The Reality Of Reinforcement Learning: Does It Deliver On Its AI Promises?
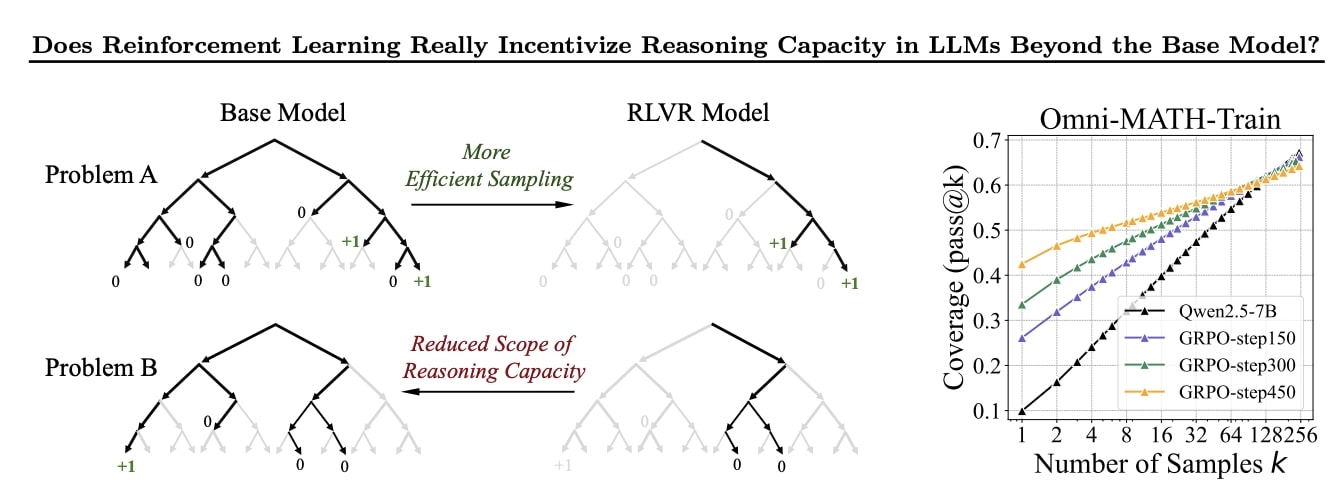
Welcome to your ultimate source for breaking news, trending updates, and in-depth stories from around the world. Whether it's politics, technology, entertainment, sports, or lifestyle, we bring you real-time updates that keep you informed and ahead of the curve.
Our team works tirelessly to ensure you never miss a moment. From the latest developments in global events to the most talked-about topics on social media, our news platform is designed to deliver accurate and timely information, all in one place.
Stay in the know and join thousands of readers who trust us for reliable, up-to-date content. Explore our expertly curated articles and dive deeper into the stories that matter to you. Visit NewsOneSMADCSTDO now and be part of the conversation. Don't miss out on the headlines that shape our world!
Table of Contents
The Reality of Reinforcement Learning: Does it Deliver on its AI Promises?
Reinforcement learning (RL), a subfield of artificial intelligence (AI), has captured the imagination with its potential to create intelligent agents capable of learning complex tasks. From mastering games like Go to optimizing robotics control, RL's advancements are undeniable. But amidst the hype, a crucial question remains: does RL truly deliver on its ambitious promises? This article delves into the reality of reinforcement learning, exploring its successes, limitations, and future prospects.
The Allure of Reinforcement Learning:
Reinforcement learning operates on a simple yet powerful principle: learning through trial and error. An RL agent interacts with an environment, receiving rewards for desirable actions and penalties for undesirable ones. Through this iterative process, the agent learns an optimal policy—a strategy for maximizing its cumulative reward. This approach holds immense appeal because it mimics how humans and animals learn, allowing for the development of adaptable and intelligent systems.
RL's Triumphs: Real-World Applications:
While still a relatively young field, RL has already achieved remarkable successes:
- Game Playing: DeepMind's AlphaGo famously defeated a world champion Go player, showcasing RL's ability to master complex games with immense search spaces. Similar successes have been achieved in other games like chess and StarCraft II.
- Robotics: RL algorithms are increasingly used to control robots, enabling them to perform intricate tasks such as grasping objects, navigating complex environments, and even cooperating with humans.
- Resource Optimization: In industries like energy and telecommunications, RL is employed to optimize resource allocation, leading to increased efficiency and cost savings.
- Personalized Recommendations: E-commerce platforms utilize RL to personalize product recommendations, enhancing user experience and driving sales.
The Challenges and Limitations:
Despite its successes, RL faces significant hurdles:
- Sample Inefficiency: RL agents often require vast amounts of data and interaction with the environment to learn effectively. This can be computationally expensive and time-consuming, especially in real-world applications.
- Reward Design: Defining appropriate reward functions is crucial for RL's success. Poorly designed reward functions can lead to unexpected and undesirable behaviors, a problem known as reward hacking.
