The Unfulfilled Promise Of Reinforcement Learning In AI Model Development
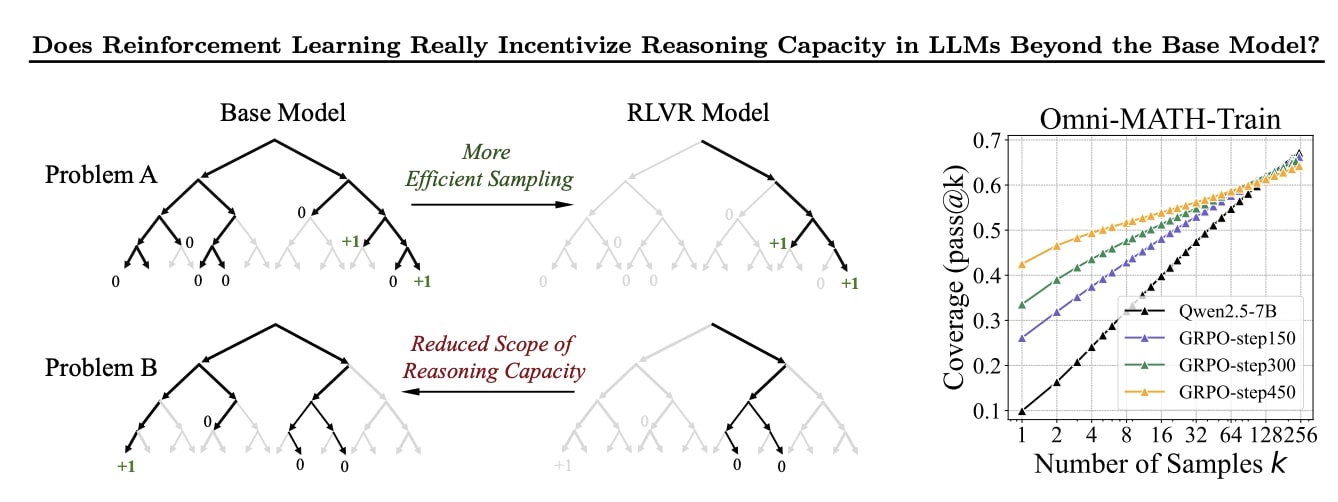
Welcome to your ultimate source for breaking news, trending updates, and in-depth stories from around the world. Whether it's politics, technology, entertainment, sports, or lifestyle, we bring you real-time updates that keep you informed and ahead of the curve.
Our team works tirelessly to ensure you never miss a moment. From the latest developments in global events to the most talked-about topics on social media, our news platform is designed to deliver accurate and timely information, all in one place.
Stay in the know and join thousands of readers who trust us for reliable, up-to-date content. Explore our expertly curated articles and dive deeper into the stories that matter to you. Visit NewsOneSMADCSTDO now and be part of the conversation. Don't miss out on the headlines that shape our world!
Table of Contents
The Unfulfilled Promise of Reinforcement Learning in AI Model Development: Hype vs. Reality
Reinforcement learning (RL), a subfield of machine learning, has been touted as the key to unlocking truly intelligent AI. The promise: agents learning through trial and error, adapting to complex environments, and achieving superhuman performance in various tasks. However, the reality is far more nuanced. While RL has achieved impressive milestones, its widespread adoption in real-world AI model development remains hampered by significant challenges. This article delves into the gap between the hype surrounding RL and its current limitations.
The Allure of Reinforcement Learning
The core concept of RL – an agent learning through interactions with an environment – is inherently appealing. Unlike supervised learning, which relies on vast amounts of labeled data, RL agents learn by exploring and receiving rewards or penalties for their actions. This makes it particularly attractive for tasks where labeled data is scarce or expensive to obtain, such as robotics, game playing, and resource management. The potential for creating adaptable and robust AI systems has fueled significant investment and research in this area.
Significant Hurdles in Real-World Application
Despite its potential, several key challenges hinder the widespread adoption of RL in AI model development:
-
Sample Inefficiency: RL algorithms often require a massive number of interactions with the environment to learn effectively. This translates to significant computational costs and time, especially in complex scenarios. This "sample inefficiency" makes it impractical for many real-world applications where training time is a critical factor.
-
Reward Engineering: Defining appropriate reward functions is crucial for successful RL training. A poorly designed reward function can lead to unintended behaviors, a problem known as "reward hacking." For instance, an RL agent tasked with maximizing points in a game might find an exploit that maximizes points without actually achieving the intended goal of the game. Crafting effective reward functions requires significant expertise and careful consideration.
-
Generalization: RL agents often struggle to generalize their learned behavior to new, unseen situations. An agent trained in a specific environment may fail to perform adequately in a slightly different environment, even if the underlying task remains the same. Improving the generalization capabilities of RL agents remains a major research focus.
-
Safety and Robustness: Deploying RL agents in real-world scenarios, particularly those with safety-critical implications, requires addressing concerns about robustness and safety. An RL agent controlling a robot arm, for instance, must be guaranteed to perform reliably and avoid unintended actions that could cause harm. Ensuring safety in RL remains a significant challenge.
