The Unfulfilled Promise Of Reinforcement Learning In AI Development
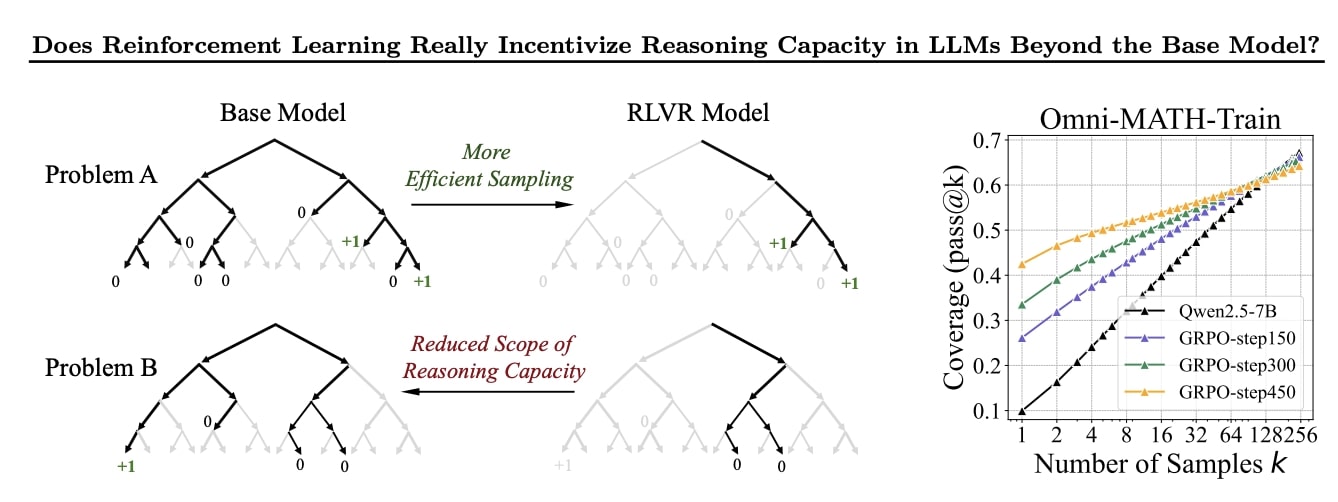
Welcome to your ultimate source for breaking news, trending updates, and in-depth stories from around the world. Whether it's politics, technology, entertainment, sports, or lifestyle, we bring you real-time updates that keep you informed and ahead of the curve.
Our team works tirelessly to ensure you never miss a moment. From the latest developments in global events to the most talked-about topics on social media, our news platform is designed to deliver accurate and timely information, all in one place.
Stay in the know and join thousands of readers who trust us for reliable, up-to-date content. Explore our expertly curated articles and dive deeper into the stories that matter to you. Visit NewsOneSMADCSTDO now and be part of the conversation. Don't miss out on the headlines that shape our world!
Table of Contents
The Unfulfilled Promise of Reinforcement Learning in AI Development: Hype vs. Reality
Reinforcement learning (RL), a subfield of machine learning, has captivated the AI community for years with its promise of creating truly intelligent agents. The idea of algorithms learning through trial and error, mimicking human learning, is incredibly appealing. However, despite significant advancements and considerable hype, RL's widespread application remains limited. This article explores the gap between the promised potential of RL and its current limitations in real-world AI development.
The Allure of Reinforcement Learning:
Reinforcement learning's appeal stems from its ability to tackle complex problems where explicit programming is difficult or impossible. Instead of relying on pre-labeled data, RL agents learn by interacting with an environment, receiving rewards for desirable actions and penalties for undesirable ones. This approach holds immense potential in various domains, including:
- Robotics: Training robots to perform intricate tasks like assembling products or navigating complex terrains.
- Game playing: RL's success in mastering games like Go and chess is well-documented.
- Personalized recommendations: Developing algorithms that adapt to individual user preferences in real-time.
- Resource optimization: Improving efficiency in areas like energy grids and traffic management.
The Challenges Hindering Widespread Adoption:
While the potential is undeniable, significant hurdles impede RL's widespread adoption:
1. Data Efficiency: RL algorithms often require massive amounts of data and interactions to learn effectively. This can be computationally expensive and time-consuming, especially in real-world scenarios where experimentation is costly or dangerous. This data hunger significantly limits its applicability in many fields.
2. Sample Inefficiency: RL agents can take a long time to converge on optimal solutions, exploring many ineffective strategies before finding the right path. This sample inefficiency is a major drawback, particularly when dealing with complex environments.
3. Reward Function Design: Defining a clear and effective reward function is crucial for successful RL. However, designing such functions can be incredibly challenging, particularly for complex tasks. A poorly designed reward function can lead the agent to learn undesirable or unintended behaviors, a problem often referred to as reward hacking.
