The Reality Of Reinforcement Learning: Its True Role In Modern AI
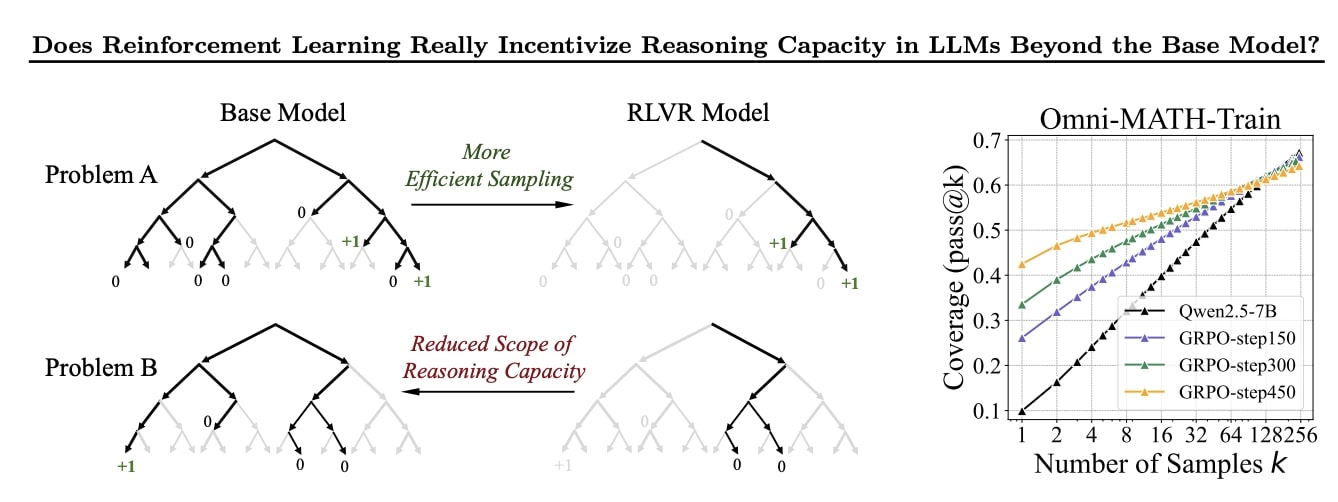
Welcome to your ultimate source for breaking news, trending updates, and in-depth stories from around the world. Whether it's politics, technology, entertainment, sports, or lifestyle, we bring you real-time updates that keep you informed and ahead of the curve.
Our team works tirelessly to ensure you never miss a moment. From the latest developments in global events to the most talked-about topics on social media, our news platform is designed to deliver accurate and timely information, all in one place.
Stay in the know and join thousands of readers who trust us for reliable, up-to-date content. Explore our expertly curated articles and dive deeper into the stories that matter to you. Visit NewsOneSMADCSTDO now and be part of the conversation. Don't miss out on the headlines that shape our world!
Table of Contents
The Reality of Reinforcement Learning: Its True Role in Modern AI
Reinforcement learning (RL), a captivating field within artificial intelligence, often evokes images of superhuman AI agents mastering complex games like Go and Dota 2. While these achievements are undeniably impressive, the reality of RL's role in modern AI is far more nuanced and multifaceted than popular narratives suggest. This article delves into the true capabilities and limitations of RL, exploring its current applications and future potential.
Beyond the Game: Real-World Applications of Reinforcement Learning
While breakthroughs in game playing have propelled RL into the spotlight, its true potential lies in tackling real-world problems. Several sectors are already witnessing significant advancements thanks to RL:
-
Robotics: RL algorithms are instrumental in training robots to perform complex tasks, from navigating dynamic environments to manipulating objects with dexterity. This includes applications in manufacturing, logistics, and even surgical assistance. Imagine robots autonomously assembling intricate components or assisting surgeons with pinpoint accuracy – RL is making this a reality.
-
Personalized Medicine: RL is showing promise in optimizing treatment plans for individual patients. By learning from patient data and treatment outcomes, RL algorithms can adapt treatment strategies in real-time, maximizing efficacy and minimizing side effects. This personalized approach holds immense potential for improving healthcare outcomes.
-
Resource Management: Optimizing resource allocation is crucial in various fields, from energy grids to traffic flow. RL algorithms can analyze complex systems and learn optimal strategies for managing resources efficiently, reducing waste and improving overall performance. This translates to cost savings and improved sustainability.
-
Finance: Algorithmic trading strategies are increasingly leveraging RL to identify profitable opportunities and manage risk. RL's ability to learn from market data and adapt to changing conditions makes it a powerful tool in the finance industry.
The Challenges and Limitations of Reinforcement Learning
Despite its impressive potential, RL faces significant challenges:
-
Data Requirements: RL algorithms require vast amounts of data for effective training, often necessitating extensive simulations or real-world interactions. This can be time-consuming and expensive.
-
Sample Inefficiency: RL can be notoriously sample-inefficient, meaning it requires a large number of training trials to converge on an optimal solution. This can limit its applicability in situations where data collection is costly or dangerous.