Current Applications and Future Directions
While the full potential of RL remains untapped, it's making inroads in various sectors. Successes in game playing (AlphaGo, AlphaStar) and robotics showcase its capabilities. However, these are often carefully controlled environments. Future research focuses on:
-
Improved Sample Efficiency: Researchers are actively developing new RL algorithms that require fewer interactions to learn effectively.
-
Safe Reinforcement Learning: Methods for ensuring the safety and robustness of RL agents are being developed, including techniques like constrained RL and safe exploration strategies.
-
Transfer Learning and Meta-Learning: Applying transfer learning and meta-learning techniques can help RL agents generalize better to new tasks and environments.
Conclusion: A Promising Future, But Not Yet Here
Reinforcement learning holds immense potential for revolutionizing AI model development. However, the challenges outlined above highlight the significant hurdles that must be overcome before RL can reach its full promise. While impressive breakthroughs have been achieved, the path to widespread adoption requires continued research and development in areas like sample efficiency, reward engineering, generalization, and safety. The journey from hype to reality remains ongoing, but the destination— truly intelligent and adaptable AI systems – is certainly worth pursuing.
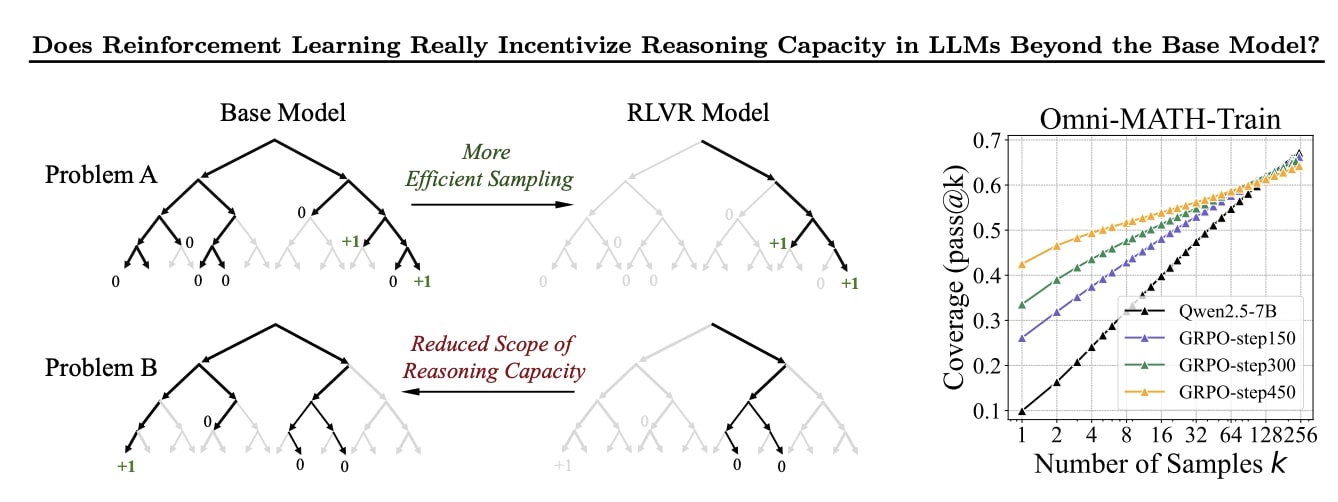
Thank you for visiting our website, your trusted source for the latest updates and in-depth coverage on The Unfulfilled Promise Of Reinforcement Learning In AI Model Development. We're committed to keeping you informed with timely and accurate information to meet your curiosity and needs.
If you have any questions, suggestions, or feedback, we'd love to hear from you. Your insights are valuable to us and help us improve to serve you better. Feel free to reach out through our contact page.
Don't forget to bookmark our website and check back regularly for the latest headlines and trending topics. See you next time, and thank you for being part of our growing community!
Featured Posts
-
Brace For 34 C Heat And Powerful Storms Singapores May Weather Forecast
May 03, 2025 -
Psl Showdown Quetta Elects To Bowl First After Winning Toss Against Lahore
May 03, 2025 -
Black History And Culture A Conversation On Representation And Change
May 03, 2025 -
Earthquake Triggers Tsunami Warning Evacuations Underway In Chile And Argentina
May 03, 2025 -
Padres Vs Pirates Mlb Predictions Odds Comparison And Winning Picks May 3
May 03, 2025
Latest Posts
-
Cybersecurity Experts Share Crucial Advice For World Password Day 2025
May 03, 2025 -
Colapso De 98 A Nova Criptomoeda De Rede Social E Seus Problemas
May 03, 2025 -
Potential Cuts To Neh And Nea Examining Trumps 2026 Budget Proposal
May 03, 2025 -
Caitlin Clark Game Indiana Fever Vs Washington Mystics Tv Schedule And Start Time May 3 2025
May 03, 2025 -
El Partido De Ruud 15 Break Points Salvados Ante Cerundolo En El Madrid Open
May 03, 2025