01, 2025
Latest Posts
-
The Impact Of Brendan Carrs Leadership On Fcc Consumer Policies
May 01, 2025 -
Barca Inter Styles Opposes Pour Une Demi Finale Explosive De Ligue Des Champions
May 01, 2025 -
Asi Luce El Cesped Del Nuevo Camp Nou El Regreso Del Fc Barcelona Esta Mas Cerca
May 01, 2025 -
Propellantless Drive Technology Challenges And Potential For Future Space Exploration
May 01, 2025 -
Spain And Portugal Restore Power After Widespread Outage Investigation Underway
May 01, 2025