The Reality Of Reinforcement Learning: Does It Fundamentally Improve AI?
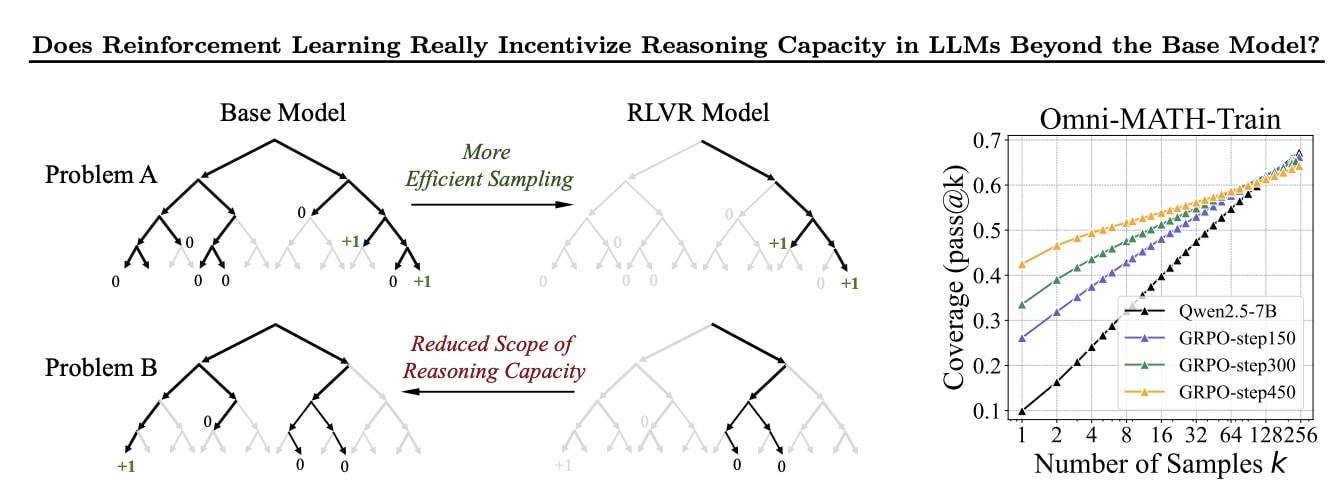
Welcome to your ultimate source for breaking news, trending updates, and in-depth stories from around the world. Whether it's politics, technology, entertainment, sports, or lifestyle, we bring you real-time updates that keep you informed and ahead of the curve.
Our team works tirelessly to ensure you never miss a moment. From the latest developments in global events to the most talked-about topics on social media, our news platform is designed to deliver accurate and timely information, all in one place.
Stay in the know and join thousands of readers who trust us for reliable, up-to-date content. Explore our expertly curated articles and dive deeper into the stories that matter to you. Visit NewsOneSMADCSTDO now and be part of the conversation. Don't miss out on the headlines that shape our world!
Table of Contents
The Reality of Reinforcement Learning: Does it Fundamentally Improve AI?
Reinforcement learning (RL), a subfield of machine learning, has captured the imagination of researchers and the public alike. From mastering complex games like Go and chess to controlling robots with impressive dexterity, RL's achievements are undeniable. But does this transformative technology fundamentally improve AI, or is the hype outpacing the reality? This article delves into the current state of RL, examining its strengths, limitations, and ultimate impact on the future of artificial intelligence.
RL: A Powerful Tool, But Not a Silver Bullet
Reinforcement learning differs from other machine learning approaches by focusing on learning through trial and error. An agent interacts with an environment, receiving rewards or penalties for its actions. Over time, the agent learns an optimal policy – a strategy to maximize its cumulative reward. This iterative process allows RL agents to tackle problems that are difficult or impossible to solve using traditional methods. The success stories are compelling: AlphaGo's victory over a world champion Go player, impressive robotics feats, and advancements in game AI are all testaments to RL's power.
However, the reality is far more nuanced. Several significant challenges limit the widespread adoption and true fundamental improvement of AI through RL:
- Data Efficiency: RL algorithms often require vast amounts of data and many iterations to learn effectively. This can be computationally expensive and time-consuming, particularly in real-world scenarios where data collection is costly or dangerous.
- Reward Function Design: Defining a suitable reward function is crucial for successful RL. A poorly designed reward function can lead the agent to exploit loopholes or achieve unintended goals, a problem often referred to as "reward hacking." This highlights the critical need for careful consideration and expert design.
- Sample Inefficiency: Many RL algorithms suffer from sample inefficiency, meaning they require a large number of interactions with the environment to converge on an optimal solution. This makes them impractical for applications where interactions are expensive or time-sensitive.
- Generalization Challenges: RL agents often struggle to generalize their learned behavior to new, unseen situations. An agent trained to play one version of a game might perform poorly on a slightly modified version, hindering their adaptability and real-world applicability.
- Interpretability and Explainability: Understanding why an RL agent makes a particular decision is often difficult. The complex internal workings of these algorithms can make it challenging to debug errors or build trust in their decisions, particularly in critical applications like healthcare or finance.