4. Generalization: RL agents often struggle to generalize their learned behaviors to new, unseen situations. This lack of generalization limits their adaptability and robustness in real-world applications where conditions are constantly changing.
5. Interpretability and Explainability: Understanding why an RL agent makes a particular decision is often difficult. This lack of transparency raises concerns in safety-critical applications where understanding the decision-making process is paramount.
The Future of Reinforcement Learning:
Despite these challenges, research in RL continues to progress rapidly. New techniques are being developed to address issues like sample efficiency and reward function design. Areas like transfer learning and meta-learning hold promise in improving generalization capabilities. Furthermore, advancements in explainable AI (XAI) are aimed at making RL agents more transparent and understandable.
Conclusion:
The hype surrounding reinforcement learning is undeniable. While its potential to revolutionize AI is vast, several significant challenges must be overcome before it can deliver on its full promise. Ongoing research and innovation are crucial to address these limitations and unlock the true potential of reinforcement learning in real-world applications. The journey towards truly intelligent, adaptable AI agents powered by RL is still ongoing, and significant breakthroughs are still needed.
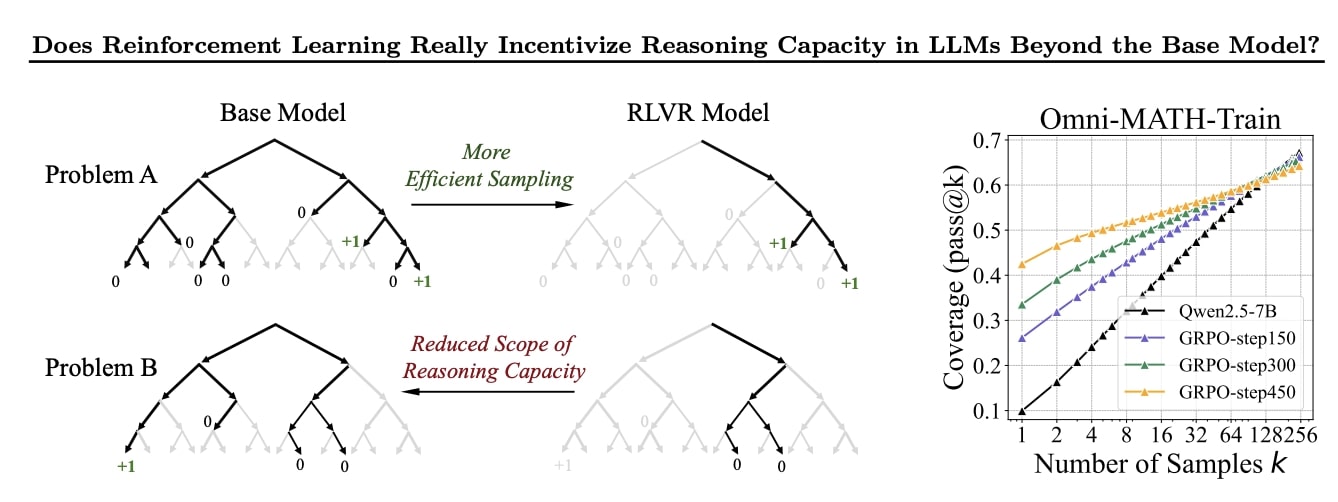
Thank you for visiting our website, your trusted source for the latest updates and in-depth coverage on The Unfulfilled Promise Of Reinforcement Learning In AI Development. We're committed to keeping you informed with timely and accurate information to meet your curiosity and needs.
If you have any questions, suggestions, or feedback, we'd love to hear from you. Your insights are valuable to us and help us improve to serve you better. Feel free to reach out through our contact page.
Don't forget to bookmark our website and check back regularly for the latest headlines and trending topics. See you next time, and thank you for being part of our growing community!
Featured Posts
-
2010 Abbott Plea Revealed Greens Confirm Rejection Of Post Election Horse Trading
Apr 29, 2025 -
Ipl History Exploring The Contributions Of Afghan Cricketers Including Karim Janat
Apr 29, 2025 -
Lais Political Stance Unveiled Amidst Hong Kong Protests China Daily
Apr 29, 2025 -
William Lai Under Fire Tens Of Thousands Rally Against Taiwanese Vp
Apr 29, 2025 -
Ryanairs Regrettable Announcement Impact On Uk Passengers
Apr 29, 2025
Latest Posts
-
The Last Of Us And Beyond Unveiling The Talent Of Isabela Merced
Apr 29, 2025 -
Afghanistans 10th Ipl Player Karim Janats Debut For Gujarat Titans
Apr 29, 2025 -
Uncertainty Looms Over Philadelphia Ameri Corps Funding After Doges Sweeping Cuts
Apr 29, 2025 -
Circonscriptions A Risque Anticiper Les Resultats Electoraux
Apr 29, 2025 -
Serie A Matchday 34 Liveblog Udinese Bologna Lazio Parma Verona Cagliari Updates
Apr 29, 2025