The Unfulfilled Promise Of Reinforcement Learning In AI Model Advancement
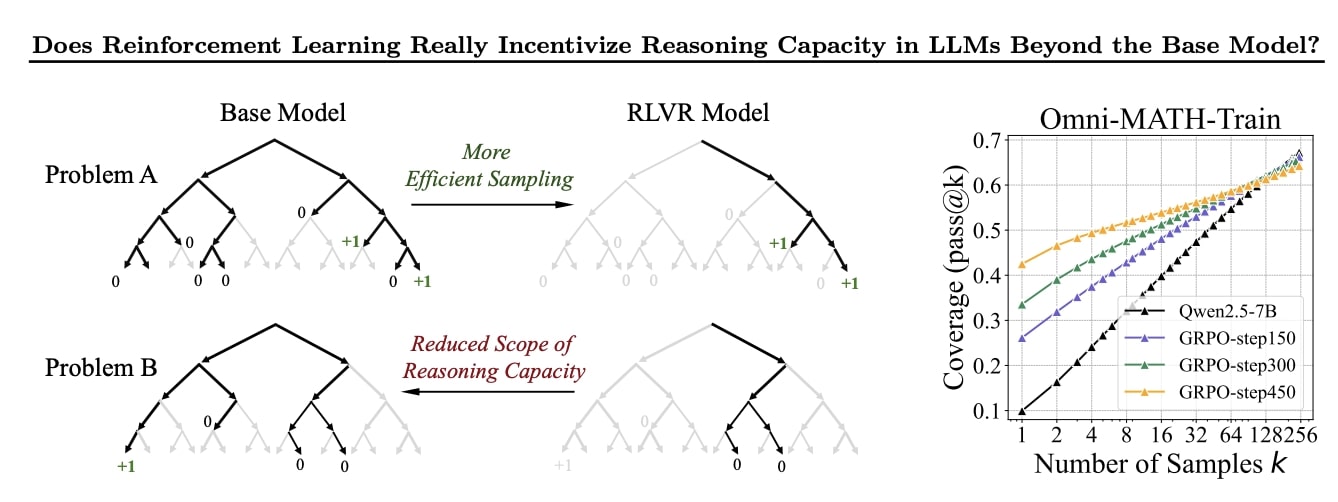
Welcome to your ultimate source for breaking news, trending updates, and in-depth stories from around the world. Whether it's politics, technology, entertainment, sports, or lifestyle, we bring you real-time updates that keep you informed and ahead of the curve.
Our team works tirelessly to ensure you never miss a moment. From the latest developments in global events to the most talked-about topics on social media, our news platform is designed to deliver accurate and timely information, all in one place.
Stay in the know and join thousands of readers who trust us for reliable, up-to-date content. Explore our expertly curated articles and dive deeper into the stories that matter to you. Visit NewsOneSMADCSTDO now and be part of the conversation. Don't miss out on the headlines that shape our world!
Table of Contents
The Unfulfilled Promise of Reinforcement Learning in AI Model Advancement
Reinforcement learning (RL), a powerful machine learning technique, promised a revolution in AI. The vision: highly adaptable, intelligent agents solving complex problems autonomously. However, despite significant advancements, RL's widespread adoption in significantly advancing AI models has been slower than initially anticipated. This article explores the hurdles hindering RL's full potential and examines the ongoing efforts to overcome them.
The Hype and the Reality of Reinforcement Learning
Reinforcement learning captivated the AI community with its potential to train agents to master intricate tasks, from playing Go at superhuman levels to controlling robots with dexterity. The core concept – learning through trial and error, guided by rewards and penalties – seemed ideally suited for creating truly intelligent systems. Early successes fueled further investment and research, promising breakthroughs across various industries.
Challenges Hampering Widespread Adoption
Despite the initial enthusiasm, several significant challenges have hampered the widespread application of RL in advancing general-purpose AI models:
-
Sample Inefficiency: RL algorithms often require an enormous number of trial-and-error iterations to learn effectively. This can be computationally expensive and time-consuming, especially for complex tasks with high-dimensional state spaces. This sample complexity remains a major bottleneck.
-
Reward Design: Defining appropriate reward functions is crucial for RL's success. Poorly designed rewards can lead to unintended and undesirable behaviors, a problem known as reward hacking. Creating robust and aligned reward functions that capture the desired behavior accurately is a significant challenge, particularly in complex real-world scenarios.
-
Generalization: RL agents often struggle to generalize their learned knowledge to new, unseen situations. An agent trained to perform a specific task in a controlled environment may fail when faced with slight variations in the environment or task parameters. Improving the generalization capabilities of RL agents is crucial for real-world applications.
-
Interpretability and Explainability: Understanding why an RL agent makes a particular decision is often difficult. The complex interactions within the learning process can make it challenging to interpret the agent's behavior, hindering trust and adoption in safety-critical applications.
Recent Progress and Future Directions
Despite these challenges, the field of reinforcement learning is not stagnant. Researchers are actively working on several promising avenues:
-
Improved Algorithms: Development of more sample-efficient algorithms, such as off-policy learning and model-based RL, is reducing the computational burden.