- Safety and Robustness: Deploying RL agents in real-world settings necessitates careful consideration of safety and robustness. Unforeseen circumstances or adversarial attacks can lead to catastrophic failures.
- Explainability and Interpretability: Understanding why an RL agent makes a particular decision can be challenging. This lack of transparency hinders trust and adoption in safety-critical applications.
The Future of Reinforcement Learning:
The future of RL hinges on overcoming these limitations. Active research areas include:
- Sample Efficient RL: Developing algorithms that learn effectively with less data.
- Safe RL: Designing methods to ensure the safety and robustness of RL agents.
- Transfer Learning: Enabling RL agents to transfer knowledge learned in one environment to another, reducing the need for extensive retraining.
- Explainable RL: Developing techniques to make the decision-making process of RL agents more transparent and understandable.
Conclusion:
Reinforcement learning holds tremendous potential to revolutionize various aspects of AI. While it has already achieved significant milestones, significant challenges remain. Addressing these challenges through ongoing research and development is crucial for realizing RL's full potential and ensuring its responsible deployment in the real world. The journey toward truly intelligent, adaptable, and reliable AI systems powered by RL is ongoing, but the progress made so far is undeniably impressive.
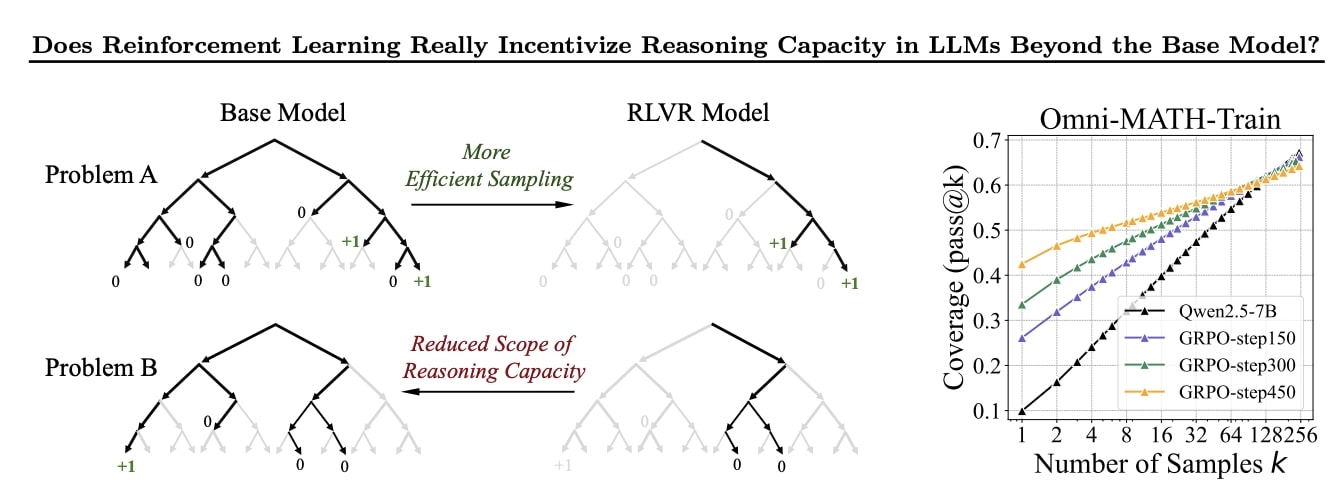
Thank you for visiting our website, your trusted source for the latest updates and in-depth coverage on The Reality Of Reinforcement Learning: Does It Deliver On Its AI Promises?. We're committed to keeping you informed with timely and accurate information to meet your curiosity and needs.
If you have any questions, suggestions, or feedback, we'd love to hear from you. Your insights are valuable to us and help us improve to serve you better. Feel free to reach out through our contact page.
Don't forget to bookmark our website and check back regularly for the latest headlines and trending topics. See you next time, and thank you for being part of our growing community!
Featured Posts
-
Zemos Absence From Thunderbolts The Director Speaks Out
Apr 29, 2025 -
Revealed The Lewis Hamilton Flaw Frustrating Ferrari
Apr 29, 2025 -
Solid State Power Bank Revolution Kuxius Premium Offering And Its Advantages
Apr 29, 2025 -
Boeings 737 Max And Other Aircraft Back In The Air After China Ban
Apr 29, 2025 -
Understanding Juneteenth Seattles Commemoration And Its Meaning
Apr 29, 2025
Latest Posts
-
Transfer News Atletico Madrid Eye Arsenals Myles Lewis Skelly
Apr 30, 2025 -
Trump Complained To Bezos About Critical Amazon Report Sources
Apr 30, 2025 -
Unfavorable Conditions And Tosses Decide Rcb Vs Pietersens Team
Apr 30, 2025 -
Madrid Open Update Rublev Eliminated Sabalenka Wins Following Zverev Photo Warning
Apr 30, 2025 -
Adam Johnson Death Investigation Arrest Leads To No Criminal Charges Filed
Apr 30, 2025