-
Reward Function Design: Defining a suitable reward function is crucial for guiding the RL agent towards desirable behavior. Poorly designed reward functions can lead to unintended consequences and suboptimal performance. This is a significant hurdle in many real-world applications.
-
Interpretability and Explainability: Understanding why an RL agent makes a specific decision can be difficult. Lack of transparency can hinder trust and adoption, particularly in high-stakes applications like healthcare and finance.
The Future of Reinforcement Learning in AI
Despite these challenges, the future of RL looks bright. Ongoing research is focused on addressing these limitations through:
-
Improved algorithms: Researchers are developing more sample-efficient and robust RL algorithms.
-
Transfer learning: Leveraging knowledge learned in one environment to accelerate learning in another can significantly reduce data requirements.
-
Safe RL: Methods for ensuring safe and reliable behavior in RL agents are crucial for widespread adoption in real-world applications.
-
Explainable RL (XRL): Developing techniques to make RL agents more transparent and interpretable is essential for building trust and understanding.
Conclusion:
Reinforcement learning is not a magic bullet for all AI problems, but its potential to solve complex real-world challenges is undeniable. While the captivating game-playing achievements are noteworthy, the true impact of RL lies in its ability to optimize complex systems, personalize solutions, and improve decision-making across various industries. As researchers continue to overcome the current limitations, RL is poised to play an increasingly significant role in shaping the future of AI.
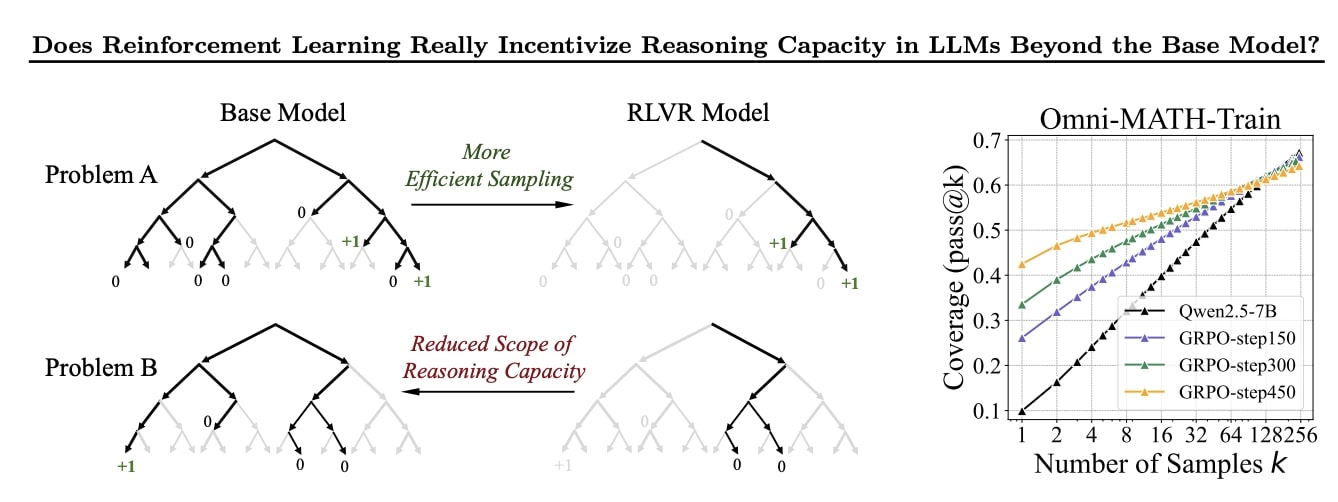
Thank you for visiting our website, your trusted source for the latest updates and in-depth coverage on The Reality Of Reinforcement Learning: Its True Role In Modern AI. We're committed to keeping you informed with timely and accurate information to meet your curiosity and needs.
If you have any questions, suggestions, or feedback, we'd love to hear from you. Your insights are valuable to us and help us improve to serve you better. Feel free to reach out through our contact page.
Don't forget to bookmark our website and check back regularly for the latest headlines and trending topics. See you next time, and thank you for being part of our growing community!
Featured Posts
-
Meta Xr Glasses Release Date Latest Speculation Points To A 2023 Launch
Apr 30, 2025 -
Analysis Of Dual Propulsion Experiments Conducted On Otp 2
Apr 30, 2025 -
Mescal Wright Barbaro And Coughlan Exciting New Productions At The National Theatre
Apr 30, 2025 -
Urgent Evacuations Wildfire Races Southeast Of Tucson Due To Strong Winds
Apr 30, 2025 -
Woo Commerce Users Under Attack Phishing Campaign Exploits Fake Update To Install Malware
Apr 30, 2025
Latest Posts
-
How To Watch Manchester United Vs Chelsea Womens Super League Clash
Apr 30, 2025 -
Ryzen 3950 X Powered Workstation Targets Ai Professionals Challenges Nvidia Dgx Spark
Apr 30, 2025 -
Inzaghi Sceglie La Formazione Le Ultime Novita Dall Inter Prima Del Barcellona
Apr 30, 2025 -
Spains Energy Minister Rejects Renewable Energy Blame For Recent Blackout
Apr 30, 2025 -
Jordan Travis Announces Retirement From The Jets
Apr 30, 2025