The Future of Reinforcement Learning and AI
Despite these challenges, reinforcement learning remains a vital area of research with immense potential. Ongoing research focuses on addressing these limitations:
- Improved algorithms: Researchers are developing more efficient and sample-efficient RL algorithms, reducing the computational cost and data requirements.
- Safe RL: Techniques are being developed to ensure that RL agents behave safely and reliably in real-world environments, minimizing the risk of unintended consequences.
- Transfer learning: Research aims to improve the ability of RL agents to transfer knowledge learned in one environment to another, enhancing generalization capabilities.
- Explainable RL (XRL): Efforts are underway to make RL agents more interpretable and transparent, increasing trust and facilitating debugging.
In conclusion, while reinforcement learning has demonstrably advanced the field of AI, it's not a universal solution. Its impact is significant, but its limitations must be acknowledged. The future of AI hinges on continued research and development addressing the challenges mentioned above. Only then can we truly unlock the transformative potential of RL and its capacity to fundamentally reshape our world. The journey towards truly intelligent, adaptable, and trustworthy AI systems powered by reinforcement learning is ongoing, and the breakthroughs to come are eagerly awaited.
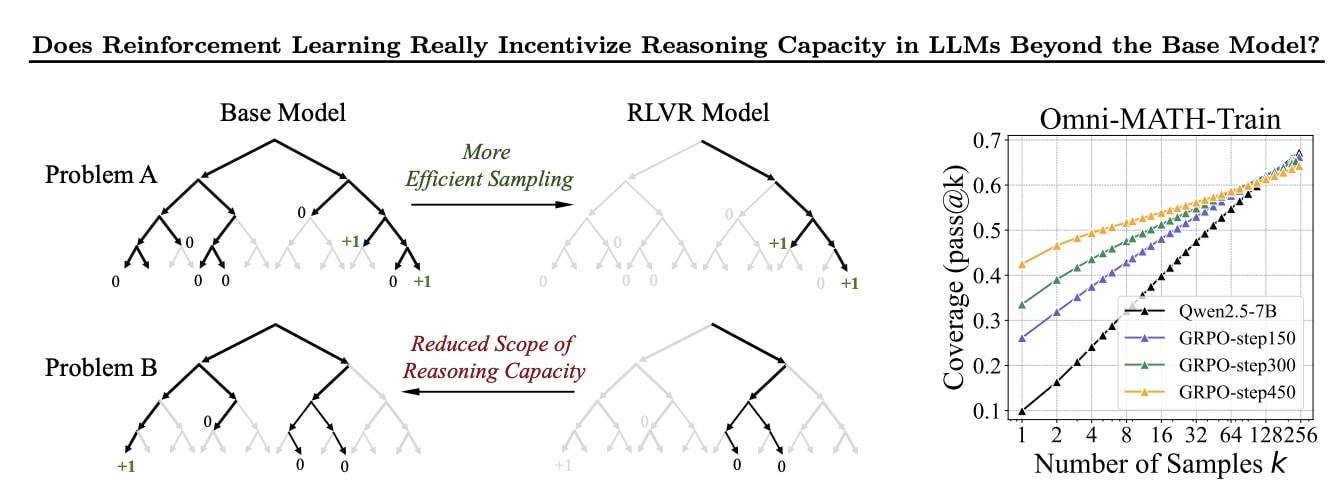
Thank you for visiting our website, your trusted source for the latest updates and in-depth coverage on The Reality Of Reinforcement Learning: Does It Fundamentally Improve AI?. We're committed to keeping you informed with timely and accurate information to meet your curiosity and needs.
If you have any questions, suggestions, or feedback, we'd love to hear from you. Your insights are valuable to us and help us improve to serve you better. Feel free to reach out through our contact page.
Don't forget to bookmark our website and check back regularly for the latest headlines and trending topics. See you next time, and thank you for being part of our growing community!
Featured Posts
-
Kolkata Triumphs Over Delhi In Ipl Narines Crucial Role In The Knight Riders Victory
Apr 30, 2025 -
499 Overseas Flights Qantas Unveils Massive Sale
Apr 30, 2025 -
Ligue Des Champions Premier Test Pour Le Psg A Londres Face A Arsenal
Apr 30, 2025 -
Sabalenka Extends Winning Streak To Nine Against Mertens
Apr 30, 2025 -
Medvedev Rejects Another Tennis Documentary A Definite No
Apr 30, 2025
Latest Posts
-
Update Case Closed No Charges In Death Of Former Nhl Player Adam Johnson
Apr 30, 2025 -
Follow Dc Vs Kkr Live Cricket Score Ball By Ball Updates
Apr 30, 2025 -
The Smashing Machine Trailer Dwayne Johnson As Ufc Legend Mark Kerr
Apr 30, 2025 -
Diana Shnaider Vs Iga Swiatek Madrid Open Odds And Predictions
Apr 30, 2025 -
11 Jump In Residential Bills Dte Rate Hike Request Sparks Outrage
Apr 30, 2025