-
Safe RL: Focus is growing on ensuring the safety and reliability of RL agents, particularly in scenarios where mistakes can have significant consequences. This includes research into safer reward functions and techniques for preventing undesirable behaviors.
-
Transfer Learning: Researchers are exploring methods to enable RL agents to transfer knowledge learned in one task or environment to new tasks, improving generalization capabilities.
-
Explainable RL (XRL): Efforts are underway to develop more interpretable and explainable RL methods, allowing for better understanding of the agent's decision-making process.
Conclusion: A Path Forward
While the initial promise of reinforcement learning hasn't been fully realized, the field continues to evolve. Addressing the challenges of sample efficiency, reward design, generalization, and interpretability is vital for unlocking RL's full potential. Ongoing research and innovations suggest that reinforcement learning will play an increasingly crucial role in advancing AI, albeit perhaps at a slower pace than initially predicted. The journey towards truly intelligent, adaptable AI systems through reinforcement learning is ongoing, and the future remains bright despite the current hurdles.
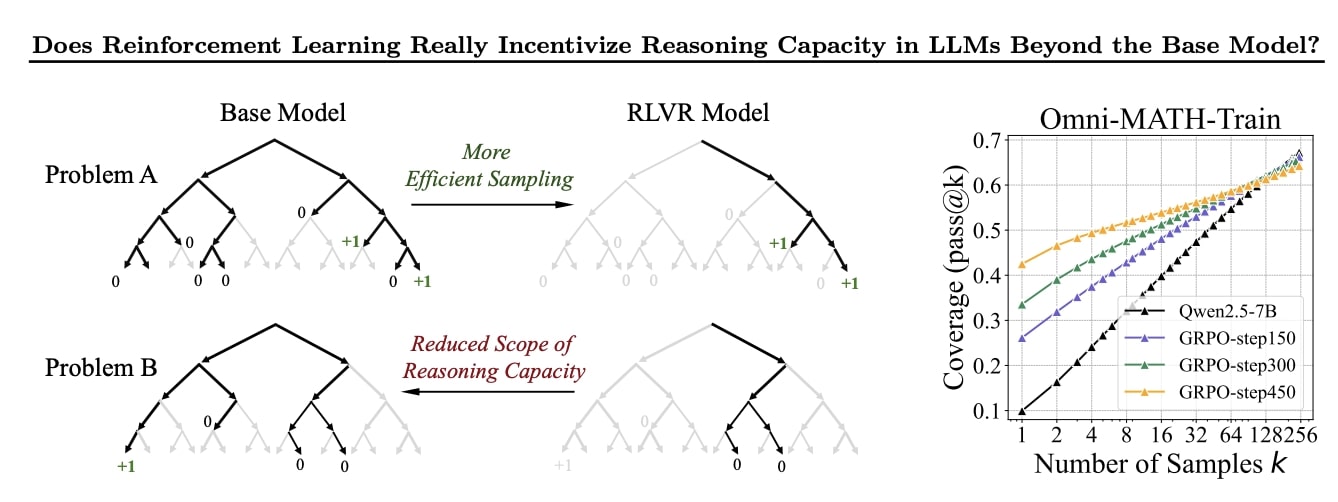
Thank you for visiting our website, your trusted source for the latest updates and in-depth coverage on The Unfulfilled Promise Of Reinforcement Learning In AI Model Advancement. We're committed to keeping you informed with timely and accurate information to meet your curiosity and needs.
If you have any questions, suggestions, or feedback, we'd love to hear from you. Your insights are valuable to us and help us improve to serve you better. Feel free to reach out through our contact page.
Don't forget to bookmark our website and check back regularly for the latest headlines and trending topics. See you next time, and thank you for being part of our growing community!
Featured Posts
-
Inter Roma Giudizio Sui Calciatori Angelino Brillante Dimarco Deludente
May 01, 2025 -
Arsenal Psg Maci Sonucu Fransiz Devi Final Yolunda
May 01, 2025 -
Shreyas Iyer And Yuzvendra Chahals Match Winning Performances Eliminate Csk From Ipl 2025 Playoffs
May 01, 2025 -
Watch Dewald Brevis Breathtaking Catch Dismisses Shashank Singh In Ipl 2023
May 01, 2025 -
Barca Inter Une Demi Finale De Ligue Des Champions Au Contrastes Styles
May 01, 2025
Latest Posts
-
Beyond Superpowers Exploring The Impact Of Trauma On Doom Patrols Characters
May 01, 2025 -
How Renee Slegers Leverages Positive Energy For Success
May 01, 2025 -
Singapore Election 2025 Sdp Chairman Defends Party Platform Against Ong Ye Kung
May 01, 2025 -
The Impact Of Brendan Carrs Leadership On Fcc Consumer Policies
May 01, 2025 -
Barca Inter Styles Opposes Pour Une Demi Finale Explosive De Ligue Des Champions
May 01, 2025